Academia.edu no longer supports Internet Explorer.
To browse Academia.edu and the wider internet faster and more securely, please take a few seconds to upgrade your browser .
Enter the email address you signed up with and we'll email you a reset link.
- We're Hiring!
- Help Center
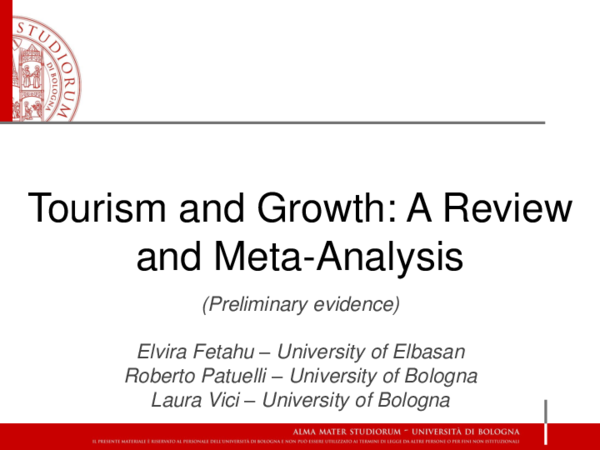

Tourism and Growth: A Review and Meta-Analysis

In recent decades, several waves of studies on tourism have considered its potential role in generating economic development. This objective is often operationalized by attempting to measure the effect of a number of tourism-related variables on GDP or other economic outcomes. In other words, a so-called “tourism-led growth hypothesis” (TLGH) is tested. Most studies in this line of research focus on single countries or even destinations, building on rather specific case studies or on a limited number of observations. On the other hand, other studies present evidence for groups of countries, aiming at generating generalizable findings. Several different methods are employed for this purpose, going from standard time series and Granger causality approaches to advanced dynamic panel data models. Only few papers have till now tried to reconcile the results from such different approaches and case studies, either qualitatively in the form of reviews, or quantitatively by means of meta-analysis (the only available contribution in this case being the one by Castro-Nuño et al., 2013, Journal of Travel Research). This paper aims to provide an overall assessment of a large sample of almost 100 studies on the TLGH published over the last thirty years and their respective findings. We do so by first providing a detailed literature review, and by subsequently carrying out a set of meta-analytical regressions. In particular, we focus on the different dimensions by which studies vary, such as the year of publication, the type of database used, country of application, period considered, econometric method employed and more. In addition, we control for the different possible variables used as indicators of economic outcome as well as to measure tourism demand (e.g., in this case, tourism receipts, tourism expenditure, tourist arrivals).
Related Papers
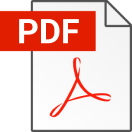
Nicholas M. Odhiambo
Th e development of tourism has become one of the central issues taken up by many countries – in light of the potential benefi ts it has for the economy. Moreover, the causal link between tourism and economic growth has long been a subject of interest in many studies, with no unanimous agreement on the direction of causality between the two variables. Th e main arguments that have been put forward on the direction of causality are: Firstly, that tourism causes economic growth (tourism-led growth hypothesis); and secondly, that it is economic growth that leads to the growth of the tourism sector (growth-led tourism hypothesis). In this paper, we review some of the previous empirical studies that have been conducted, in order to examine the causality between tourism-sector development and economic growth in both developed and developing countries. Th ese studies have used time series data analysis, panel/cross sectional data analysis as well as input/output analysis. Our empirical lit...
Mina Dragouni
The current literature on the tourism-economic growth causal relationship has not yet reached to a clear empirical consensus. The aim of this paper is to revisit this ambiguous relationship by examining the dynamics between tourism and economic growth from a more holistic view. In particular, we focus on 113 countries over the period 1995-2011, which we group into clusters based on six different criteria. A Panel Vector Autoregressive model is employed to reveal the tourism-economy interdependencies across these clusters. Overall, our findings cannot support the tourism-led economic growth hypothesis in any of our clusters. Rather, the economic-driven tourism growth hypothesis seems to prevail is most cases, although some short-lived bidirectional causalities are also identified. Thus, depending on the level of tourism competitiveness and economic development different policy implications apply.
Journal of Travel Research
Robin Nunkoo, Ph.D , Zameelah Khan Jaffur , Warren Moraghen
Numerous studies have focused on delineating the relationship between tourism and economic growth. In this article, we present the results of a rigorous meta-regression analysis based on 545 estimates drawn from 113 studies that empirically tested the tourism-led growth hypothesis (TLGH). The results suggest the presence of publication bias in the literature on this topic, where the majority of studies report positive and statistically significant estimates. Findings provide support for the TLGH, but they also suggest that the estimates are sensitive to a number of factors that are related to country, data, specification, and estimation characteristics, and time span. Such sensitivities suggest that greater emphasis should be placed on reporting estimates of the relationship between tourism and economic growth across a variety of methodological characteristics and specification and estimation choices. The implications of the results for theory development are also discussed.
This article revisits the ambiguous relationship between tourism and economic growth, providing a comprehensive study of destinations across the globe which takes into account the key dynamics that influence tourism and economic performance. We focus on 113 countries over the period 1995 to 2014, clustered, for the first time, around six criteria that reflect their economic, political, and tourism dimensions. A panel vector autoregressive model is employed, which, in contrast to previous studies, allows the data to reveal any tourism-economy interdependencies across these clusters, without imposing a priori the direction of causality. Overall, the economic-driven tourism growth hypothesis seems to prevail in countries which are developing, nondemocratic, highly bureaucratic and have low tourism specialization. Conversely, bidirectional relationships are established for economies that are stronger, democratic and with higher levels of government effectiveness. Thus, depending on the ...
Elma Satrovic , CANSU UNVER ERBAS
Over the past several decades, the relationship between tourism and economic growth, for both developing and developed countries, has been a popular issue of debate. Taking into account the fact that the tourism is an important sector in the world economy, the knowledge of the sign and size of the impact of tourism earnings on economic growth is of particular importance to policy makers. This paper aims to explore the impact of tourism earnings on economic growth. Panel data of 113 countries are used for the years that span from 1995 to 2015. The potential contribution of tourism to economic growth is analyzed within the conventional augmented Solow growth model. GMM method is employed to account for a dynamic phenomenon of economic growth. The obtained results indicate a significant positive impact of tourism on economic growth. Furthermore, the obtained results indicate that tourism-economic growth nexus differs among income disparity. The obtained findings imply that low-income countries should enhance their economic growth by strengthening their tourism industry.
The aim of this paper is to analyse the relationship between tourism activity and economic growth for Next-11 (N-11) countries. It is concluded that there is a long run relationship between tourist arrivals and gross domestic product (GDP) and tourism arrivals has positive effect on GDP growth in N-11 countries. We find that a unidirectional causality from economic growth to tourism is valid confirming economic driven tourism growth hypothesis.
Current Issues in Tourism
Manuela Pulina
Applied Economics
Anas Mehmud
Tourism Economics
Stanislav Ivanov
RELATED PAPERS
Jimmy Rachmat
Raden Mas Cozar
Txt: Leituras Transdisciplinares de Telas e Textos
Carla Viana Coscarelli
SANGUK CHEON
Journal of University of Human Development
pshtiwan qader
Revista Brasileira de Geografia Física
Leovigildo Santos
IEEE Transactions on Medical Robotics and Bionics
Andria Farrens
EMC - Ginecología-Obstetricia
abdullahi zakariyya
Rahul Sharma
Graciela Cuesta
Schizophrenia Bulletin
Sonia Canals
Plant physiology and biochemistry : PPB
Patricio Arce-johnson
Dados-revista De Ciencias Sociais
Christian E C Lynch
Liceddy Castañeda
Enfermería Clínica
Susana Navalpotro Pascual
Human Mutation
Santiago Rodriguez
European respiratory review : an official journal of the European Respiratory Society
Harm Bogaard
Quantitative Economics and Management Studies
louis Nkwatoh
IAIABC Journal
Jennifer Christian
C. Imbriani
African Journal of Microbiology Research
BMC Neuroscience
RELATED TOPICS
- We're Hiring!
- Help Center
- Find new research papers in:
- Health Sciences
- Earth Sciences
- Cognitive Science
- Mathematics
- Computer Science
- Academia ©2024
Tourism and Economic Growth: A Meta-regression Analysis
- Department of Marketing
- University of Mauritius
- University of Johannesburg
- Griffith University Queensland
Research output : Contribution to journal › Journal article › Research › peer-review
Bibliographical note
- Economic growth
- Meta-regression analysis
- Methodology
- Publication bias
- Tourism-led growth hypothesis
This output contributes to the following UN Sustainable Development Goals (SDGs)
Access to Document
- 10.1177/0047287519844833 Licence: Unspecified
- Persistent link
T1 - Tourism and Economic Growth
T2 - A Meta-regression Analysis
AU - Nunkoo, Robin
AU - Seetanah, Boopen
AU - Jaffur, Zameelah Rifkha Khan
AU - Moraghen, Paul George Warren
AU - Sannassee, Raja Vinesh
N1 - Published online: 29. May 2019
PY - 2020/3
Y1 - 2020/3
N2 - Numerous studies have focused on delineating the relationship between tourism and economic growth. In this article, we present the results of a rigorous meta-regression analysis based on 545 estimates drawn from 113 studies that empirically tested the tourism-led growth hypothesis (TLGH). The results suggest the presence of publication bias in the literature on this topic, where the majority of studies report positive and statistically significant estimates. Findings provide support for the TLGH, but they also suggest that the estimates are sensitive to a number of factors that are related to country data, specification, and estimation characteristics, and time span. Such sensitivities suggest that greater emphasis should be placed on reporting estimates of the relationship between tourism and economic growth across a variety of methodological characteristics and specification and estimation choices. The implications of the results for theory development are also discussed.
AB - Numerous studies have focused on delineating the relationship between tourism and economic growth. In this article, we present the results of a rigorous meta-regression analysis based on 545 estimates drawn from 113 studies that empirically tested the tourism-led growth hypothesis (TLGH). The results suggest the presence of publication bias in the literature on this topic, where the majority of studies report positive and statistically significant estimates. Findings provide support for the TLGH, but they also suggest that the estimates are sensitive to a number of factors that are related to country data, specification, and estimation characteristics, and time span. Such sensitivities suggest that greater emphasis should be placed on reporting estimates of the relationship between tourism and economic growth across a variety of methodological characteristics and specification and estimation choices. The implications of the results for theory development are also discussed.
KW - Economic growth
KW - Meta-regression analysis
KW - Methodology
KW - Publication bias
KW - Tourism-led growth hypothesis
U2 - 10.1177/0047287519844833
DO - 10.1177/0047287519844833
M3 - Journal article
AN - SCOPUS:85066825129
SN - 0047-2875
JO - Journal of Travel Research
JF - Journal of Travel Research
Click through the PLOS taxonomy to find articles in your field.
For more information about PLOS Subject Areas, click here .
Loading metrics
Open Access
Peer-reviewed
Research Article
Tourism cooperation in the Belt and Road Initiative from economic and spatial insights
Roles Conceptualization, Writing – original draft
Affiliation Department of Exhibition Economy and Management, Huaqiao University, Quanzhou, China

Roles Project administration, Supervision
* E-mail: [email protected]
Affiliation Department of Management Sciences, Tamkang University, New Taipei, Taiwan
Roles Data curation, Writing – review & editing
- Jie Yin,
- Yensen Ni,
- Yangchu Fan
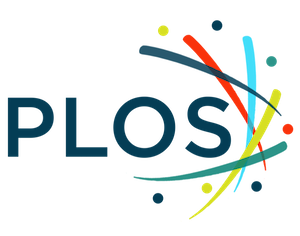
- Published: May 20, 2024
- https://doi.org/10.1371/journal.pone.0300392
- Reader Comments
This study examines the potential benefits of cooperation among Belt and Road Initiative (BRI) countries in achieving common goals within the international tourism cooperation network. Despite its significance, limited research has been conducted on this topic in terms of economic and spatial insights. To address this gap, we utilized the gravity model, social network, and quadratic regression. The revealed findings suggest that while the intermediary function among BRI countries is declining, the tourism cooperation network is gradually strengthening. Furthermore, reducing the gap between the governance and consumption levels of BRI countries can improve the network. The study offers new insights into the BRI tourism cooperation network, which could be critical for the future growth of regional tourism.
Citation: Yin J, Ni Y, Fan Y (2024) Tourism cooperation in the Belt and Road Initiative from economic and spatial insights. PLoS ONE 19(5): e0300392. https://doi.org/10.1371/journal.pone.0300392
Editor: Ricky Chee Jiun Chia, Universiti Malaysia Sabah, MALAYSIA
Received: August 16, 2023; Accepted: February 27, 2024; Published: May 20, 2024
Copyright: © 2024 Yin et al. This is an open access article distributed under the terms of the Creative Commons Attribution License , which permits unrestricted use, distribution, and reproduction in any medium, provided the original author and source are credited.
Data Availability: All relevant data are available within the paper and its Supporting Information files.
Funding: "The authors appreciate the funding from Huaqiao University's academic project supported by the Fundamental Research Funds for the Central Universities (23SKGC-QT01) to JY. The funders had no role in study design, data collection and analysis, decision to publish, or preparation of the manuscript."
Competing interests: The authors have declared that no competing interests exist.
1. Introduction
The absence of regional coordination and collaboration in tourism growth can lead to destination competition and slow economic development [ 1 ]. In contrast, cooperation between organizations and countries can result in advantageous partnerships that facilitate the attainment of shared objectives [ 2 ]. Previous research argued that regional tourism cooperation is the current trend of tourism development [ 3 ]. Consequently, numerous studies have explored the benefits of cooperation in an increasing range of fields, such as public administration [ 4 ] and tourism [ 5 , 6 ]. Specifically, research in tourism cooperation has examined various aspects, including tourism attractions [ 7 ], tourism organizations [ 5 ], tourism destinations [ 8 , 9 ], tourism enterprises [ 10 ], and tourism marketing [ 1 ]. Moreover, a country’s authority should improve transportation and facilities to enhance tourism cooperation with other countries [ 11 ]. In recent decades, cross-border tourism cooperation has also witnessed growth [ 12 – 16 ].
In the context of measuring tourism cooperation relationships, network theory posits that a network is composed of actors or "nodes," as well as the connections or "edges" between these actors [ 17 , 18 ]. Actors within a network have various types of ties, including communication ties, formal ties, affective ties, material or workflow ties, proximity ties, and cognitive ties [ 17 ]. Network theory is commonly used to explain how countries interact, relate to one another, and evolve within a complex network [ 19 ]. However, most existing tourism cooperation studies focus on small-scale cooperation networks, such as destination stakeholder cooperation networks [ 5 ] and tourism enterprise cooperation networks [ 8 , 10 ], with little attention paid to large-scale international tourism cooperation networks [ 15 ]. Therefore, we employ network theory to examine a large-scale international tourism cooperation network among BRI countries, which has been largely overlooked in current literature.
Furthermore, previous research has delved into the cooperation among countries across a range of sectors, including the economy and tourism [ 20 ]. Over the past few decades, tourism cooperation has become increasingly important due to its positive impact on the economy, including employment growth, increased income, and higher consumption in the tourism sector [ 21 , 22 ]. However, as previous research has primarily focused on regional [ 23 ] and national cooperation [ 24 ], we contend that exploring tourism cooperation with BRI countries warrants further investigation. Many studies on tourism cooperation not only examine ways to enhance cooperation among BRI countries [ 25 ] but also explore tourism cooperation across various spatial scales.
We argue that this study may contribute to the existing literature. Firstly, unlike previous studies that focused on tourism enterprise cooperation networks, tourism research cooperation networks, and tourism stakeholders’ cooperation networks [ 26 ], we deconstruct a tourism cooperation network among BRI nations employing this gravity model and social network analysis. Second, in addition to uncovering various critical influential factors affecting such a network, we disclosed that narrowing the gap between BRI countries’ governance and consumption levels will enhance the network. Third, this network is steadily strengthening, and the function of "intermediary" among BRI countries is decreasing, signaling that authorities should direct the tourism cooperation network among BRI countries. We explain the roles of leader, intermediary, and autonomy among BRI countries and separate them into distinct blocks in the tourism cooperation network, including broker, sycophants, primary, and isolate blocks, both of which are underrepresented in the previous research.
The study aims to investigate two critical areas of concern. Firstly, it seeks to explore this network from 2000 to 2018 through a comprehensive analysis of global, small-group, and individual perspectives. Secondly, it intends to identify and examine the key driving factors of the tourism cooperation network in the BRI region. By addressing these two objectives, this study aims to contribute to the existing body of knowledge on BRI tourism cooperation and provide valuable insights for policymakers and practitioners in the tourism industry.
2. Research design
2.1. tourism cooperation ties measurement.
The gravity model, initially proposed in the 1960s, has continued to be widely applied for assessing network ties across various fields, including trade and urban development. Researchers have recently focused on studying economic relationships using the gravity model, and its effectiveness has been demonstrated in studies investigating economic links, making it a well-regarded model.
2.2. Social network analysis
Given the ability of social network analysis to depict node relationships in a network, we employ this methodology to gauge both the collective and individual attributes of the network [ 7 , 32 ]. Additionally, we employ the quadratic assignment procedure (QAP) approach [ 7 ], to further explore the principal factors driving the tourism cooperation network. Hence, we provide a succinct overview of the measurement of the network’s general, small group, and individual features, as well as the QAP analysis methodology, as follows.
- Measuring the network’s overall characteristics: To measure the characteristics of the tourism cooperation network, several metrics can be used, such as network density, centralization, and E-I index [ 33 ]. Network density, which is the ratio of the actual number of links to the maximum number of possible links in the network [ 34 ], can provide insights into the connectivity of network entities. The higher the network density, the closer the entities are connected [ 35 ]. In addition, individual characteristics can be assessed through centralization measures such as degree centralization, betweenness centralization, and closeness centralization. These measures can indicate the degree of concentration within the network [ 35 ]. Another important metric is the E-I index, which is used to evaluate the level of the faction within the cooperation network. The E-I index has a possible range of -1 to 1, with a higher number indicating a larger degree of faction within the network and a lower value indicating a lesser degree of faction. The E-I index can also be negative. A figure that is close to zero may indicate that the faction’s position inside the corporate network is unclear [ 35 ].
- Measuring the network’s small-group characteristics: An appropriate way for analyzing the features of small groups inside a network is to use the block model [ 36 ]. In order to assist understand the internal dynamics of small groups, Burt (1979) postulated four separate network positions, which included the isolate block, the sycophants block, the broker block, and the primary block [ 37 ]. An isolate block is characterized by limited interaction between members and the outside world, while a sycophants block features weaker internal ties than external ones. A broker block is defined by members who facilitate external relationships more than internal ones, while a primary block consists of members who primarily establish either external or internal relationships [ 37 ]. With the aim of evaluating small group cooperation within the BRI tourism cooperation network, we leverage the block model as a suitable analytical tool.
- Measuring the network’s individual characteristics: In the evolution of a network, the structural hole, as measured by various indicators, plays a crucial role [ 38 , 39 ]. Betweenness, effective size, efficiency, and constraints are common metrics used to measure the structural hole. Betweenness measures an organization’s ability to control information and act as an intermediary in a network. A higher value of betweenness indicates better control and mediation ability of members. Effective size is another indicator of control power, which measures the extent to which a node can influence others in the network. A higher effective size value suggests that the node has strong control power and is efficient in influencing other nodes [ 40 ]. Therefore, it is important to assess these metrics to understand the role of the structural hole in shaping the dynamics of a network.
- The QAP regression: This study uses Quadratic Assignment Procedure (QAP) regression to analyze the causal link between the variables, which may effectively address the issue of multicollinearity [ 41 ]. The correlation coefficient is calculated using QAP regression. This aids in identifying the important aspects influencing the tourist collaboration network. This study can rapidly analyze the impact of several factors on the network topology using QAP regression while avoiding the issue of multicollinearity, which is a prevalent difficulty in regression analysis. As such, this technique may help us to get insights into the complex interactions between variables and their significance in shaping the BRI tourist cooperation network.
2.3. Selection of driving factors
Tourism activities may be significantly influenced by the distinction between the tourist source nation and the destination nation. As a result, we looked into the major determinants that affect the tourism cooperation network among BRI countries, concentrating on the differences across eight factors: security (SD), economics (ED), land adjacency (LA), tourism openness (TOD), population density (PDD), language (LD), consumption level (CLD), and governance (GD). These factors were chosen to develop a thorough knowledge of the motivations that drive international tourist cooperation while taking into consideration various ways that countries vary from one another.
- Security difference (SD): When evaluating the driving factors affecting the tourism cooperation network among BRI countries, one crucial element is the differences in tourism security between the tourist source and destination countries. This factor has been shown to influence international tourism collaboration [ 16 ]. Intentional homicide rates are frequently used to gauge a country’s security situation. To assess the occurrence of a security gap between two BRI nations, we compute the average difference in intentional homicide rates over 19 years (2000–2018). This 19-year average difference serves as the baseline against which we measure each year. If the difference in intentional homicide rates between two BRI countries is less than the benchmark, we set SD as 0. This allows us to assess the impact of security differences on the tourism collaboration network among BRI countries.
- Economic difference (ED): Differences in economic factors such as price, income, and currency rates can have a significant impact on tourist flow [ 42 , 43 ]. As a proxy for determining the economic difference (ED) that exists between the two countries in the BRI network, we utilize the difference in gross national income (GNI). Specifically, we compare the GNI difference between the two countries with the benchmark, and if the difference is less than the benchmark, we set ED to 1. By using GNI as a proxy, we can effectively capture the economic situation and its potential influence on tourism activities in BRI countries.
- Land adjacency (LA): Geographical distance, particularly land adjacency, is a critical factor in influencing tourism activities between countries. Studies have shown that neighboring countries have a significant impact on trade volume [ 44 ] and tourists’ destination choices [ 7 ]. Therefore, we use land adjacency as a proxy for the LA variable. If the two countries being compared are bordering countries, we assign LA a value of 1.
- Tourist openness difference (TOD): Tourism openness (TO) is a crucial factor that influences tourism collaboration as it reflects the level of tourism development [ 45 ]. Cultural exchange and the positive image of a place can be promoted by tourism openness, leading to increased tourist inflow [ 46 ]. The measure of tourism openness is calculated as the sum of inbound and outbound tourism openness, weighted equally to represent the degree of tourism openness for a country. The inbound (outbound) tourism openness is computed as inbound (outbound) tourism revenue (spending) divided by the GDP of the country [ 47 ]. If the difference in tourism openness between two countries is less than the benchmark, we assign TOD a value of 0.
- Population density difference (PDD): The population density of a country is an important determinant of tourism demand, as higher population densities are often associated with a greater propensity for overseas travel. Thus, we include population density as a driving factor in our analysis of tourism cooperation among BRI countries. Specifically, we calculate the population density difference between two distinct nations, and if this difference is smaller than the benchmark, we assign a value of 0 to PDD. Previous research has highlighted the value of population density as a predictor of tourism demand [ 48 , 49 ], emphasizing its importance as a factor in our investigation of the BRI tourism network.
- Language difference (LD): From a cross-cultural perspective, differences can play a significant role in boosting tourism activities [ 50 , 51 ]. Among these differences, language is considered a crucial factor since it has a significant impact on international tourism activities and enhances the tourism experience [ 50 , 52 ]. Tourists tend to travel to countries where they can communicate in the same language [ 53 ]. Thus, we consider the language difference as a crucial driving factor affecting tourism cooperation. Specifically, if two distinct BRI countries share the same official language, we set LD to 1.
- Consumption level difference (CLD): The level of consumption in a destination may reflect the cost of a trip to that destination. Given that tourism activities are significantly impacted by the cost of traveling, Timothy and Kim (2015) argued that travel expenses are one of the most important factors influencing tourism demand [ 16 ]. To assess the level of consumption, we utilize per capita consumption. If the difference in per capita consumption between two distinct BRI countries is less than the benchmark, we set CLD to 0.
- Governance difference (GD): To enhance tourism development, a country must have robust governance structures in place [ 54 , 55 ]. Therefore, governance difference (GD) is a crucial factor to consider in tourism promotion [ 56 ]. To measure GD, the World Bank employs six indicators including voice and accountability, political stability and absence of violence/terrorism, government effectiveness, regulatory quality, rule of law, and control of corruption. Prior research suggested averaging these six indicators to assess the governance ability of BRI countries [ 57 ]. If the difference between the governance abilities of two BRI countries is lower than the benchmark, GD is set to 0.
2.4. Data sources
The primary objective of tourism cooperation among BRI members is to increase tourist inflows, thereby generating higher tourism income for these countries. To achieve this objective, we use international tourist arrivals and international tourism income as important indicators rather than total tourist arrivals and tourism income in a country, as Eq ( 1 ) demonstrates.
To assess the industrial development environment, this study uses service employment as a percentage of total employment [ 47 ]. The data for this analysis was obtained from the World Bank’s World Development Indicators database. Additionally, the CEPII database was used to calculate the spatial distance between two capitals as a measure of the geographic distance between two BRI countries. A total of 60 BRI countries were included in this analysis over the 2000–2018 period, as data was unavailable for six countries, namely Iraq, Syria, Afghanistan, Turkmenistan, and Uzbekistan. Due to the difficulty in creating country-pairs data, these 60 BRI countries were either considered as source or destination countries in this study.
In terms of the driving factors, intentional homicide rates were collected from the United Nations Office on Drugs and Crime’s International Homicide Statistics database ( http://www.unodc.org/ ) to assess a country’s security. The World Bank provided data on inbound tourism revenue, outbound tourism spending, GDP, population density, per capita consumption, gross national income (GNI), and governance including voice and accountability, political stability and absence of violence/terrorism, government effectiveness, regulatory quality, rule of law, and control of corruption. Additionally, data on the official languages of BRI countries was sourced from the French CEPII database. The study collected data from 2000 to 2018 for this analysis.
3. The international tourism cooperation’s characteristics
3.1. the network’s overall characteristics.
We have constructed a tourism cooperation relationship matrix using the revised gravity model and have visually represented the tourism cooperation network for four stages, specifically in 2000, 2006, 2012, and 2018, as illustrated below ( Fig 1 ) by employing the Ucinet and Netdraw software. The average value of tourism collaboration ties from 2000 to 2018 was utilized as the breakpoint value in this analysis so that we could assess the value of the relationships between the tourism organizations. If the value between two BRI nations is greater than the mean, this study sets the value of the relationship to 1, and if it was lower than the average value, we set it to 0. It can be seen that the network of tourism collaboration is getting increasingly intertwined as the number of cooperation relations between BRI countries increased from 1308 in the year 2000 to 1423 in the year 2006, 1693 in the year 2012, and 1737 in the year 2018. The steady increase in the number of cooperation ties over the years suggests the potential for further growth and collaboration within the tourism industry.
- PPT PowerPoint slide
- PNG larger image
- TIFF original image
(a) 2000, (b) 2006, (c) 2012 and (d) 2018.
https://doi.org/10.1371/journal.pone.0300392.g001
In addition, this study employs network density, E-I index, and centralization to evaluate the comprehensive properties of the tourism collaboration network across the BRI. The outcomes of this analysis are illustrated in Fig 2 .
https://doi.org/10.1371/journal.pone.0300392.g002
Regarding network density, our findings reveal a gradual increase from 0.3574 in 2000 to 0.4746 in 2018, indicating an increment in the extent of interconnectivity among the participating countries. The centralization of the network displayed a slow increase over time but remained below 40%, indicating the absence of any dominant player in the network. However, the betweenness centralization declined, suggesting that the role of intermediaries has diminished due to increased direct collaboration among the member states. This is a noteworthy finding that could impact future tourism-related policies and cooperation. Additionally, the closeness centralization showed a fluctuation of around 30% higher than other characteristics, indicating that the links between "central countries" and "edge countries" (In this study, central countries are defined as those that may have a strong economy and consumption power, whereas edge countries are those that may not have a strong economy and consumption power) need strengthening. Furthermore, the E-I index exhibited a narrow range of fluctuation around -0.4, indicating the possibility of fractional relations among the BRI countries. We detected patterns of small group cooperation in the network, highlighting opportunities to enhance overall tourism collaboration in the future.
3.2. The network’s small group characteristics
The E-I index has revealed that the tourism cooperation network consists of small groups. To explore this further, we have utilized the Convergence of Iterated Correlations (CONCOR) method to cluster the BRI countries, with a maximum segmentation of 2 and a convergence criterion of 0.2. Through this method, we have successfully partitioned the selected 60 BRI countries into four distinct blocks [ 40 ]. Before discussing our findings, we will first elucidate the roles played by each of these blocks.
3.2.1. The role of various blocks.
Based on the image matrix for each year, this study has developed a succinct relationship diagram depicting the roles of various blocks, namely block 1, block 2, block 3, and block 4, as presented in Fig 3 . The arrow situated above each block represents the relationship "sent" by a block, which "returns" to this block (Some members in a block contact with other members in the block each other, indicating some members "send" messages to other members, and other members "return" messages to some members after receiving the message), suggesting that each block has specific relationships with internal members.
https://doi.org/10.1371/journal.pone.0300392.g003
Fig 3 reveals that block 1 not only possesses relationships among internal members but also accepts the relationships initiated by block 2 and sends the relationship to block 4. This observation indicates that block 1 may function as a "bridge" to facilitate communication between block 2 and block 4, rendering it the broker block. Conversely, block 4 may be considered the sycophant block since it frequently receives and sends relations for other blocks, including block 1 and block 3, instead of its own.
Concerning block 3, it can be discerned that it serves as the primary block because it not only sends and receives relationships from block 4 but also has a close relationship with its internal members. Moreover, block 2 could be considered the isolate block since, compared to other blocks, it seldom receives and sends relationships to other blocks.
3.2.2. The members of the various blocks.
In this study, we employed Ucinet software to calculate the density between blocks, assigning a value of 1 if the density was greater than 1 and 0 otherwise. Using this information, we constructed an image matrix to analyze the relationship between blocks and divided BRI countries into various blocks. As demonstrated in Fig 4 , the members in these blocks vary across the 2000–2018 timeframe.
https://doi.org/10.1371/journal.pone.0300392.g004
As depicted in Fig 4 , the number of members in the isolate blocks gradually decreased over time, indicating that the isolate role may no longer be suitable for current cooperation mainstreams. On the other hand, the number of members in the prime block has shown an upward trend, suggesting that there has been an improvement in tourism cooperation among BRI countries. However, the overall number of brokers has decreased, indicating that intermediaries may no longer play as critical a role in tourism cooperation as they once did.
In addition, it can be observed from Fig 4 that the members in the sycophants block exhibit a rising trend from 2000 to 2009, followed by two decreasing trends after 2009, indicating a higher level of volatility compared to other blocks. It is noteworthy that by the end of the data period, the number of "outward-oriented" members in the sycophants block, i.e., those with a tendency to receive and send relationships for other blocks, dropped to less than ten nations for tourism cooperation.
Overall, this study suggests that the interaction among BRI countries, including direct cooperation, has been improving from 2000 to 2018, as evidenced by the upward trend of the primary block, which contains more countries compared to other blocks, as shown in Fig 4 . In this study, 60 BRI nations were categorized into different blocks for the tourism cooperation network in 2018, including the broker block, isolate block, primary block, and sycophants block, as illustrated in Table 1 .
https://doi.org/10.1371/journal.pone.0300392.t001
3.3. The network’s individual characteristics
This study has evaluated the individual characteristics of the BRI countries, such as effective scale, efficiency, constraints, and centrality among these countries. The findings are presented in Table 2 .
https://doi.org/10.1371/journal.pone.0300392.t002
Our analysis reveals a decline in the role of "intermediaries" for BRI countries over the years, as shown by the decrease in the maximum centrality value from 8.865 (Russia) in 2000 to 5.105 (China) in 2018. This trend suggests that direct cooperation among BRI countries is gaining prominence, reducing the need for intermediaries.
We also observe a trend of increased cooperation and intensity among the BRI countries, as indicated by the rising effective scale values in Table 2 , irrespective of a country’s minimum and maximum scale. China, in particular, has consistently held the top rank in the effective scale since 2010, signifying its crucial role in the BRI tourism cooperation network.
In terms of efficiency, our analysis demonstrates a changing trend in the most dominant country, with Russia, Jordan, Ukraine, Thailand, and China taking on this role at different times. However, the overall efficiency of the BRI network is declining, implying a shift towards equality and balance among the participating countries.
Finally, our analysis indicates a gradual decrease in constraints for the BRI countries, reflecting the improvement in cooperation liberalization and facilitation. We suggest that countries with lower levels of cooperation liberalization, such as Tajikistan, Myanmar, Bangladesh, Pakistan, Cambodia, and Yemen, should increase their cooperation with other countries to further enhance their tourism networks.
4. The BRI tourism cooperation network’s driving factors
Utilizing QAP regression, this study examines the potential influences of security difference (SD), economic difference (ED), land adjacency (LA), tourism openness difference (TOD), population density difference (PDD), language difference (LD), consumption level difference (CLD), and governance difference (GD) on the tourism cooperation network among BRI countries. The findings, as shown in Table 3 , shed light on the impact of those factors on tourism cooperation.
https://doi.org/10.1371/journal.pone.0300392.t003
We find that, except for the 2010–2013 period, security differences do not significantly affect tourism cooperation. This result could be attributed to increased attention to tourist security among countries. Thus, other factors may play a more significant role in promoting tourism cooperation. Conversely, economic differences demonstrate a positive and robust impact on tourism cooperation. This finding suggests that countries with varying national incomes are more likely to cooperate in tourism, as tourists may prefer to visit countries with diverse infrastructure, pricing, consumption levels, and other factors.
Our analysis reveals that land adjacency has a favorable impact on tourism cooperation from 2000 to 2004, indicating that boundary tours continue to dominate tourism cooperation. However, the effect of land adjacency on tourism cooperation is gradually decreasing, as seen in the falling coefficients from 2005 to 2018. Thus, tourism cooperation may eventually break through regional cooperation and expand to global cooperation.
Tourism openness, defined as the percentage of incoming/outgoing tourism revenue and spending to GDP, is negatively and significantly related to tourism cooperation only in 2003 and 2004, suggesting that tourism openness differences may not weaken tourism cooperation after 2004. Population density differences have a complex influence on tourism cooperation, as we observe favorable impacts from 2000 to 2004 and from 2013 to 2017, but detrimental effects from 2005 to 2012 and 2018. This finding implies that population density differences may hinder tourism activity.
Language differences have a favorable impact on tourism cooperation, with positive effects observed from 2002 to 2007 and throughout the research period. This finding indicates that differences in traditional culture, humanistic environment, and resource endowment may attract tourists and promote tourism cooperation. In contrast, differences in consumption levels tend to impair tourism collaboration, suggesting that tourism cooperation may not be strengthened if countries have vastly different consumption levels. This finding could be attributed to tourists’ quality concerns and budget constraints.
Finally, we find that governance differences are consistently negatively related to tourism cooperation year after year, highlighting governance as a significant impediment to tourism cooperation. The development of tourism is aided by strong governance, but considerable governance differences between countries could severely hinder tourism cooperation. Therefore, we suggest that BRI nations’ governments should strive to improve their governance to enhance tourism cooperation.
5. Concluding remarks
5.1. conclusion and discussion.
The study aimed to deconstruct the tourism cooperation network among Belt and Road Initiative (BRI) countries by employing the modified gravity model and social network analysis to analyze the network’s characteristics and driving factors. Our results provide a comprehensive overview of the tourism cooperation network among BRI countries and reveal several crucial findings.
First, we find that the tourism cooperation network among BRI countries is becoming more closely connected, which may lead to a decrease in the importance of leadership and brokers within the network. The network’s increasing autonomy and the existence of small-group cooperation suggest that no single entity can dominate the network. This close tourism cooperation can be attributed to the growth of international tourism cooperation and frequent international tourist flows, resulting in greater tourism collaboration among BRI countries. To some extent, the close tourism cooperation among BRI countries may be attributed to growing international tourism cooperation [ 58 ] as well as frequent international tourist flows [ 19 , 59 ]. That is, the closer tourism cooperation among BRI countries may result from the rapid growth of international tourism [ 52 ].
Second, our analysis indicates that the tourism cooperation network consists of brokers, sycophants, primary, and isolated blocks, and small group cooperation exists within the network. Our findings demonstrate that isolated block members are decreasing while primary block members are generally increasing, indicating that the isolation function is not suitable for current tourism collaboration among BRI countries. Additionally, Central Asia and Islamic countries have been gaining increasing attention in tourism cooperation. A cooperative relationship is a viable alternative, whereas isolation may be impractical [ 10 ]. Recently, in addition to BRI tourism cooperation, regional cooperation such as China-ASEAN [ 60 ], Central Asia [ 61 ], and Islamic nations [ 62 ] has received much more attention.
Third, we find that cooperation limitations among BRI nations are gradually decreasing, and the trend toward liberalized cooperation is becoming more apparent. The betweenness of intermediaries has weakened, indicating that direct linking between BRI countries would be more visible. Positive collaboration effects may make direct cooperation popular [ 63 , 64 ], leading to the reduction of cooperation constraints or the role of intermediaries. As the restraints on BRI countries are steadily eliminated, the tourism cooperation network will become more liberated, and some countries with major influence will shrink, leading to greater equality within the network. China plays a significant role in the tourism cooperation network, as indicated by its top ranking in efficiency and its position as the world’s largest provider of outbound tourism and a major recipient of tourists. Since 2012, China has become the world’s largest source of outbound tourism [ 65 ] as well as one of the world’s primary recipients of tourists [ 66 ], since the number of inbound visitors has increased dramatically.
Finally, our QAP regression analysis identifies differences in security, tourism openness, population density, consumption level, and governance as negative factors affecting the tourism cooperation network among BRI countries, while differences in economics, language, and land adjacency have positive effects, which is consistent with previous research [ 50 , 52 , 67 ].
In conclusion, our study provides a comprehensive deconstruction of the tourism cooperation network among BRI countries, offering insights into the characteristics and driving factors of the network. Our findings suggest that increased direct linking, reduced cooperation constraints, and greater equality within the network will facilitate more extensive and effective tourism collaboration among BRI countries.
5.2. Research implications
Based on the study’s findings, we found several implications that could have a substantial impact on the tourism industry in BRI countries. First, the research recommends that BRI countries focus on enhancing their tourism marketing and management. Given that tourism is less harmful and provides limitless business prospects, it represents a fantastic chance for these countries to enhance their economies and living standards. However, to attract tourists, it is critical to improve not only marketing activities, but also tourism infrastructure management, such as roads, rest spots, and traffic. This can be accomplished by increasing the number of management employees and giving tourists clear recommendations for navigating the destination.
Second, the use of social media platforms like Facebook, YouTube, and Twitter to promote tourist destinations and make a favorable impression on tourists is an appealing option. In addition, word-of-mouth advertising is an efficient method for promoting a company that also can bring in a greater number of customers. As a result, the tourism industry can stand to gain from this form of advertising if it is done well.
Third, because the association between poverty and social unrest may not be low, this study anticipates that the economy of those countries will improve, resulting in a drop in those living in poverty and an increase in those able to have a better living standard. Thus, the tourism industry can contribute to the overall well-being of these countries by providing economic opportunities for the local population.
However, it is important to note that the severe impact of COVID-19 may have long-lasting effects on the tourism industry and the benefits outlined above may be difficult to demonstrate in the short term. Despite this, we believe that the findings of this study can help guide future efforts to promote and develop the tourism industry in BRI countries.
5.3. Limitations and future research
Despite uncovering the key characteristics and driving sources of the network, this study is not without limitations. First, while we employed a range of methodologies such as the revised gravity model and social network analysis, which have been supported by previous studies, we must still justify why our methods are superior in deconstructing the tourism cooperation network. Thus, further research is necessary to examine this issue thoroughly. Second, language differences serving as a proxy for culture, which is crucial factor influencing tourism cooperation, may be considered a limitation of this study. In future research, it may be worthwhile to explore alternative proxies for the culture that could provide more accurate assessments. Third, given the establishment of regional cooperation organizations, our study identifies the existence of small cooperative groups within the tourism cooperation network. Further investigation into the essential cooperative characteristics of these small groups and the mechanisms of tourism cooperation formation among them could provide valuable insights for future research.
Supporting information
https://doi.org/10.1371/journal.pone.0300392.s001
- View Article
- Google Scholar
- 18. Wasserman S, Faust K. Social network analysis: Cambridge university press; 1994.
- PubMed/NCBI

Tourism and Economic Growth: A Meta-regression Analysis
Mauritius - 27 September 2019
Trade topics: Tourism
Numerous studies have focused on delineating the relationship between tourism and economic growth. In this article, we present the results of a rigorous meta-regression analysis based on 545 estimates drawn from 113 studies that empirically tested the tourism-led growth hypothesis (TLGH). The results suggest the presence of publication bias in the literature on this topic, where the majority of studies report positive and statistically significant estimates. Findings provide support for the TLGH, but they also suggest that the estimates are sensitive to a number of factors that are related to country data, specification, and estimation characteristics, and time span. Such sensitivities suggest that greater emphasis should be placed on reporting estimates of the relationship between tourism and economic growth across a variety of methodological characteristics and specification and estimation choices. The implications of the results for theory development are also discussed.
- JTR Meta (3).pdf
Author(s): Nunkoo, Seetanah, Z K Jaffur, Moraghen and Sannassee
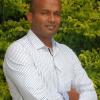
Boopen Seetanah
- Publications
- Key Findings
- Interactive data and economy profiles
- Full report
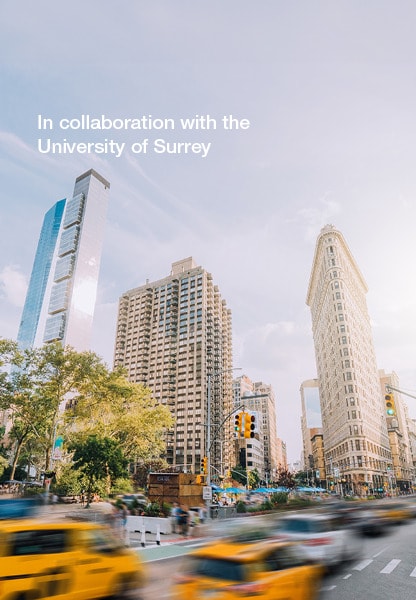
Press release and related contributions
Tourism is back to pre-pandemic levels, but challenges remain.
- High-income economies in Europe and Asia-Pacific continue to lead the World Economic Forum Travel and Tourism Index, with the United States, Spain and Japan topping the rankings again.
- Despite post-pandemic growth, the global tourism sector still faces complex challenges, with recovery varied by region; only marginal overall score improvements since the 2021 edition.
- Developing economies are making strides – who account for 52 out of 71 economies improving since 2019 – but significant investment is needed to bridge gaps and increase market share.
- Read the report here .
New York, USA, 21 May 2024 – International tourist arrivals and the travel and tourism sector’s contribution to global GDP are expected to return to pre-pandemic levels this year, driven by the lifting of COVID-19-related travel restrictions and strong pent-up demand, as per the new World Economic Forum travel and tourism study, released today.
Topping the 2024 list of economies are the United States, Spain, Japan, France and Australia. The Middle East had the highest recovery rates in international tourist arrivals (20% above the 2019 level), while Europe, Africa and the Americas all showed a strong recovery of around 90% in 2023.
These are some of the top findings of the Travel & Tourism Development Index 2024 (TTDI) , a biennial report published in collaboration with the University of Surrey, which analyses the travel and tourism sectors of 119 countries around a range of factors and policies.
“This year marks a turning point for the travel and tourism sector, which we know has the capacity to unlock growth and serve communities through economic and social transformation,” said Francisco Betti, Head of the Global Industries team at the World Economic Forum. “The TTDI offers a forward-looking window into the current and future state of travel and tourism for leaders to navigate the latest trends in this complex sector and sustainably unlock its potential for communities and countries across the world.”
Post-pandemic recovery
The global tourism industry is expected to recover from the lows of the COVID-19 pandemic and surpass the levels seen before the crisis. This is largely being driven by a significant increase in demand worldwide, which has coincided with more available flights, better international openness, and increased interest and investment in natural and cultural attractions.
However, the global recovery has been mixed. While 71 of the 119 ranked economies increased their scores since 2019, the average index score is just 0.7% above pre-pandemic levels.
Although the sector has moved past the shock of the global health crisis, it continues to deal with other external challenges, from growing macroeconomic, geopolitical and environmental risks, to increased scrutiny of its sustainability practices and the impact of new digital technologies, such as big data and artificial intelligence. In addition, labour shortages are ongoing, and air route capacity, capital investment, productivity and other sector supply factors have not kept up with the increase in demand. This imbalance, worsened by global inflation, has increased prices and service issues.
TTDI 2024 highlights Out of the top 30 index scorers in 2024, 26 are high-income economies, 19 are based in Europe, seven are in Asia-Pacific, three are in the Americas and one (the United Arab Emirates) is in the Middle East and North Africa region (MENA). The top 10 countries in the 2024 edition are the United States, Spain, Japan, France, Australia, Germany, the United Kingdom, China, Italy and Switzerland.
The results highlight that high-income economies generally continue to have more favourable conditions for travel and tourism development. This is helped by conducive business environments, dynamic labour markets, open travel policies, strong transport and tourism infrastructure, and well-developed natural, cultural and non-leisure attractions.
Nevertheless, developing countries have seen some of the greatest improvements in recent years. Among the upper-middle-income economies, China has cemented its ranking in the top 10; major emerging travel and tourism destinations of Indonesia, Brazil and Türkiye have joined China in the top quartile of the rankings. More broadly, low- to upper-middle-income economies account for over 70% of countries that have improved their scores since 2019, while MENA and sub-Saharan Africa are among the most improved regions. Saudi Arabia and the UAE are the only high-income economies to rank among the top 10 most improved economies between 2019 and 2024.
Despite these strides, the TTDI warns that significant investment is needed to close gaps in enabling conditions and market share between developing and high-income countries. One possible pathway to help achieve this would be sustainably leveraging natural and cultural assets – which are less correlated with country income level than other factors – and can offer developing economies an opportunity for tourism-led economic development.
“It’s essential to bridge the divide between differing economies’ ability to build a strong environment for their travel and tourism sector to thrive,” said Iis Tussyadiah, Professor and Head of the School of Hospitality and Tourism Management at the University of Surrey. “The sector has big potential to foster prosperity and mitigate global risks, but that potential can only be fully realized through a strategic and inclusive approach.”
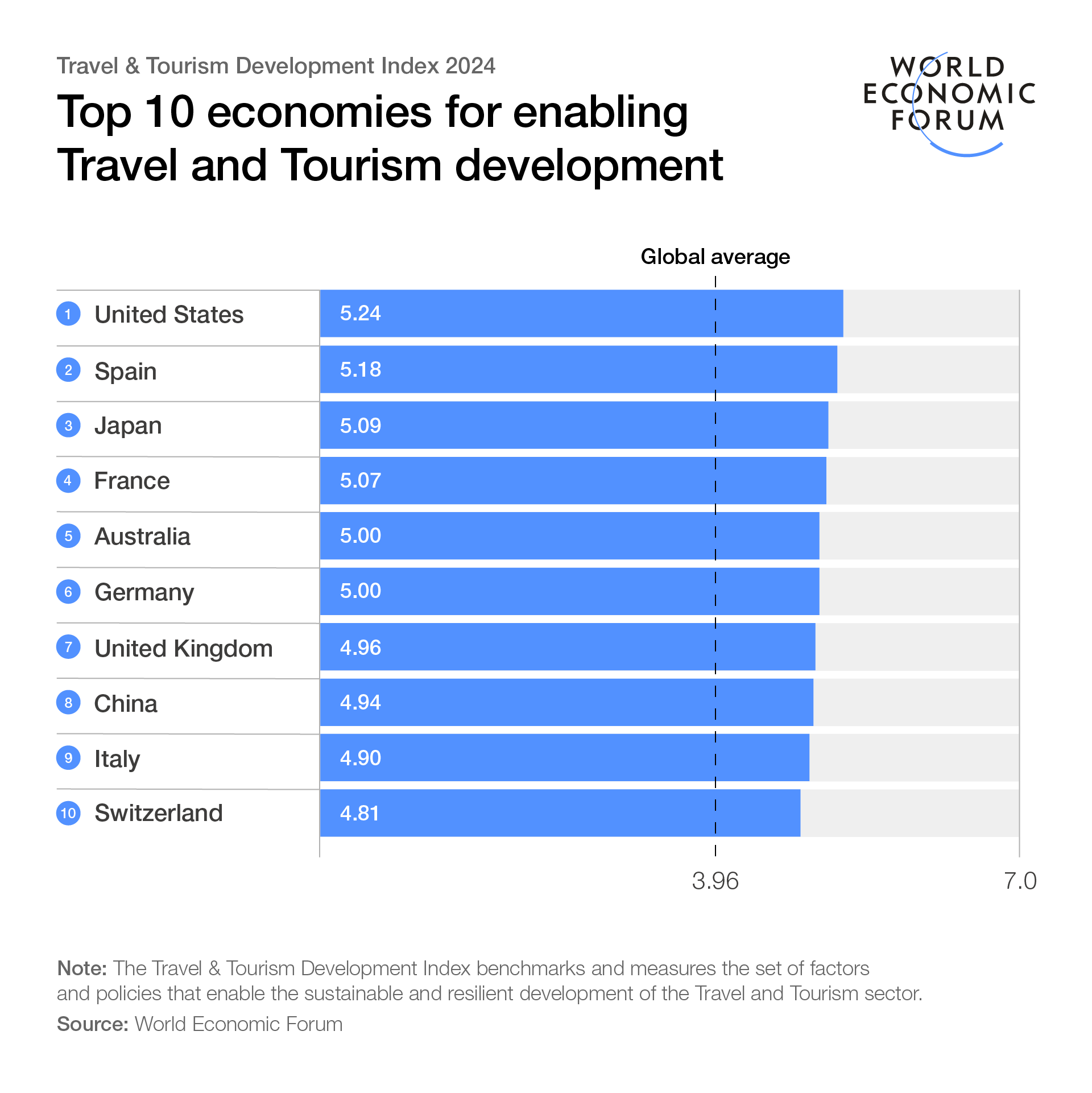
Mitigating future global challenges
According to the World Economic Forum’s 2024 Global Risks Report, the travel and tourism sector faces various complex risks , including geopolitical uncertainties, economic fluctuations, inflation and extreme weather. Balancing growth with sustainability also remains a major problem, due to high seasonality, overcrowding, and a likely return of pre-pandemic emissions levels. The report also analyses persistent concerns about equity and inclusion. While the tourism sector offers a major source of relatively high-wage jobs, particularly in developing countries, gender parity remains a major issue for regions such as MENA and South Asia.
Despite these challenges, the sector can play a significant role in addressing them. To achieve this, decision-makers should prioritize actions such as leveraging tourism for nature conservation efforts; investing in skilled, inclusive and resilient workforces; strategically managing visitor behaviour and infrastructure development; encouraging cultural exchange between visitors and local communities; and using the sector to bridge the digital divide, among other policies.
If managed strategically, the travel and tourism sector – which has historically represented 10% of global GDP and employment – has the potential to emerge as a key contributor to the well-being and prosperity of communities worldwide.
About the Travel and Tourism Development Index 2024
The 2024 edition of the TTDI includes several improvements based on newly available data and recently developed indicators on the environmental and social impact of travel and tourism. The changes made to the 2024 Index limit its comparability to the previously published TTDI 2021. This year's report includes recalculated 2019 and 2021 results, using new adjustments. TTDI 2024 reflects the latest available data at the time of collection – end of 2023. The TTDI is part of the Forum’s broader work with industry communities actively working to build a better future enabled by sustainable, inclusive, and resilient industry ecosystems.
Notes to editors
Read the Forum Agenda also in Spanish | Mandarin | Japanese Learn about the Forum’s impact Check out the Forum’s Strategic Intelligence Platform and Transformation Maps Follow the Forum on social media: @wef | Instagram | LinkedIn | Facebook | TikTok | Weibo | Threads | WhatsApp
Watch Forum videos at wef.ch/videos | YouTube Get Forum podcasts at wef.ch/podcasts | YouTube Subscribe to Forum news releases
Thank you for visiting nature.com. You are using a browser version with limited support for CSS. To obtain the best experience, we recommend you use a more up to date browser (or turn off compatibility mode in Internet Explorer). In the meantime, to ensure continued support, we are displaying the site without styles and JavaScript.
- View all journals
- Explore content
- About the journal
- Publish with us
- Sign up for alerts
- Published: 08 May 2024
A meta-analysis on global change drivers and the risk of infectious disease
- Michael B. Mahon ORCID: orcid.org/0000-0002-9436-2998 1 , 2 na1 ,
- Alexandra Sack 1 , 3 na1 ,
- O. Alejandro Aleuy 1 ,
- Carly Barbera 1 ,
- Ethan Brown ORCID: orcid.org/0000-0003-0827-4906 1 ,
- Heather Buelow ORCID: orcid.org/0000-0003-3535-4151 1 ,
- David J. Civitello 4 ,
- Jeremy M. Cohen ORCID: orcid.org/0000-0001-9611-9150 5 ,
- Luz A. de Wit ORCID: orcid.org/0000-0002-3045-4017 1 ,
- Meghan Forstchen 1 , 3 ,
- Fletcher W. Halliday 6 ,
- Patrick Heffernan 1 ,
- Sarah A. Knutie 7 ,
- Alexis Korotasz 1 ,
- Joanna G. Larson ORCID: orcid.org/0000-0002-1401-7837 1 ,
- Samantha L. Rumschlag ORCID: orcid.org/0000-0003-3125-8402 1 , 2 ,
- Emily Selland ORCID: orcid.org/0000-0002-4527-297X 1 , 3 ,
- Alexander Shepack 1 ,
- Nitin Vincent ORCID: orcid.org/0000-0002-8593-1116 1 &
- Jason R. Rohr ORCID: orcid.org/0000-0001-8285-4912 1 , 2 , 3 na1
Nature volume 629 , pages 830–836 ( 2024 ) Cite this article
6710 Accesses
607 Altmetric
Metrics details
- Infectious diseases
Anthropogenic change is contributing to the rise in emerging infectious diseases, which are significantly correlated with socioeconomic, environmental and ecological factors 1 . Studies have shown that infectious disease risk is modified by changes to biodiversity 2 , 3 , 4 , 5 , 6 , climate change 7 , 8 , 9 , 10 , 11 , chemical pollution 12 , 13 , 14 , landscape transformations 15 , 16 , 17 , 18 , 19 , 20 and species introductions 21 . However, it remains unclear which global change drivers most increase disease and under what contexts. Here we amassed a dataset from the literature that contains 2,938 observations of infectious disease responses to global change drivers across 1,497 host–parasite combinations, including plant, animal and human hosts. We found that biodiversity loss, chemical pollution, climate change and introduced species are associated with increases in disease-related end points or harm, whereas urbanization is associated with decreases in disease end points. Natural biodiversity gradients, deforestation and forest fragmentation are comparatively unimportant or idiosyncratic as drivers of disease. Overall, these results are consistent across human and non-human diseases. Nevertheless, context-dependent effects of the global change drivers on disease were found to be common. The findings uncovered by this meta-analysis should help target disease management and surveillance efforts towards global change drivers that increase disease. Specifically, reducing greenhouse gas emissions, managing ecosystem health, and preventing biological invasions and biodiversity loss could help to reduce the burden of plant, animal and human diseases, especially when coupled with improvements to social and economic determinants of health.
This is a preview of subscription content, access via your institution
Access options
Access Nature and 54 other Nature Portfolio journals
Get Nature+, our best-value online-access subscription
24,99 € / 30 days
cancel any time
Subscribe to this journal
Receive 51 print issues and online access
185,98 € per year
only 3,65 € per issue
Buy this article
- Purchase on Springer Link
- Instant access to full article PDF
Prices may be subject to local taxes which are calculated during checkout
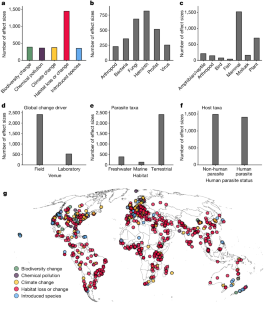
Similar content being viewed by others
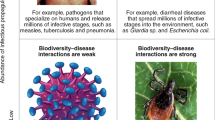
Towards common ground in the biodiversity–disease debate
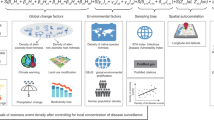
Biological invasions facilitate zoonotic disease emergences
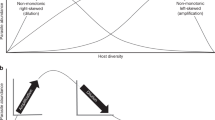
Measuring the shape of the biodiversity-disease relationship across systems reveals new findings and key gaps
Data availability.
All the data for this Article have been deposited at Zenodo ( https://doi.org/10.5281/zenodo.8169979 ) 52 and GitHub ( https://github.com/mahonmb/GCDofDisease ) 53 .
Code availability
All the code for this Article has been deposited at Zenodo ( https://doi.org/10.5281/zenodo.8169979 ) 52 and GitHub ( https://github.com/mahonmb/GCDofDisease ) 53 . R markdown is provided in Supplementary Data 1 .
Jones, K. E. et al. Global trends in emerging infectious diseases. Nature 451 , 990–994 (2008).
Article ADS CAS PubMed PubMed Central Google Scholar
Civitello, D. J. et al. Biodiversity inhibits parasites: broad evidence for the dilution effect. Proc. Natl Acad. Sci USA 112 , 8667–8671 (2015).
Halliday, F. W., Rohr, J. R. & Laine, A.-L. Biodiversity loss underlies the dilution effect of biodiversity. Ecol. Lett. 23 , 1611–1622 (2020).
Article PubMed PubMed Central Google Scholar
Rohr, J. R. et al. Towards common ground in the biodiversity–disease debate. Nat. Ecol. Evol. 4 , 24–33 (2020).
Article PubMed Google Scholar
Johnson, P. T. J., Ostfeld, R. S. & Keesing, F. Frontiers in research on biodiversity and disease. Ecol. Lett. 18 , 1119–1133 (2015).
Keesing, F. et al. Impacts of biodiversity on the emergence and transmission of infectious diseases. Nature 468 , 647–652 (2010).
Cohen, J. M., Sauer, E. L., Santiago, O., Spencer, S. & Rohr, J. R. Divergent impacts of warming weather on wildlife disease risk across climates. Science 370 , eabb1702 (2020).
Article CAS PubMed PubMed Central Google Scholar
Rohr, J. R. et al. Frontiers in climate change-disease research. Trends Ecol. Evol. 26 , 270–277 (2011).
Altizer, S., Ostfeld, R. S., Johnson, P. T. J., Kutz, S. & Harvell, C. D. Climate change and infectious diseases: from evidence to a predictive framework. Science 341 , 514–519 (2013).
Article ADS CAS PubMed Google Scholar
Rohr, J. R. & Cohen, J. M. Understanding how temperature shifts could impact infectious disease. PLoS Biol. 18 , e3000938 (2020).
Carlson, C. J. et al. Climate change increases cross-species viral transmission risk. Nature 607 , 555–562 (2022).
Halstead, N. T. et al. Agrochemicals increase risk of human schistosomiasis by supporting higher densities of intermediate hosts. Nat. Commun. 9 , 837 (2018).
Article ADS PubMed PubMed Central Google Scholar
Martin, L. B., Hopkins, W. A., Mydlarz, L. D. & Rohr, J. R. The effects of anthropogenic global changes on immune functions and disease resistance. Ann. N. Y. Acad. Sci. 1195 , 129–148 (2010).
Rumschlag, S. L. et al. Effects of pesticides on exposure and susceptibility to parasites can be generalised to pesticide class and type in aquatic communities. Ecol. Lett. 22 , 962–972 (2019).
Allan, B. F., Keesing, F. & Ostfeld, R. S. Effect of forest fragmentation on Lyme disease risk. Conserv. Biol. 17 , 267–272 (2003).
Article Google Scholar
Brearley, G. et al. Wildlife disease prevalence in human‐modified landscapes. Biol. Rev. 88 , 427–442 (2013).
Rohr, J. R. et al. Emerging human infectious diseases and the links to global food production. Nat. Sustain. 2 , 445–456 (2019).
Bradley, C. A. & Altizer, S. Urbanization and the ecology of wildlife diseases. Trends Ecol. Evol. 22 , 95–102 (2007).
Allen, T. et al. Global hotspots and correlates of emerging zoonotic diseases. Nat. Commun. 8 , 1124 (2017).
Sokolow, S. H. et al. Ecological and socioeconomic factors associated with the human burden of environmentally mediated pathogens: a global analysis. Lancet Planet. Health 6 , e870–e879 (2022).
Young, H. S., Parker, I. M., Gilbert, G. S., Guerra, A. S. & Nunn, C. L. Introduced species, disease ecology, and biodiversity–disease relationships. Trends Ecol. Evol. 32 , 41–54 (2017).
Barouki, R. et al. The COVID-19 pandemic and global environmental change: emerging research needs. Environ. Int. 146 , 106272 (2021).
Article CAS PubMed Google Scholar
Nova, N., Athni, T. S., Childs, M. L., Mandle, L. & Mordecai, E. A. Global change and emerging infectious diseases. Ann. Rev. Resour. Econ. 14 , 333–354 (2021).
Zhang, L. et al. Biological invasions facilitate zoonotic disease emergences. Nat. Commun. 13 , 1762 (2022).
Olival, K. J. et al. Host and viral traits predict zoonotic spillover from mammals. Nature 546 , 646–650 (2017).
Guth, S. et al. Bats host the most virulent—but not the most dangerous—zoonotic viruses. Proc. Natl Acad. Sci. USA 119 , e2113628119 (2022).
Nelson, G. C. et al. in Ecosystems and Human Well-Being (Millennium Ecosystem Assessment) Vol. 2 (eds Rola, A. et al) Ch. 7, 172–222 (Island Press, 2005).
Read, A. F., Graham, A. L. & Raberg, L. Animal defenses against infectious agents: is damage control more important than pathogen control? PLoS Biol. 6 , 2638–2641 (2008).
Article CAS Google Scholar
Medzhitov, R., Schneider, D. S. & Soares, M. P. Disease tolerance as a defense strategy. Science 335 , 936–941 (2012).
Torchin, M. E. & Mitchell, C. E. Parasites, pathogens, and invasions by plants and animals. Front. Ecol. Environ. 2 , 183–190 (2004).
Bellay, S., de Oliveira, E. F., Almeida-Neto, M. & Takemoto, R. M. Ectoparasites are more vulnerable to host extinction than co-occurring endoparasites: evidence from metazoan parasites of freshwater and marine fishes. Hydrobiologia 847 , 2873–2882 (2020).
Scheffer, M. Critical Transitions in Nature and Society Vol. 16 (Princeton Univ. Press, 2020).
Rohr, J. R. et al. A planetary health innovation for disease, food and water challenges in Africa. Nature 619 , 782–787 (2023).
Reaser, J. K., Witt, A., Tabor, G. M., Hudson, P. J. & Plowright, R. K. Ecological countermeasures for preventing zoonotic disease outbreaks: when ecological restoration is a human health imperative. Restor. Ecol. 29 , e13357 (2021).
Hopkins, S. R. et al. Evidence gaps and diversity among potential win–win solutions for conservation and human infectious disease control. Lancet Planet. Health 6 , e694–e705 (2022).
Mitchell, C. E. & Power, A. G. Release of invasive plants from fungal and viral pathogens. Nature 421 , 625–627 (2003).
Chamberlain, S. A. & Szöcs, E. taxize: taxonomic search and retrieval in R. F1000Research 2 , 191 (2013).
Newman, M. Fundamentals of Ecotoxicology (CRC Press/Taylor & Francis Group, 2010).
Rohatgi, A. WebPlotDigitizer v.4.5 (2021); automeris.io/WebPlotDigitizer .
Lüdecke, D. esc: effect size computation for meta analysis (version 0.5.1). Zenodo https://doi.org/10.5281/zenodo.1249218 (2019).
Lipsey, M. W. & Wilson, D. B. Practical Meta-Analysis (SAGE, 2001).
R Core Team. R: A Language and Environment for Statistical Computing Vol. 2022 (R Foundation for Statistical Computing, 2020); www.R-project.org/ .
Viechtbauer, W. Conducting meta-analyses in R with the metafor package. J. Stat. Softw. 36 , 1–48 (2010).
Pustejovsky, J. E. & Tipton, E. Meta-analysis with robust variance estimation: Expanding the range of working models. Prev. Sci. 23 , 425–438 (2022).
Lenth, R. emmeans: estimated marginal means, aka least-squares means. R package v.1.5.1 (2020).
Bartoń, K. MuMIn: multi-modal inference. Model selection and model averaging based on information criteria (AICc and alike) (2019).
Burnham, K. P. & Anderson, D. R. Multimodel inference: understanding AIC and BIC in model selection. Sociol. Methods Res. 33 , 261–304 (2004).
Article MathSciNet Google Scholar
Marks‐Anglin, A. & Chen, Y. A historical review of publication bias. Res. Synth. Methods 11 , 725–742 (2020).
Nakagawa, S. et al. Methods for testing publication bias in ecological and evolutionary meta‐analyses. Methods Ecol. Evol. 13 , 4–21 (2022).
Gurevitch, J., Koricheva, J., Nakagawa, S. & Stewart, G. Meta-analysis and the science of research synthesis. Nature 555 , 175–182 (2018).
Bates, D., Mächler, M., Bolker, B. & Walker, S. Fitting linear mixed-effects models using lme4. J. Stat. Softw. 67 , 1–48 (2015).
Mahon, M. B. et al. Data and code for ‘A meta-analysis on global change drivers and the risk of infectious disease’. Zenodo https://doi.org/10.5281/zenodo.8169979 (2024).
Mahon, M. B. et al. Data and code for ‘A meta-analysis on global change drivers and the risk of infectious disease’. GitHub github.com/mahonmb/GCDofDisease (2024).
Download references
Acknowledgements
We thank C. Mitchell for contributing data on enemy release; L. Albert and B. Shayhorn for assisting with data collection; J. Gurevitch, M. Lajeunesse and G. Stewart for providing comments on an earlier version of this manuscript; and C. Carlson and two anonymous reviewers for improving this paper. This research was supported by grants from the National Science Foundation (DEB-2109293, DEB-2017785, DEB-1518681, IOS-1754868), National Institutes of Health (R01TW010286) and US Department of Agriculture (2021-38420-34065) to J.R.R.; a US Geological Survey Powell grant to J.R.R. and S.L.R.; University of Connecticut Start-up funds to S.A.K.; grants from the National Science Foundation (IOS-1755002) and National Institutes of Health (R01 AI150774) to D.J.C.; and an Ambizione grant (PZ00P3_202027) from the Swiss National Science Foundation to F.W.H. The funders had no role in study design, data collection and analysis, decision to publish or preparation of the manuscript.
Author information
These authors contributed equally: Michael B. Mahon, Alexandra Sack, Jason R. Rohr
Authors and Affiliations
Department of Biological Sciences, University of Notre Dame, Notre Dame, IN, USA
Michael B. Mahon, Alexandra Sack, O. Alejandro Aleuy, Carly Barbera, Ethan Brown, Heather Buelow, Luz A. de Wit, Meghan Forstchen, Patrick Heffernan, Alexis Korotasz, Joanna G. Larson, Samantha L. Rumschlag, Emily Selland, Alexander Shepack, Nitin Vincent & Jason R. Rohr
Environmental Change Initiative, University of Notre Dame, Notre Dame, IN, USA
Michael B. Mahon, Samantha L. Rumschlag & Jason R. Rohr
Eck Institute of Global Health, University of Notre Dame, Notre Dame, IN, USA
Alexandra Sack, Meghan Forstchen, Emily Selland & Jason R. Rohr
Department of Biology, Emory University, Atlanta, GA, USA
David J. Civitello
Department of Ecology and Evolutionary Biology, Yale University, New Haven, CT, USA
Jeremy M. Cohen
Department of Botany and Plant Pathology, Oregon State University, Corvallis, OR, USA
Fletcher W. Halliday
Department of Ecology and Evolutionary Biology, Institute for Systems Genomics, University of Connecticut, Storrs, CT, USA
Sarah A. Knutie
You can also search for this author in PubMed Google Scholar
Contributions
J.R.R. conceptualized the study. All of the authors contributed to the methodology. All of the authors contributed to investigation. Visualization was performed by M.B.M. The initial study list and related information were compiled by D.J.C., J.M.C., F.W.H., S.A.K., S.L.R. and J.R.R. Data extraction was performed by M.B.M., A.S., O.A.A., C.B., E.B., H.B., L.A.d.W., M.F., P.H., A.K., J.G.L., E.S., A.S. and N.V. Data were checked for accuracy by M.B.M. and A.S. Analyses were performed by M.B.M. and J.R.R. Funding was acquired by D.J.C., J.R.R., S.A.K. and S.L.R. Project administration was done by J.R.R. J.R.R. supervised the study. J.R.R. and M.B.M. wrote the original draft. All of the authors reviewed and edited the manuscript. J.R.R. and M.B.M. responded to reviewers.
Corresponding author
Correspondence to Jason R. Rohr .
Ethics declarations
Competing interests.
The authors declare no competing interests.
Peer review
Peer review information.
Nature thanks Colin Carlson and the other, anonymous, reviewer(s) for their contribution to the peer review of this work. Peer reviewer reports are available.
Additional information
Publisher’s note Springer Nature remains neutral with regard to jurisdictional claims in published maps and institutional affiliations.

Extended data figures and tables
Extended data fig. 1 prisma flowchart..
The PRISMA flow diagram of the search and selection of studies included in this meta-analysis. Note that 77 studies came from the Halliday et al. 3 database on biodiversity change.
Extended Data Fig. 2 Summary of the number of studies (A-F) and parasite taxa (G-L) in the infectious disease database across ecological contexts.
The contexts are global change driver ( A , G ), parasite taxa ( B , H ), host taxa ( C , I ), experimental venue ( D , J ), study habitat ( E , K ), and human parasite status ( F , L ).
Extended Data Fig. 3 Summary of the number of effect sizes (A-I), studies (J-R), and parasite taxa (S-a) in the infectious disease database for various parasite and host contexts.
Shown are parasite type ( A , J , S ), host thermy ( B , K , T ), vector status ( C , L , U ), vector-borne status ( D , M , V ), parasite transmission ( E , N , W ), free living stages ( F , O , X ), host (e.g. disease, host growth, host survival) or parasite (e.g. parasite abundance, prevalence, fecundity) endpoint ( G , P , Y ), micro- vs macroparasite ( H , Q , Z ), and zoonotic status ( I , R , a ).
Extended Data Fig. 4 The effects of global change drivers and subsequent subcategories on disease responses with Log Response Ratio instead of Hedge’s g.
Here, Log Response Ratio shows similar trends to that of Hedge’s g presented in the main text. The displayed points represent the mean predicted values (with 95% confidence intervals) from a meta-analytical model with separate random intercepts for study. Points that do not share letters are significantly different from one another (p < 0.05) based on a two-sided Tukey’s posthoc multiple comparison test with adjustment for multiple comparisons. See Table S 3 for pairwise comparison results. Effects of the five common global change drivers ( A ) have the same directionality, similar magnitude, and significance as those presented in Fig. 2 . Global change driver effects are significant when confidence intervals do not overlap with zero and explicitly tested with two-tailed t-test (indicated by asterisks; t 80.62 = 2.16, p = 0.034 for CP; t 71.42 = 2.10, p = 0.039 for CC; t 131.79 = −3.52, p < 0.001 for HLC; t 61.9 = 2.10, p = 0.040 for IS). The subcategories ( B ) also show similar patterns as those presented in Fig. 3 . Subcategories are significant when confidence intervals do not overlap with zero and were explicitly tested with two-tailed one sample t-test (t 30.52 = 2.17, p = 0.038 for CO 2 ; t 40.03 = 4.64, p < 0.001 for Enemy Release; t 47.45 = 2.18, p = 0.034 for Mean Temperature; t 110.81 = −4.05, p < 0.001 for Urbanization); all other subcategories have p > 0.20. Note that effect size and study numbers are lower here than in Figs. 3 and 4 , because log response ratios cannot be calculated for studies that provide coefficients (e.g., odds ratio) rather than raw data; as such, all observations within BC did not have associated RR values. Despite strong differences in sample size, patterns are consistent across effect sizes, and therefore, we can be confident that the results presented in the main text are not biased because of effect size selection.
Extended Data Fig. 5 Average standard errors of the effect sizes (A) and sample sizes per effect size (B) for each of the five global change drivers.
The displayed points represent the mean predicted values (with 95% confidence intervals) from the generalized linear mixed effects models with separate random intercepts for study (Gaussian distribution for standard error model, A ; Poisson distribution for sample size model, B ). Points that do not share letters are significantly different from one another (p < 0.05) based on a two-sided Tukey’s posthoc multiple comparison test with adjustment for multiple comparisons. Sample sizes (number of studies, n, and effect sizes, k) for each driver are as follows: n = 77, k = 392 for BC; n = 124, k = 364 for CP; n = 202, k = 380 for CC; n = 517, k = 1449 for HLC; n = 96, k = 355 for IS.
Extended Data Fig. 6 Forest plots of effect sizes, associated variances, and relative weights (A), Funnel plots (B), and Egger’s Test plots (C) for each of the five global change drivers and leave-one-out publication bias analyses (D).
In panel A , points are the individual effect sizes (Hedge’s G), error bars are standard errors of the effect size, and size of the points is the relative weight of the observation in the model, with larger points representing observations with higher weight in the model. Sample sizes are provided for each effect size in the meta-analytic database. Effect sizes were plotted in a random order. Egger’s tests indicated significant asymmetries (p < 0.05) in Biodiversity Change (worst asymmetry – likely not bias, just real effect of positive relationship between diversity and disease), Climate Change – (weak asymmetry, again likely not bias, climate change generally increases disease), and Introduced Species (relatively weak asymmetry – unclear whether this is a bias, may be driven by some outliers). No significant asymmetries (p > 0.05) were found in Chemical Pollution and Habitat Loss/Change, suggesting negligible publication bias in reported disease responses across these global change drivers ( B , C ). Egger’s test included publication year as moderator but found no significant relationship between Hedge’s g and publication year (p > 0.05) implying no temporal bias in effect size magnitude or direction. In panel D , the horizontal red lines denote the grand mean and SE of Hedge’s g and (g = 0.1009, SE = 0.0338). Grey points and error bars indicate the Hedge’s g and SEs, respectively, using the leave-one-out method (grand mean is recalculated after a given study is removed from dataset). While the removal of certain studies resulted in values that differed from the grand mean, all estimated Hedge’s g values fell well within the standard error of the grand mean. This sensitivity analysis indicates that our results were robust to the iterative exclusion of individual studies.
Extended Data Fig. 7 The effects of habitat loss/change on disease depend on parasite taxa and land use conversion contexts.
A) Enemy type influences the magnitude of the effect of urbanization on disease: helminths, protists, and arthropods were all negatively associated with urbanization, whereas viruses were non-significantly positively associated with urbanization. B) Reference (control) land use type influences the magnitude of the effect of urbanization on disease: disease was reduced in urban settings compared to rural and peri-urban settings, whereas there were no differences in disease along urbanization gradients or between urban and natural settings. C) The effect of forest fragmentation depends on whether a large/continuous habitat patch is compared to a small patch or whether disease it is measured along an increasing fragmentation gradient (Z = −2.828, p = 0.005). Conversely, the effect of deforestation on disease does not depend on whether the habitat has been destroyed and allowed to regrow (e.g., clearcutting, second growth forests, etc.) or whether it has been replaced with agriculture (e.g., row crop, agroforestry, livestock grazing; Z = 1.809, p = 0.0705). The displayed points represent the mean predicted values (with 95% confidence intervals) from a metafor model where the response variable was a Hedge’s g (representing the effect on an infectious disease endpoint relative to control), study was treated as a random effect, and the independent variables included enemy type (A), reference land use type (B), or land use conversion type (C). Data for (A) and (B) were only those studies that were within the “urbanization” subcategory; data for (C) were only those studies that were within the “deforestation” and “forest fragmentation” subcategories. Sample sizes (number of studies, n, and effect sizes, k) in (A) for each enemy are n = 48, k = 98 for Virus; n = 193, k = 343 for Protist; n = 159, k = 490 for Helminth; n = 10, k = 24 for Fungi; n = 103, k = 223 for Bacteria; and n = 30, k = 73 for Arthropod. Sample sizes in (B) for each reference land use type are n = 391, k = 1073 for Rural; n = 29, k = 74 for Peri-urban; n = 33, k = 83 for Natural; and n = 24, k = 58 for Urban Gradient. Sample sizes in (C) for each land use conversion type are n = 7, k = 47 for Continuous Gradient; n = 16, k = 44 for High/Low Fragmentation; n = 11, k = 27 for Clearcut/Regrowth; and n = 21, k = 43 for Agriculture.
Extended Data Fig. 8 The effects of common global change drivers on mean infectious disease responses in the literature depends on whether the endpoint is the host or parasite; whether the parasite is a vector, is vector-borne, has a complex or direct life cycle, or is a macroparasite; whether the host is an ectotherm or endotherm; or the venue and habitat in which the study was conducted.
A ) Parasite endpoints. B ) Vector-borne status. C ) Parasite transmission route. D ) Parasite size. E ) Venue. F ) Habitat. G ) Host thermy. H ) Parasite type (ecto- or endoparasite). See Table S 2 for number of studies and effect sizes across ecological contexts and global change drivers. See Table S 3 for pairwise comparison results. The displayed points represent the mean predicted values (with 95% confidence intervals) from a metafor model where the response variable was a Hedge’s g (representing the effect on an infectious disease endpoint relative to control), study was treated as a random effect, and the independent variables included the main effects and an interaction between global change driver and the focal independent variable (whether the endpoint measured was a host or parasite, whether the parasite is vector-borne, has a complex or direct life cycle, is a macroparasite, whether the study was conducted in the field or lab, habitat, the host is ectothermic, or the parasite is an ectoparasite).
Extended Data Fig. 9 The effects of five common global change drivers on mean infectious disease responses in the literature only occasionally depend on location, host taxon, and parasite taxon.
A ) Continent in which the field study occurred. Lack of replication in chemical pollution precluded us from including South America, Australia, and Africa in this analysis. B ) Host taxa. C ) Enemy taxa. See Table S 2 for number of studies and effect sizes across ecological contexts and global change drivers. See Table S 3 for pairwise comparison results. The displayed points represent the mean predicted values (with 95% confidence intervals) from a metafor model where the response variable was a Hedge’s g (representing the effect on an infectious disease endpoint relative to control), study was treated as a random effect, and the independent variables included the main effects and an interaction between global change driver and continent, host taxon, and enemy taxon.
Extended Data Fig. 10 The effects of human vs. non-human endpoints for the zoonotic disease subset of database and wild vs. domesticated animal endpoints for the non-human animal subset of database are consistent across global change drivers.
(A) Zoonotic disease responses measured on human hosts responded less positively (closer to zero when positive, further from zero when negative) than those measured on non-human (animal) hosts (Z = 2.306, p = 0.021). Note, IS studies were removed because of missing cells. (B) Disease responses measured on domestic animal hosts responded less positively (closer to zero when positive, further from zero when negative) than those measured on wild animal hosts (Z = 2.636, p = 0.008). These results were consistent across global change drivers (i.e., no significant interaction between endpoint and global change driver). As many of the global change drivers increase zoonotic parasites in non-human animals and all parasites in wild animals, this may suggest that anthropogenic change might increase the occurrence of parasite spillover from animals to humans and thus also pandemic risk. The displayed points represent the mean predicted values (with 95% confidence intervals) from a metafor model where the response variable was a Hedge’s g (representing the effect on an infectious disease endpoint relative to control), study was treated as a random effect, and the independent variable of global change driver and human/non-human hosts. Data for (A) were only those diseases that are considered “zoonotic”; data for (B) were only those endpoints that were measured on non-human animals. Sample sizes in (A) for zoonotic disease measured on human endpoints across global change drivers are n = 3, k = 17 for BC; n = 2, k = 6 for CP; n = 25, k = 39 for CC; and n = 175, k = 331 for HLC. Sample sizes in (A) for zoonotic disease measured on non-human endpoints across global change drivers are n = 25, k = 52 for BC; n = 2, k = 3 for CP; n = 18, k = 29 for CC; n = 126, k = 289 for HLC. Sample sizes in (B) for wild animal endpoints across global change drivers are n = 28, k = 69 for BC; n = 21, k = 44 for CP; n = 50, k = 89 for CC; n = 121, k = 360 for HLC; and n = 29, k = 45 for IS. Sample sizes in (B) for domesticated animal endpoints across global change drivers are n = 2, k = 4 for BC; n = 4, k = 11 for CP; n = 7, k = 20 for CC; n = 78, k = 197 for HLC; and n = 1, k = 2 for IS.
Supplementary information
Supplementary information.
Supplementary Discussion, Supplementary References and Supplementary Tables 1–3.
Reporting Summary
Peer review file, supplementary data 1.
R markdown code and output associated with this paper.
Supplementary Table 4
EcoEvo PRISMA checklist.
Rights and permissions
Springer Nature or its licensor (e.g. a society or other partner) holds exclusive rights to this article under a publishing agreement with the author(s) or other rightsholder(s); author self-archiving of the accepted manuscript version of this article is solely governed by the terms of such publishing agreement and applicable law.
Reprints and permissions
About this article
Cite this article.
Mahon, M.B., Sack, A., Aleuy, O.A. et al. A meta-analysis on global change drivers and the risk of infectious disease. Nature 629 , 830–836 (2024). https://doi.org/10.1038/s41586-024-07380-6
Download citation
Received : 02 August 2022
Accepted : 03 April 2024
Published : 08 May 2024
Issue Date : 23 May 2024
DOI : https://doi.org/10.1038/s41586-024-07380-6
Share this article
Anyone you share the following link with will be able to read this content:
Sorry, a shareable link is not currently available for this article.
Provided by the Springer Nature SharedIt content-sharing initiative
By submitting a comment you agree to abide by our Terms and Community Guidelines . If you find something abusive or that does not comply with our terms or guidelines please flag it as inappropriate.
Quick links
- Explore articles by subject
- Guide to authors
- Editorial policies
Sign up for the Nature Briefing: Anthropocene newsletter — what matters in anthropocene research, free to your inbox weekly.

Uncovering the impact of cultural heritage on economic growth: empirical evidence from Greek regions, 2000–2019
- Original Paper
- Open access
- Published: 16 May 2024
Cite this article
You have full access to this open access article
- Ioannis Kostakis ORCID: orcid.org/0000-0002-3507-5737 1 &
- Sarantis Lolos 2
193 Accesses
Explore all metrics
This paper brings empirical evidence on the role of cultural heritage assets in promoting economic growth. The case of Greece at regional level over the period 2000–2019 is taken as an example, owing to important cultural heritage endowment. Economic growth is approached by formulating a neoclassical growth model augmented with a dynamic cultural heritage index. The relationship between economic growth and cultural heritage is examined through a heterogeneous and cross-correlated panel data analysis. The empirical results reveal a positive impact of cultural heritage endowment on economic growth. Also, a significant positive influence of physical capital and a negative impact of unemployment on the economy are evidenced. The conclusions drawn could be useful to regions and countries to upgrade their cultural heritage endowment to accelerate economic growth.
Similar content being viewed by others
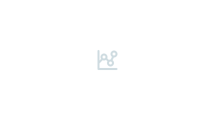
Causality Between Cultural Diversity and Economic Growth in India: Cross-State Study, 1990–1991 through 2017–2018
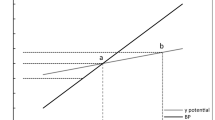
How do inequalities in cultural engagement impact on economic growth?
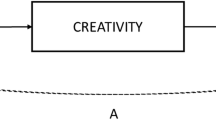
A new perspective on the cultural heritage–development nexus: the role of creativity
Avoid common mistakes on your manuscript.
1 Introduction
Cultural heritage is of outmost importance, affecting various aspects of people's lives. The European cultural heritage is a primary resource for democratic engagement supporting cultural diversity and sustainable development. It represents cultural capital from which future cultural, social and economic development may be generated (Faro Convention 2005 ). Footnote 1 In the European context, cultural heritage has been recognised as an advantage and an engine of growth. The European Union focuses on cultural positive contribution to Europe's societies and economies and is committed to promoting, restoring, and conserving cultural heritage. The role of cultural heritage became crucial in achieving innovative, sustainable and inclusive growth in the “Europe 2020” strategy (European Commission 2010 ), while in “Getting cultural heritage to work for Europe” (European Commission 2015 ), cultural heritage is considered as a production factor and an important resource for innovation, social inclusion and sustainability. It is also considered a means of improving economic performance, people's lives and living environments. Also, in the “New European agenda for culture” (European Commission 2018 ) it was decided to strengthen the European Union's cultural dimension further.
The role of cultural heritage in economic development has gained more and more interest in recent years. Many researchers realize the positive contribution of culture and cultural heritage to economic growth and argue that cultural variables can increase the explanatory power of the classic econometric model. They propose that cultural variables must be treated as important causal variables and they incorporate cultural factors, such as cultural capital, into formal behavioural models of economic growth and development (Throsby 1999 ; Granato et al. 1996 ; Altman 2001 ). Many studies evaluate the economic impact of cultural heritage on economic growth but formal empirical analysis is limited. Most econometric studies investigate the impact of cultural heritage on growth directly or through its relation to tourism demand. In these cases, cultural heritage assets are usually proxied by “the number of UNESCO's World Heritage Sites (WHS)” situated in a country/region, where WHS are treated as a time-invariant structural variable (Faria and León-Ledesma 2008 ). Cultural heritage assets started to be considered as elements of territorial attractiveness, differentiation, competitiveness, and cultural heritage and tourism began to be considered inextricably linked (OECD 2008 ).
This paper aims to empirically bring out the role of cultural heritage endowment as a driver for economic growth. The impact of cultural heritage endowment on the economy is investigated via an empirical neoclassical growth model (Solow 1956 ) augmented with a cultural heritage variable (Throsby 1999 ). In our model, the impact of cultural heritage on economic growth takes place through the tourism demand channel. Thus, promoting cultural heritage assets makes them more attractive to visitors, giving rise to a greater demand for cultural tourism which generates more income and employment (Richards 2002 , 2007 ). At the same time the process of promoting cultural heritage assets improves cultural and social capital which further boosts economic development and growth (Beugelsdijk and van Schaik 2005 ; Malecki 2012 ). Thus, cultural heritage assets create incomes, employment, revenues and wealth. The case of Greece (2000–2019) serves as an example for our empirical investigation. Cultural heritage has been recognized in the EU as a European advantage and an engine of growth, making this investigation more valuable. Our paper brings fresh ideas in many respects.
The first novelty is the choice of a cultural heritage variable incorporated in the empirical model that depicts the impact of cultural heritage on the economy. We define a cultural heritage variable, the cultural heritage (CH) index, which is a proxy of the relative importance of cultural tourism with respect to total tourism volume. The CH index is calculated as the ratio of visitors to historical monuments to the total number of tourists in a region. The number of visitors to historical monuments indicates the attractiveness and market value of cultural heritage assets, which may be due to the development of the number of open to public monuments (supply side effect) and/or the number of cultural visitors (demand side effect). Furthermore, the CH index is a dynamic measure that accounts for developing cultural heritage market value over time. Hence, the CH index captures cultural heritage's economic impact more efficiently than using a time-invariant proxy such as “the number of UNESCO's World Heritage Sites” used in most relevant empirical studies.
The second point of interest is that we investigate the impact of cultural heritage assets on the economy in broad terms, since we include in the analysis a significant number of important cultural heritage monuments and not only those of UNESCO's list, which again have been the focus of the majority of relevant empirical studies. To this end we have chosen to investigate the case of Greece, a country with an endowment of 156 open-to-public archaeological sites and 175 museums in 2019, including monuments of UNESCO's list, showing a strong regional allocation. Footnote 2
Third, we explore the impact of cultural heritage on economic growth at regional level using panel estimators that account for cross-region heterogeneity and cross-sectional dependence. By doing so, we can consider various sources of heterogeneity among regions. Moreover, we examine the effect of cultural heritage on economic growth across conditional distribution, which is vital for policymaking as it provides insights into the effects at the extremes of the distribution. Neglecting distributional heterogeneity in the analysis may lead to erroneous results and inappropriate policy recommendations. Also, we employ a novel bootstrapping panel Granger non-causality approach that reduces cross-sectional dependence. This approach yields more detailed information on causal relationships, leading to more accurate policy implications.
The rest of the paper is organised as follows. Section 2 is the literature review. Section 3 briefly presents features of the Greek economy. Section 4 discusses the methodology, data and variables used in the empirical analysis. Section 5 presents the empirical results, and Sect. 6 summarizes the analysis results and concludes the issue.
2 Literature review
Several researchers have empirically investigated the relationship between cultural heritage and economic growth. Most empirical studies use “the number of UNESCO's World Heritage Sites (WHS)” situated in a country/region as a proxy to capture the impact of cultural heritage assets on the economy.
This variable was initially introduced by Faria and León-Ledesma ( 2008 ) as a proxy for cultural capital stock to assess its impact on economic growth. The rationale is that this variable depicts all aspects of the cultural contribution of each nation to humankind. The idea is that this proxy captures the intensity of culture, where a country with a large stock of cultural heritage has a comparative advantage over others with a smaller cultural heritage. It also reflects comparative cultural advantage in individual attitudes and social institutions that become or influence universal values, beliefs and norms. Their analysis of 87 countries showed that a country with a higher cultural heritage share grows faster, a finding strongly supporting the hypothesis that cultural heritage has a positive and significant impact on economic growth (Faria and León-Ledesma ( 2022 ). Similarly, Saccone and Bertacchini ( 2011 ), using UNESCO's World Heritage Sites for a panel of 131 countries over the period 1978–2007, found that economic size and participation in the World Heritage system are positively related; and the promotion and preservation actions of cultural heritage may create development opportunities.
Many empirical studies on the relationship between cultural heritage and economic growth have a strong local dimension; also, they provide a comprehensive understanding of the multifaceted role of cultural heritage in economic growth and development. They emphasize that regions and cities may build competitiveness by leveraging their cultural heritage (Scott 2000 ; Bandarin et al. 2011 ; Licciardi and Amirtahmasebi 2012 ). Promoting localized cultural industries is considered necessary in generating opportunities for commercial initiatives, business expansion, and employment growth and providing increased incomes and widespread community benefits. Furthermore, developing the local economy through a higher cultural and social capital attracts creative people, making production more efficient via higher technology, innovation, learning and entrepreneurship (Beugelsdijk and van Schaik 2005 ; Beugelsdijk et al. 2006 ; Sasaki 2010 ; Malecki 2012 ). The development of cultural heritage and cultural environments also attract specific skilled individuals from other regions (Backman and Nilsson 2018 ). Furthermore, cultural heritage embodies the character of a specific area, thus highlighting the effort of local policymakers and territorial institutions to develop programs and policies in order to promote and preserve this heritage (Rodríguez-Pose 2013 , 2020 ).
Several empirical studies support that tourism positively affects economic development, since a country/region with a significant cultural heritage stock has an advantage over others with a smaller endowment. When a transmission channel is considered, it is usually related to tourism, since cultural heritage and tourism are considered inextricably linked (OECD 2008 ).
Yang et al. ( 2010 ) empirically address the impact of cultural heritage on tourism flows in China, thus implying an indirect impact of cultural heritage assets on economic development. The number of UNESCO's World Heritage Sites is used as a proxy depicting the economic impact of cultural heritage. Their results show that this variable significantly explains the number of international tourism arrivals. Also, Arezki et al. ( 2012 ) investigate whether tourism specialization is a viable strategy for development by estimating standard growth equations for a cross-section of 127 countries from 1980 to 2002. Their models are augmented with an instrument measuring tourism specialization; the number of UNESCO's World Heritage Sites per 100,000 inhabitants in 2002. Their results show that the presence of UNESCO's sites significantly fosters tourism activities.
Cuccia et al . ( 2016 ) explore the effects of cultural heritage in fostering tourism demand. They use a DEA model to investigate the role of UNESCO's World Heritage Sites in boosting tourism destinations' competitiveness. The empirical analysis is carried out for 21 Italian regions and autonomous provinces from 1995 to 2010. The results show that cultural and environmental endowment positively affects the performance of Italian tourism destinations; also the presence of UNESCO's Sites exerts opposite effects. Bacsi and Tóth ( 2019 ) investigate the relationship between the presence of unique World Heritage Sites for tourist attractions and international tourism performance. Their empirical analysis for 129 countries over the period 2014–2017 shows that cultural and natural world heritage sites are generally strong tourist attractions and can contribute to increased tourism. Škrabic et al. ( 2021 ) estimate the impact of various cultural indicators on tourism development in 27 EU member states over the period 2008–2018, using dynamic panel data analysis. Their results indicate that the number of UNESCO sites significantly positively affects international tourism receipts and employment.
Kostakis et al. ( 2020 ) empirically investigate the relationship between cultural heritage assets and economic growth at regional level in the case of Greece (1998–2016). They employ a neoclassical growth model, and the analysis is based on GMM dynamic panel data. The empirical results reveal a positive impact of cultural heritage assets demand -reflected in the number of monument visitors- on growth. The results also show an influence of other growth factors, such as physical and human capital, fertility and unemployment on growth. Also, Doulgeraki ( 2018 ) investigated the macroeconomic impact of cultural heritage -in terms of the number of visitors to monuments- and tourist demand -in terms of tourism arrivals- on economic growth in Greece over the period 1970–2015. She found a strong impact of cultural heritage and tourism demand on economic growth.
Panzera et al. ( 2021 ), empirically investigate the impact of tangible cultural heritage on the tourism attractiveness of European regions using a Bayesian multilevel gravity model. Their study covers locally and nationally defined monuments, museums, and several monuments on UNESCO's list. They find that UNESCO's sites are far more attractive than regional or national monuments that play a more limited role in international tourism. Panzera ( 2023 ) investigates the impact of cultural heritage and tourism attractiveness on local economic development. The empirical analysis for 269 European regions in the mid-2010s was carried out by estimating a structural equation model with cultural heritage, tourism attractiveness and economic development. Her results show that cultural heritage attracts tourists and, in turn, this increased tourism demand positively affects local economies.
Besides overall tourism, cultural tourism is closely related to cultural heritage offering a specific identity to tourist destinations and visitors. Cultural tourism is a kind of tourism activity where the visitors' essential motivation is to learn, discover, experience, taste and enjoy the tangible and intangible cultural attractions/products in a tourist destination (Richards 2018 ). It directly and positively affects economic growth, since a country or region with a significant cultural heritage stock has an advantage over others with a smaller endowment (Sasaki 2010 ; Licciardi and Amirtahmasebi 2012 ). Cultural tourism has been recognized as one of the most crucial transmission channels of cultural heritage endowment on economic performance (Richards 2002 , 2007 ; Lee and Chhabra 2015 ). It brings new and fresh resources to the cultural sector, spotting new entrepreneurial opportunities by boosting economic activity, incomes and employment (Hampton 2005 ; Alberti and Guisti 2012 ; UNWTO 2018 ). However, cultural tourism is not the only transmission channel that affects economic development. Ashworth ( 2013 ) presents several channels through which cultural heritage could boost economic development. As a commercial activity, as a location factor for other economic activities, as a contributor to environmental amenities and local identity, as a factor of place image promotion and branding (Pasquinelli et al. 2023 ) and as an element of neighbourhood regeneration. A recent study by Cerisola ( 2019 ) shows that cultural heritage in Italy indirectly affects regional development through artistic and scientific creativity.
A number of studies raise the sustainability issue of the cultural heritage-tourism relationship. In particular, Loulanski and Loulanski ( 2011 ) reviewed 483 studies and provided an interpretive synthesis of 15 critical factors for the sustainable integration of heritage and tourism. They show that tourism raises the economic and cultural value of heritage, but an increased economic value cannot compensate for losing other values in the longer term. They conclude that cultural heritage should be viewed as an irreplaceable form of capital (cultural, social, environmental and economic) wisely used, preserved, sustained and enhanced instead of being irretrievably consumed by tourism. Also, Nocca ( 2017 ) investigates cultural heritage's role in sustainable development by analysing the case of 40 cultural heritage conservation/regeneration projects, proposing a set of nine categories of multidimensional indicators. It is shown that in most cases, only the economic component is highlighted, leaving out the social and environmental dimensions. Furthermore, Romão and Neuts ( 2017 ) investigate the impact of tourism specialization to regional development, accounting for natural and cultural features, innovation capabilities and specialization patterns, for 252 European regions. They find the coexistence of different regional patterns of tourism dynamics leading to important spatial unbalances.
On the other hand, Dümcke and Gnedovsky ( 2013 ) provide evidence from several empirical studies, showing that generating knowledge and developing educational and innovative products is an indispensable feature of the heritage sector (smart growth). In addition, cultural heritage has a great potential for skills development and the generation of direct and indirect jobs, thus fostering social cohesion (inclusive growth). Finally, they support that cultural heritage contributes to sustainable growth by merging modernity and tradition and raises the profile of places, making them more competitive. Finally, micro-approaches assess the economic impact of cultural heritage on the local economy (e.g. Bowitz and Ibenholt 2009 ) or the impact of specific cultural events (e.g. Srakar and Vecco 2017 ).
3 Features of the Greek economy
In the period under review (2000–2019), the Greek economy followed two different paths (Fig. 1 ). Over most of the 2000s, the economy achieved high average growth rates of around 4% per annum, well above those of the EU countries. However, after 2007 there was a rapid deterioration of the Greek economy's internal and external balances, which almost coincided with the beginning of the global financial crisis (2008). In 2009, the external imbalance and the public sector deficit rocketed to over 15% of GDP, while the public debt to GDP ratio increased to 127% from around 100% in the first half of the 2000s (OECD 2009 ).
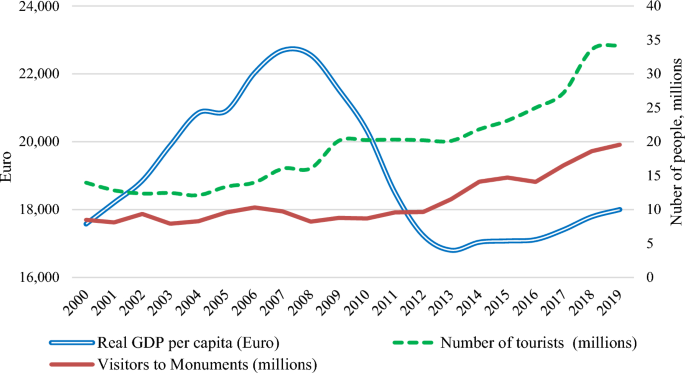
Real GDP per capita (left axis); number of tourists and visitors to monuments (right axis), 2000–2019. Sources : Eurostat and ELSTAT (Hellenic Statistical Authority)
In 2010, the government, to avoid default, was obliged to enforce a severe structural adjustment programme which would turn into a series of adjustment programmes (2010, 2012, 2015) mainly financed by the EU countries (European Commission 2018a ; IMF 2019 ). Various governments that came to office were thus obliged to implement severe austerity measures for fiscal consolidation, the achievement of external balance and competitiveness improvement, including tax increases and cuts in wages, pensions and salaries. As a result, the economic activity underwent an unprecedented depression, losing around 25% of its output (2010–2017) (Fig. 1 ), while the rate of unemployment tripled to 28% relatively to previous periods. After 2017/8 the economy started recovering with annual rates of 2% by 2019, while the unemployment rate dropped gradually to 17% (OECD 2023 ).
Tourism has always been a priority sector in Greece related to the country’s exceptional natural features, history and cultural heritage which are considered to be the catalyst in tourism development. Over the period 2000–2019 the number of international tourists more than doubled, from 13.8 to 34.2 million people (Fig. 1 ). In particular after the Athens Olympics (2004) and in conjunction with the adverse conditions in the tourist markets in the Eastern Mediterranean in the early 2010s, Footnote 3 tourism flows increased sharply. The industry enjoys easy finance and in recent years a more even expansion of tourism across regions is observed. However, the nature of Greek tourism greatly relies on (“sun and sea”) mass tourism showing a strong seasonality. In the post Greek economic crisis period (2010), positive tourist developments were facilitated by tourist market reforms giving a boost to the struggling domestic demand (Bank of Greece 2019 ).
Owing to its long-standing history, a distinct feature of Greece is its significant cultural heritage endowment, such as historical monuments, archaeological sites and museums. The country's rich historical legacy is reflected in UNESCO's World Heritage List. Footnote 4 Over the period 2000–2019, the number of visitors to archaeological sites and museums more than doubled from 8.5 to 19.6 million people (Fig. 1 ). Footnote 5 This ascending trend, especially over the second half of the period, reflects an expansion in cultural tourism demand and is also due to an increased number of museums and publicly accessible archaeological sites, including the launching of the flagship Acropolis Museum (2009). Note that the number of accessible public monuments has doubled over the period under examination (2000: 168, 2004: 183, 2010: 254, 2015: 274, 2019: 332). Footnote 6
The historical sites and museums are scattered across regions. However, the regional distribution of visitors is not uniform, depending on the characteristics of each monument, such as its historical importance, the convenience of visiting it, the attractiveness of the specific region, etc. About half of the regions, mainly those where important UNESCΟ monuments are situated, attract most visitors to historical sites and museums. Footnote 7 In particular, from 2000 to 2019 Attica attracted about 35% of total visitors to monuments in Greece and another three highly touristic regions (South Aegean, Crete and Peloponnesus) attracted around 15% of total visitors each. These top three regions are highly appreciated by about 80% of total visitors to monuments. They are followed by three regions (Central Macedonia, Central and Western Greece) with about 5% visitors each, while the attractiveness of the remaining six regions (Eastern Macedonia-Thrace, Western Macedonia, Epirus, Ionian Islands, Thessaly and Northern Aegean) is lower but not negligible.
Regarding the value of Cultural Heritage index, defined as the ratio of the total number of visitors to monuments to the total number of tourists in Greece, it amounts to around 50% on average over the period 2000–2019. Therefore, besides the richness of historical monuments in Greece, it seems that, on average, less than half of the tourists visit historical monuments, since many tourists visit more than one monument.
The regional distribution of the cultural heritage index in Greece is shown in Fig. 2 . Note that in four regions (Attica, Peloponnesus, Western and Central Greece) the index is well above the country's average (80–120%) and in another two regions (Southern Aegean, Crete) is close (60%) to the average. However, in the remaining seven regions the cultural heritage index is relatively low (15%), which means that the number of monument visitors in those regions can be increased substantially. Note that extensive overcrowding (“over-tourism”) in regions, where world heritage sites are situated has not been so far noticed.
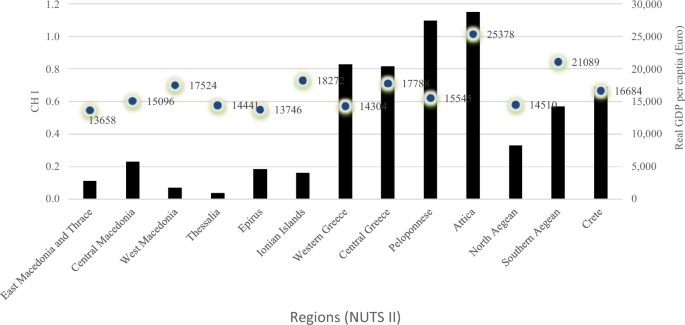
Cultural Heritage index (columns-left axis) and real GDP per capita (dots-right axis), average 2000–2019. Sources : Eurostat and ELSTAT (Hellenic Statistical Authority)
The relationship of real GDP per capita of the Greek regions and the cultural heritage index for the period 2000–2019 is shown in Fig. 3 , where a positive correlation between the two variables is observed. Also, it is shown an uneven regional distribution, since in six regions the per capita income is around the country’s average (16,771€) and the cultural heritage index is above 0.80, while the remaining seven lagging behind regions have a cultural heritage index of less than 0.20.
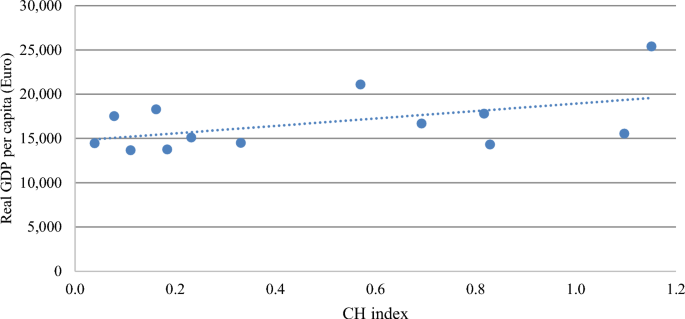
Cultural Heritage index and real GDP per capita, average 2000–2019. Sources : Eurostat and ELSTAT (Hellenic Statistical Authority)
4 The model, data and methodology
4.1 the model.
Economic theory and especially neoclassical models usually incorporate only strictly economic variables, such as capital and labour (e.g. Solow 1956 ). Following the discussion above, if the role of cultural heritage in economic performance is assumed away, there is a danger of a misleading solution (Gray 1996 ). Thus, an empirical model incorporating cultural and economic variables is superior to an explanation emphasizing one set of these variables. The point of departure of our empirical analysis is a modified neoclassical growth model that incorporates cultural factors as independent variables affecting economic growth. The empirical model is written as follows Footnote 8 :
where \(i = 1, \ldots ,13\) denotes regions, \(t = 2000, \ldots ,{ }2019\) denotes time, \(\beta_{i}\) s are the estimated coefficients and \(\varepsilon_{i,t}\) is the error term.
4.2 Data and variables
The dataset covers 13 Greek regions (NUTS II) over the period 2000–2019. Table 1 provides information on the variables used in the analysis. The data for constructing the variables is obtained from Eurostat and ELSTAT (Hellenic Statistical Authority).
The dependent variable \({\text{GDP}}_{i,t}\) represents the per capita real gross domestic product (in Euro). It is calculated as the ratio of real GDP (chain linked volume) to the average population of each year. The explanatory variables included in the empirical model are the following.
Physical Capital
\({\text{PhysCap}}_{i,t}\) indicates the per capita physical capital (in Euro). It is constructed by dividing gross fixed capital formation by the national deflator. According to the neoclassical model, gross fixed capital formation is an important growth factor that reflects investment in capital accumulation, technological innovation, and advancements that play a vital role in the growth process (Abreu 2019 ; Audretsch 2009 ; Acs and Audretsch 1988 ). At the same time, cross-sectional differences in economic performance can be associated with different investment rates (O'Mahony and de Boer 2002 ). In the case of Greece, several empirical studies have highlighted the role of physical capital on economic growth and found that physical capital exerts a strong positive impact on output (Fotopoulos and Spence 1998 ; Louri and Anagnostaki 1995 ; Liargovas and Repousis 2015 ).
Unemployment
\({\text{Unempl}}_{i,t }\) denotes the rate of unemployment for region i . It expresses the ratio of the total number of unemployed persons divided by the total labour force in the region. This variable is included in the model to capture an “Okun's law” type of growth-unemployment trade-off at regional level. An inverse impact of unemployment on economic growth is expected, highlighting that increased unemployment is related to lower output. Also, the unemployment variable in the relationship reflects the existence of an aggregate supply curve, and from an empirical perspective, the unemployment coefficient offers a tool for policymaking. Okun's Law ( 1962 ) is empirically verified at the regional level in Greece (Apergis and Rezitis 2003 ; Christopoulos 2004 ). Also, Lolos and Papapetrou ( 2012 ) empirically investigated the relationship between unemployment and real sector developments.
Cultural Heritage
The investigation of the impact of cultural heritage on economic activity is carried out through its relation to cultural tourism demand, on the grounds that cultural tourism is the most important transmission channel of cultural heritage endowment on economic performance (Richards 2002 , 2007 ; Lee and Chhabra 2015 ). Cultural heritage affects economic growth through cultural tourism demand, boosting job creation and attracting investment and businesses. Preserving and promoting cultural heritage assets can attract tourists and generate income through spending (accommodation, food, and souvenirs) and create jobs in construction, hospitality and cultural management. In addition, the development of cultural heritage necessitates the enhancement of cultural and social capital (Beugelsdijk and van Schaik 2005 ; Malecki 2012 ), by attracting specific skills (Backman and Nilsson 2018 ) such as multilingual guides, archaeologists, professional managers and computer experts and by technological upgrading production and entrepreneurship. At the same time it is created an environment for the efficient operation of institutions and the formulation of innovative policies in order to boost and preserve cultural heritage (Rodríguez-Pose 2013 ), providing regions with a sense of place and identity.
The impact of cultural heritage endowment on the economy at regional (NUTS II) level is shown in the cultural heritage index, denoted by \({\text{CH}}\;{\text{index}}_{i,t}\) . It is calculated as a ratio of the annual number of visits to archaeological sites and museums obtained from ELSTAT over total annual tourism arrivals taken from Eurostat. We use tourism arrivals to indicate the volume of tourism flow, as this variable is widely used in the relevant literature (Katircioglu 2009 ; Lolos et al. 2021 ). By the same token, we use the number of visits to archaeological sites and museums to reflect the market value of tangible cultural heritage assets. It captures the attractiveness of cultural heritage assets and gives an account of the volume of cultural tourism. Footnote 9
Thus, the CH index is a proxy of the relative importance of cultural heritage-led economic activity, i.e. cultural tourism, with respect to total tourism activity. It is a dynamic measure, incorporating demand and supply side effects of cultural heritage developments and is expected to affect income positively. Note that a positive impact of overall tourism on economic activity, the “tourism-led growth hypothesis” is empirically verified in the Greek case (Dritsakis 2004 ; Lolos et al. 2021 ).
Cultural heritage refers to cultural capital, both tangible and intangible, that has been inherited from previous generations. The tangible form includes museums, historical monuments, buildings, sites, cities or open public spaces, while intangible cultural phenomena include festivals, dances, rituals, traditional knowledge, etc. (Throsby 2012 ). Two different types of values can be derived from cultural heritage. The first is the market (use) value related to the revenue obtained from cultural heritage as a private good. Regarding monuments that tourists visit, the market value includes revenues from entrance tickets, economic benefits that tourists enjoy and revenues from the commercial exploitation of the monuments. The second is the non - market (non - use) value , related to the intangible religious, historical, social, aesthetic, emotional, or identity value accruing to those who experience the benefits of cultural heritage as a public good (Throsby 1999 ).
In our empirical analysis, only the tangible cultural heritage endowment is investigated. Intangible cultural heritage, such as handicrafts, gastronomy, festivals, concerts, oral traditions, and other cultural events, is considered to be as substantial. Still, its market value has not been accounted for due to lack of quantitative data.
4.3 Methodology
In this study, we employ panel cointegration analysis to investigate the association between gross domestic product, physical capital, unemployment rate, and cultural heritage index at a regional level. The eight-step analysis strategy regarding the econometric methodology is shown in Fig. 4 .
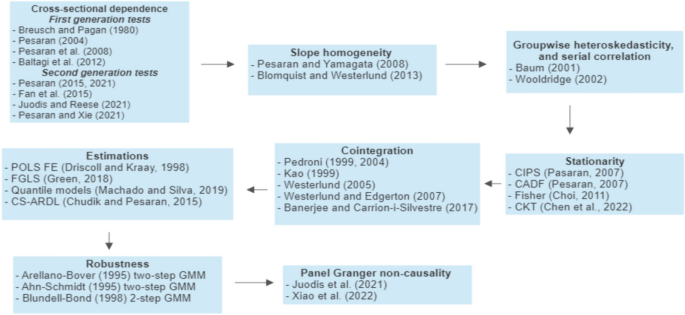
Econometric methodology flow chart
5 Empirical results
Table 2 presents the summary statistics (before log transformations) for the dependent and independent variables utilized in the analysis. Real GDP and physical capital are expressed at per capita levels. Unemployment is a ratio, and the cultural heritage index measures the total number of admissions to monuments over the total number of tourist arrivals in each region.
As can be seen, the average real GDP per capita is around 17,000 Euros. The average real gross fixed capital formation per capita is around 3000 Euros, exhibiting a core/periphery regional distribution pattern. The unemployment rate ranges from 4.7 to 31.6%, highlighting the heterogeneous regional profile of the country. The cultural heritage index's average value of around 0.50 is close to the Member States' World Trade Organization (2018) estimation. This result indicates that around 50% of tourists visit at least one museum or archaeological site.
We now turn to the econometric analysis. Firstly, we should note that regions of the same country are supposed to be highly interlinked, and the possibility of cross-sectional dependence (CSD) must be considered (Kapetanios et al. 2011 ). We may have biased stationarity and cointegration analysis estimates if cross-sectional dependence is not addressed. To this end, we employ first and second-generation cross-sectional dependence tests. The tests used are shown in Table 3 : the Pesaran ( 2004 ) CD 2004 ; the Pesaran et al., ( 2008 ) adjustedCD LM ; the Breusch-Pagan (1980) Lagrange Multiplier BP LM ; and the bias-corrected scaled LM (Baltagi et al. 2012 ) LM adj . In addition, we use second-generation cross-sectional dependence tests, such as the Pesaran ( 2015 ) CD 2015 test for weakly exogenous cross-section dependence in the large panel data econometrics combined with the Juodis-Reese ( 2022 ) CD w ; the Fan et al. ( 2015 ) CD w + ; and the Pesaran-Xie ( 2023 ) CD* test.
According to the results, the null hypotheses of cross-sectional independence (Panel A) and weak cross-sectional dependence (Panel B) are highly rejected. Hence, the empirical findings affirm the presence of CSD within the panel time-series data. This outcome signifies a level of interdependence among regions, suggesting that observations for distinct regions simultaneously may exhibit correlation or be influenced by common factors. Also, CSD implies that a positive or negative shock in one region can potentially affect another region.
After CSD, the slope homogeneity test checks whether heterogeneity among slope coefficients exists across regions. The slope homogeneity tests proposed by Pesaran and Yamagata ( 2008 ) and later updated by Blomquist and Westerlund ( 2013 ) are employed, following Bersvendsen and Ditzen ( 2020 ) approach, which allows for serial correlation, heteroscedasticity, and cross-sectional dependence, respectively. Under the null hypothesis, the slope coefficients are homogeneous across cross-sectional units. So, if the slope homogeneity hypothesis is rejected, model coefficients are heterogeneous, and estimators allowing for slope variation across regions should be employed. In addition, we check the possibility of groupwise heteroscedasticity (Wald statistic; Baum 2001 ) and cross-sectional correlation (Wooldridge test 2002 ) in the context of our series (Drukker 2003 ; Green 2000 ).
Table 4 provides the Delta ( \(\Delta\) ) and Delta-adjusted ( \(\Delta \_{\text{adj}}\) ) approaches for static and dynamic models. The results of the slope homogeneity tests, conducted at a 1% significance level, reject the null hypothesis, indicating the heterogeneity of the slope coefficients. Also, groupwise heteroscedasticity and serial correlation are present in our series. This implies that the variance of the error term varies across different cross-sectional units, while the error terms for a particular unit seem to be correlated across periods. To account for this issue we use feasible generalized least squares ( FGLS ) approach (Bai et al. 2021 ).
Subsequently, we analyse the stationarity of the series by utilizing several unit root tests. Stationarity is pivotal, denoting the constancy of statistical properties like mean and variance over time. Non-stationary data can yield spurious outcomes and erroneous inferences. Additionally, stationary series lend themselves to more straight forward modelling and analysis. Models constructed on stationary data yield more precise parameter estimates and offer more dependable forecasts. It contributes to comprehending the dynamics of each unit throughout the observed period, facilitating the identification of long-term trends and enabling meaningful cross-unit comparisons.
If there is cross-sectional dependence, the second-generation panel unit root tests, such as Cross-sectional Augmented Dickey-Fuller (CADF) and Cross-Sectional IPS (CIPS) unit root tests developed by Pesaran ( 2007 ), must be specified. Footnote 10 Under the null hypothesis, variables are unit root processes. We also perform two additional panel unit root tests as a robustness check. First, the Chen et al. ( 2022 ) panel unit root test that allows for possible structural breaks in the series, like the financial crisis, is utilized. If the series are not stationary in levels, they can be co-integrated and a long-term relationship can be estimated, regardless of the existence of structural breaks. In our case, a bootstrap procedure calculates the critical tests and p -values, assuming that errors are non-normal, cross-sectionally dependent, and cross-sectionally heteroscedastic. The null hypothesis indicates that all series in the panel are not stationary. The alternative is that some or all of the series are stationary, with breaks in the deterministic specification. Secondly, the Fisher-type (Choi 2001 ) PP test taking CSD into account ( demean option ) is also employed.
The empirical results shown in Table 5 reveal that the dependent variable ( \({\text{lnGDP}}\) ) contains a unit root but becomes stationary at first differences. The variable \({\text{lnPhysCap}}\) is stationary in its level form I(0), while for the variables \({\text{lnCHindex}}\) and \({\text{lnUnempl}}\) , the results are mixed. However, we can see that all series are stationary at their differences.
We then proceed to cointegration analysis to ascertain the presence of any long-term relationships among the variables. We run the Pedroni ( 1999 , 2004 ) approach that proposes a residual-based cointegration test for a cross-sectionally independent panel, the Kao ( 1999 ) test, which assumes homogeneity in the panels, and the Westerlund ( 2005 ) specification that mitigates the issues of optimal lag and bandwidth orders. However, these tests can generate biased estimates under cross-sectional dependence. So, we further apply the Westerlund and Edgerton ( 2007 ) and the Banerjee and Carrion-i-Silvestre ( 2017 ) panel cointegration tests. Footnote 11 All tests except the LM bootstrapping (Westerlund and Edgerton 2007 ) have a common null hypothesis of no cointegration. Footnote 12
According to the results shown in Table 6 , cointegration analysis supports a long run association between the variables for all panels. This result implies a long-term relationship among the variables under consideration across different regions and over time. Alternatively, we can say that this finding suggests that a stable equilibrium relationship binds the variables together in the long run so that we can estimate long run regressions.
Once cointegration is confirmed, we investigate all model variables' long run and short run dynamics. Given the presence of CSD and endogeneity, simple OLS, GLS, FE, and RE models might produce unreliable estimates (Sarafidis and Robertson 2009 ). Thus, we follow pooled ordinary least squares with Driscoll-Kraay ( 1998 ) standard errors ( POLS-DK ) and fixed effects with Driscoll-Kraay standard errors ( FE-DK ) that address cross-sectional dependence. We also use feasible generalized least squares ( FGLS ) model to account for heteroscedasticity and cross-section serial correlation (Bai et al. 2021 ). The results are shown in Table 7 . The following step is checking the long run and short run estimations through several specifications.
In line with recent empirical studies (O'Mahony and de Boer 2002 ; Abreu 2019 ), physical capital positively and significantly correlates with real economic growth, with an average (across models) value coefficient of 0.138. It implies that an increase of 1% in per head physical capital can boost economic growth by 0.14%. The importance of private and public investment is borne out by our results, given the crucial role the European Structural Funds play in infrastructure investment (Kachagia and Kyriazi 2021 ). The unemployment rate affects economic growth negatively, with an average value of − 0.158. Our findings indicate that a 1% increase in the unemployment rate is associated with a 0.16% drop in economic growth. This result supports the presence of an unemployment-income trade-off, aligning with previous studies for Greece, such as Christopoulos ( 2004 ) and Lolos and Papapetrou ( 2012 ).
Similarly to the impact of physical capital, our results reveal a long-term relationship between the Cultural Heritage index and economic growth. More specifically, a 1% increase in the Cultural Heritage index is associated with a 0.03% improvement in regional economic growth. Thus, there is empirical validation of a cultural heritage-led growth hypothesis, as the cultural heritage index (serving as a proxy variable for cultural tourism) exhibits a positive and statistically significant influence on regional income across all models. This finding aligns with previous research for China (Yang et al. 2010 ), Greece (Doulgeraki 2018 ; Kostakis et al. 2020 ) and European regions (Panzera 2023 ).
It should be noted that our analysis incorporates, by definition, only the effect of tangible cultural heritage assets. Intangible cultural events are not accounted for because of the non-availability of data. However, we know that many established events (e.g., the Athens and Epidaurus Festival, Patras Festival, Sani Festival, Philippi Festival) take place in the summer period in Greece, along with a significant number of ad hoc smaller-scale cultural events (local theatre performances, concerts, dancing festivals, exhibitions, religious fests, cine festivals, etc.) especially in the periphery. Footnote 13 Unfortunately, we do not have sufficient knowledge of the attention these events draw, but it might not be far from reality if we assume that the impact of the demand for intangible cultural events on the economy is comparable to that of tangible cultural heritage. Thus, we have good reasons to infer that the actual magnitude of the total effect of cultural heritage on income may well be about twice the estimated one. The estimations shown in Table 7 are robust to cross-sectional dependence, groupwise heteroscedasticity and serial correlation.
Furthermore, we investigate the relationship between the variables across the outcome variable's conditional distribution because we believe cultural heritage disparities may differ at various distribution points. To this end, we employ the novel approach of method of moments quantile regression (MMQR) developed by Machado and Silva ( 2019 ) that estimates quantile regressions with fixed effects at selected points of the conditional regional real GDP per capita distribution. This approach also allows valid conditional means estimations, while providing information on how the independent variables affect the entire conditional distribution (Hondroyiannis et al. 2022 ). This method accounts for cross-sectional dependence and slope heterogeneity. Table 8 presents the quantile regression estimates in nine deciles ( Q-0.1 to Q-0.9 , columns 2–10).
Empirical results (Table 8 ) show a substantial positive association between physical capital per head ( \({\text{lnPhysCap}}\) ) and real per capita income ( \({\text{lnGDP}}\) ) along the conditional real regional per capita income distribution. The coefficients of physical capital are positive and statistically significant at all percentiles, suggesting that physical capital is a strong growth driver for all regions, irrespectively of the conditional distribution of real GDP. Concerning the labour market indicator, results show a strong negative association between unemployment ( \({\text{lnUnempl}}\) ) and real income per capita. The effect is relatively more pronounced at the higher tails of the conditional income distribution. That is the impact of unemployment is greater in high-income regions.
Finally, the coefficients of the Cultural Heritage index ( \({\text{lnCHindex}}\) ) are positive and statistically significant across percentiles, except for the two upper tails ( Q-0.8 and Q-0.9 ). This finding implies that cultural heritage assets, as reflected in cultural tourism, significantly affect economic growth with a greater effect in regions with lower income levels. Essentially, this result indicates that cultural heritage assets have the potential to stimulate higher growth in lower and middle-income level regions, which are also those with lower attractiveness of cultural tourism (see also Fig. 3 ). This is an important finding, since policy actions to promote cultural heritage assets are more effective for lagging behind regions.
For the accuracy of the static estimations, several dynamic models that address endogeneity issues, such as GMM and IV estimators, Footnote 14 are also employed. We should instrument the potential endogenous variables in dynamic panel estimations that are more capable than static models in accounting for heterogeneity. This is primarily achieved by employing the lagged dependent variable and appropriate lags as instruments for the variables. So, we have incorporated a lagged dependent variable to model regional income, given its high correlation over time and tendency to change in incremental amounts. Including the lagged dependent variable captures the speed of adjustment of per capita income and delineates the independent variables' long-term impact. More specifically, we employ several system GMM dynamic models, including the Blundell and Bond ( 1998 ), the Arellano and Bover ( 1995 ), and the Ahn and Schmidt ( 1995 ) estimators with predetermined covariates and curtailed instruments as proposed by Kripfganz ( 2019 ). All models and parameters based on GMM and IV estimators are shown in Table 9 . As can be seen, dynamic GMM and IV estimators confirm previous findings.
Dependent variable: lnGDP to be similar with the rest of the tables
Subsequently, we employ the recently heterogeneous panel data model CS-ARDL approach (Chudik and Pesaran 2015 ) proposed by Ditzen ( 2021 ). This approach estimates both short run and long run coefficients with a mixed order of integration, tackling cross-sectional dependence (CSD) and endogeneity concerns through unit-specific autoregressive distributed lag (ARDL) specifications (Chudik et al. 2016 ). Moreover, CS-ARDL mitigates serial correlation, misspecification bias, and common correlation issues. Table 10 presents the empirical results.
According to the results of the CS-ARDL estimator, physical capital and cultural tourism contribution improve economic growth in the long run, while unemployment drops real income. Specifically, a 1% rise in physical capital per head could increase regional economic growth by 0.19%. Similarly, a 1% increase in the cultural heritage index may lead to higher economic growth by 0.13%. Note that estimating a second-generation approach (CS-ARDL) significantly improves the results. In our case, this approach gives cultural heritage assets a higher impact. Regarding unemployment, our results show that a drop in the unemployment rate by 1% could increase economic growth by 0.15%. Also, the short run effects of all variables are lower than in the long run. Finally, the results show that the error correction term is negative, less than one(-0.798), indicating that any short run imbalances return to equilibrium in around five quarters.
Finally, our study provides new empirical evidence on the causal linkage among economic growth, physical capital, unemployment rate and cultural heritage index. It is important to note that Granger causality identifies whether past values of one variable help predict another variable. We believe exploring relationships between physical capital, labour and cultural heritage variables, and economic growth is important. Also, Granger causality tests can guide model specification by helping researchers identify which variables should be included in a model to improve its forecasting performance.
Thus, a novel recently developed panel, the Granger non-causality approach, proposed by Juodis et al. ( 2021 ) and Xiao et al. ( 2023 ), is used. We follow the bootstrapping method to reduce cross-sectional dependence, and the heteroscedasticity proposed provides more detailed information about causal linkage. Table 11 reports the empirical outcomes of the bi-directional Granger association among the variables panel.
Granger-causal linkages indicate a significant bi-directional relationship between the unemployment rate and economic growth. Specifically, the unemployment rate negatively affects regional GDP (− 0.186), and economic growth adversely affects the unemployment rate (− 0.759). On the contrary, a one-way Granger causality runs from physical capital and Cultural Heritage index to regional economic growth. In particular, the results show that physical capital increases real GDP per capita in all Greek regions (0.211). Cultural tourism seems to significantly cause economic growth at regional level (0.130).
6 Conclusions and policy implications
This paper investigates the role of cultural heritage in determining economic activity by estimating a neoclassical empirical growth model augmented with a cultural heritage variable. The empirical model also accounts for other growth-related factors, such as physical capital, and unemployment. The case of Greece, a country with significant cultural heritage assets, is investigated over the period 2000–2019. The analysis is carried out at regional level, since cultural heritage assets have a strong local dimension.
Empirical results confirm that cultural heritage assets have a substantial impact on economic growth. Physical capital also has a substantial positive effect on income, while the impact of unemployment is negative. In addition, the quantile analysis shows that cultural heritage is more important in lagging behind regions that also attract lower numbers of cultural tourism. Finally, our results show the existence of a one-way causality from cultural heritage and physical capital to economic growth, while there is a two-way causality between the unemployment rate and income growth.
Given the empirical evidence of this study, several recommendations and policy guidelines can emerge to stimulate economic growth. In particular, our results call for formulating a coherent set of sustainable policy actions for promoting, preserving and developing cultural heritage with priority to regions with less visited historical monuments, which also happen to be the lagging behind regions. The success of these policies will lead to regional convergence.
These actions involve promoting monuments by disseminating information (tourist guides, leaflets), and highlighting local myths, legends and human presence over the centuries (habitation, land exploitation, art and industrial activities, food production and nutrition). They should also be carried out using information and communication technologies (creation of digital environments, websites, computer apps and videos, participation in social media, online information, digital presentation of collections, e-tickets etc.).The design and implementation of these actions will enhance regional social and cultural capital, by requiring specific and high quality skills (multilingual guides, historians, archaeologists, professional managers, computer experts, etc.) and upgrading traditional professions and businesses. Thus, a virtuous circle of promotion of cultural heritage, growth and development is introduced. A rich cultural heritage can position a region as an attractive location for businesses and entrepreneurs, as it can offer a unique environment and a pool of social capital to boost regional competiveness, incomes and wealth.
In addition, tourist policies, by shifting the emphasis from “Sea and Sun” towards “Sea, Sun and Culture” destinations, that is boosting cultural tourism, will increase the inflow of tourism to less visited monuments and regions by awarding them a local identity that will be further enhanced if connected to gastronomy and quality. These initiatives would expand the tourist period, boost employment and incomes and launch new investment, thus enhancing regional competitiveness, productive capacity and well-being. It goes without saying that cultural heritage must be viewed as an irreplaceable form of capital that must be wisely used, preserved, sustained and enhanced and not irretrievably consumed by over-tourism.
Reforms of public institutions are also required, especially in the operational framework of Greek public authorities that are responsible for all aspects of cultural heritage. The aforementioned actions will be fulfilled more efficiently if there is dear collaboration of local authorities with private institutions, along with decentralization of policy making. The public authorities should keep their supervisory role but the design and implementation of cultural heritage policies will be greatly facilitated by public–private partnership initiatives. Furthermore, in order to activate the important synergies among cultural heritage, tourism and gastronomy that enhance cultural capital, there should be an interactive cohabitation of private institutions and public authorities involved.
Note finally, that according to our empirical results, economic growth will be greatly benefited if policies are also pursued by boosting physical capital and combating unemployment. There is ample room for such actions given the availability of EU financing through the European Structural Funds and the NextGenerationEU.
Nevertheless, this study is subject to limitations mainly relating to the availability of quantitative information. In particular, our empirical analysis covers only tangible cultural heritage as quantitative data on intangible cultural heritage (handicrafts, festivals, concerts, traditions, gastronomy, etc.) is missing. We believe the volume of intangible cultural heritage is quite important, perhaps as important as the tangible counterpart. Thus, data construction describing the demand created by intangible cultural heritage is necessary. Also, to better understand the multifaceted role of cultural heritage in economic growth and development, more detailed social indicators should be produced to quantify social and cultural capital. In addition, quantitative data at local level, e.g. at NUTS III level, will definitely enrich the analysis.
Data availability
Data are available upon request.
Note that a recent Eurobarometer survey (European Union 2018 ) shows that the vast majority of European citizens think that cultural heritage is important to them personally (84%), as well as to their community (84%), region (87%), country (91%) and the EU as a whole (80%). Also, 79% of the responders agree (against 13% who disagree) that Europe's cultural heritage or cultural heritage-related activities create jobs in the EU.
Note that in the Eurobarometer survey (European Union 2018 ) the citizens were asked if their home is nearby any historical monuments or heritage sites, the positive answers in Greece were 70% (along the top 1/3 EU countries) against 60% of the EU28 average.
Events such as the Arab Spring and the Syrian civil war (2011); also the crisis of Crimea and Ukraine (2014).
For the list of monuments, see UNESCO (2018), https://whc.unesco.org/en/statesparties/gr .
According to Richards ( 2007 ) the growth of cultural tourism is largely driven by increased overall tourism.
See https://www.statistics.gr/en/statistics/-/publication/SCI21/ - for the list of archaeological sites and museums in Greece.
The thirteen NUTS II regions of Greece are Eastern Macedonia-Thrace (Anatoliki Makedonia-Thraki), Central Macedonia (Kentriki Makedonia), Western Macedonia (Dytiki Makedonia), Thessaly (Thessalia), Epirus (Ipeiros), Ionian Islands (Ionia Nisia), Western Greece (DytikiEllada), Central Greece (StereaEllada), Attica (Attiki), Peloponnesus (Peloponnisos), Northern Aegean (VoreioAigaio), Southern Aegean (NotioAigaio) and Crete (Kriti).
In the econometric analysis all variables are expressed in natural logarithms to obtain elasticities. They are denoted by the prefix ln .
The empirical approach followed is very much determined by the existing data. The available regional statistical information for Greece does not allow investigating cultural heritage in more detail, by adding more explanatory variables in the model, as in the study of Tubadji et al. ( 2022 ) for Italy.
The xtcips (Sangiácomo 2018 ) and pescadf (Lewandowski 2007 ) routines are applied in Stata software.
The Westerlund and Edgerton ( 2007 ) and Banerjee and Carrion-i-Silvestre ( 2017 ) routines are applied in GAUSS software.
The demean option has been employed in all first-generation tests to mitigate cross-sectional dependence.
Note that according to the Eurobarometer survey (European Union 2018 ), in the question to European citizens about the existence of intangible assets nearby their home (traditional events or festivals), the positive answers in Greece were 58% (along with Cyprus and Portugal) against 37% of the EU28 average.
The xtabond2 (Roodman 2009 ) and xtdpdgmm (Kripfganz 2019 ) routines are applied in Stata software.
Abreu M (2019) Neoclassical regional growth models. In: Fischer M, Nijkamp P (eds) Handbook of regional science. Springer, Berlin
Google Scholar
Acs ZJ, Audretsch DB (1988) Innovation in large and small firms: an empirical analysis. Am Econ Rev 78:678–690
Ahn SC, Schmidt P (1995) Efficient estimation of models for dynamic panel data. J Econom 68(1):5–27. https://doi.org/10.1016/0304-4076(94)01641-C
Article Google Scholar
Alberti FG, Giusti JD (2012) Cultural heritage, tourism and regional competitiveness: the Motor Valley cluster. City Cult Soc 3:261–273. https://doi.org/10.1016/j.ccs.2012.11.003
Altman M (2001) Culture, human agency, and economic theory: culture as a determinant of material welfare. J Socio-Econ 30:379–391. https://doi.org/10.1016/S1053-5357(01)00109-3
Apergis N, Rezitis A (2003) An examination of Okun’s law: evidence from regional areas in Greece. Appl Econ 35(10):1147–1151. https://doi.org/10.1080/0003684032000066787
Arellano M, Bover O (1995) Another look at the instrumental variable estimation of error-components models. J Econom 68(1):29–51. https://doi.org/10.1016/0304-4076(94)01642-D
Arezki R, Cherif R, Piotrowski J (2012) UNESCO World heritage list, tourism, and economic growth. In: Licciardi G, Amirtahmasebi R (eds) The economics of uniqueness: investing in historic city cores and cultural heritage assets for sustainable development. The World Bank, Washington, DC
Ashworth GJ (2013) Heritage and local development: a reluctant relationship. In: Rizzo I, Mignola A (eds) Handbook on the economics of cultural heritage. Edward Elgar, Cheltenham
Audretsch DB (2009) The entrepreneurial society. J Technol Transfer 34:245–254. https://doi.org/10.1007/s10961-008-9101-3
Backman M, Nilsson P (2018) The role of cultural heritage in attracting skilled individuals. J Cult Econ 42:111–138
Bacsi Z, Tóth É (2019) Word Heritage Sites as soft tourism destinations: their impacts on international arrivals and tourism receipts. Bull Geogr Socio-Econ Ser 45(45):25–44. https://doi.org/10.2478/bog-2019-0022
Bai J, Choi SH, Liao Y (2021) Feasible generalized least squares for panel data with cross-sectional and serial correlations. Empir Econ 60:309–326. https://doi.org/10.1007/s00181-020-01977-2
Baltagi BH, Feng Q, Kao C (2012) A Lagrange Multiplier test for cross-sectional dependence in a fixed effects panel data model. J Econom 170(1):164–177. https://doi.org/10.1016/j.jeconom.2012.04.004
Bandarin F, Hosagrahar J, Albernaz FS (2011) Why development needs culture. J Cult Heritage Manag Sustain Dev 1:15–25. https://doi.org/10.1108/20441261111129906
Banerjee A, Carrion-i-Silvestre JL (2017) Testing for panel cointegration using common correlated effects estimators. J Time Ser Anal 38(4):610–636. https://doi.org/10.1111/jtsa.12234
Bank of Greece (2019) Developments and prospects of inbound tourism, 2010–2019. Interim Report on Monetary policy, Box IV.3, 102–108 (in Greek)
Baum C (2001) XTTEST3: Stata module to compute Modified Wald statistic for groupwise heteroskedasticity
Bersvendsen T, Ditzen J (2020) xthst: Testing for slope homogeneity in Stata CEERP Working Paper Series No. 011. https://ceerp.hw.ac.uk/RePEc/hwc/wpaper/011.pdf
Beugelsdijk S, Van Schaik T (2005) Social capital and growth in European regions: an empirical test. Eur J Polit Econ 21(2):301–324. https://doi.org/10.1016/j.ejpoleco.2004.07.004
Beugelsdijk S, Van Schaik T, Arts W (2006) Toward a unified Europe? Explaining regional differences in value patterns by economic development, cultural heritage and historical shocks. Reg Stud 40(03):317–327
Blomquist J, Westerlund J (2013) Testing slope homogeneity in large panels with serial correlation. Econ Lett 121:374–378. https://doi.org/10.1016/j.econlet.2013.09.012
Blundell R, Bond S (1998) Initial conditions and moment restrictions in dynamic panel data models. J Econom 87(1):115–143. https://doi.org/10.1016/S0304-4076(98)00009-8
Bowitz E, Ibenholt K (2009) Economic impacts of cultural heritage: research and perspectives. J Cult Herit 10:1–8. https://doi.org/10.1016/j.culher.2008.09.002
Breusch TS, Pagan AR (1980) The Lagrange multiplier test and its applications to model specification in econometrics. Rev Econ Stud 47(1):239–253. https://doi.org/10.2307/2297111
Cerisola S (2019) A new perspective on the cultural heritage-development nexus: the role of creativity. J Cult Econ 43(1):21–56. https://doi.org/10.1007/s10824-018-9328-2
Chen P, Karavias Y, Tzavalis E (2022) Panel unit-root tests with structural breaks. Stand Genomic Sci 22(3):664–678. https://doi.org/10.1177/1536867X221124541
Choi I (2001) Unit root tests for panel data. J Int Money Finance 20(2):249–272. https://doi.org/10.1016/S0261-5606(00)00048-6
Chudik A, Pesaran MH (2015) Common correlated effects estimation of heterogeneous dynamic panel data models with weakly exogenous regressors. J Econom 188(2):393–420. https://doi.org/10.1016/j.jeconom.2015.03.007
Chudik A, Mohaddes K, Pesaran MH, Raissi M (2016) Long-run effects in large heterogeneous panel data models with cross-sectionally correlated errors. In: Essays in Honor of man Ullah. Emerald Group Publishing Limited, pp 85–135
Christopoulos DK (2004) The relationship between output and unemployment: evidence from Greek regions. Reg Sci 83:611–620. https://doi.org/10.1007/s10110-004-0198-y
Cuccia T, Guccio C, Rizzo I (2016) The effects of UNESCO world heritage list inscription on tourism destinations performance in italian regions. Econ Model 53:494–508. https://doi.org/10.1016/j.econmod.2015.10.049
Ditzen J (2021) Estimating long-run effects and the exponent of cross-sectional dependence: an update to xtdcce2. Stand Genomic Sci 21(3):687–707. https://doi.org/10.1177/1536867X2110450
Doulgeraki Ch (2018) Tourism and Economic Growth in Greece: 1970–2015. MA Dissertation, Department of Economic and Regional Development, Panteion University, Athens, Greece
Driscoll JC, Kraay AC (1998) Consistent covariance matrix estimation with spatially dependent panel data. Rev Econ Stat 80(4):549–560. https://doi.org/10.1162/003465398557825
Dritsakis N (2004) Tourism as a long-run economic growth factor: an empirical investigation for Greece using causality analysis. Tour Econ 10(3):305–316. https://doi.org/10.5367/0000000041895094
Drukker DM (2003) Testing for serial correlation in linear panel-data models. Stata J 3(2):168–177. https://doi.org/10.1177/1536867X0300300206
Dümcke C, Gnedovsky M (2013) The Social and Economic Value of Cultural Heritage: literature review. European Expert Network on Culture Paper, July
European Commission (2010) Europe 2020: A strategy for smart, sustainable and inclusive growth, COM (2010), final
European Commission (2015) Getting cultural heritage to work for Europe: report of the Horizon 2020 expert group on cultural heritage, European Commission Directorate-General for Research and Innovation.https://data.europa.eu/doi/ https://doi.org/10.2777/745666
European Commission (2018) A New European Agenda for Culture, COM (2018) 267 final
European Commission (2018a) Enhanced surveillance report-Greece. Staff Working Document SWD (2018) 508, November
European Union (2018) Special Eurobarometer Report 466: Cultural Heritage. Retrieved from https://data.europa.eu/data/datasets/s2150_88_1_466_eng?locale=en
Fan J, Liao Y, Yao J (2015) Power enhancement in high-dimensional cross-sectional tests. Econometrica 83:1497–1541. https://doi.org/10.3982/ECTA12749
Faro Convention (2005) Council of Europe framework convention on the value of cultural heritage for society, Council of Europe Treaty Series - No. 199, Faro
Faria JR, León-Ledesma ML (2008) Cultural heritage and growth. Studies in Economics 0303, School of Economics, University of Kent
Faria JR, León-Ledesma M (2022) Patrimonio Cultural e Crescimento, Planejamento E PolíticasPúblicas, 31. www.ipea.gov.br/ppp/index.php/PPP/article/view/14
Fotopoulos G, Spence N (1998) Spatial variations in new manufacturing plant openings: some empirical evidence from Greece. Reg Stud 33:219–229. https://doi.org/10.1080/00343409950082418
Granato J, Inglehart R, Leblang D (1996) The effect of culture on economic development: theory hypotheses and some empirical tests. Am J Polit Sci 40:607–631
Gray HP (1996) Culture and economic performance: policy as an intervening variable. J Comp Econ 23:278–291. https://doi.org/10.1006/jcec.1996.0065
Greene W (2000) Econometric analysis. Prentice-Hall, New York
Hampton MP (2005) Heritage, local communities and economic development. Ann Tour Res 32:735–759. https://doi.org/10.1016/j.annals.2004.10.010
Hondroyiannis G, Papapetrou E, Tsalaporta P (2022) New insights on the contribution of human capital to environmental degradation: evidence from heterogeneous and cross-correlated countries. Energy Econ 116:106–416. https://doi.org/10.1016/j.eneco.2022.106416
IMF-International Monetary Fund (2019) Greece-Selected Issues. Country Report No. 19/341, IMF, Washington DC
Juodis A, Karavias Y, Sarafidis V (2021) A homogeneous approach to testing for Granger non-causality in heterogeneous panels. Empir Econ 60:93–112. https://doi.org/10.1007/s00181-020-01970-9
Juodis A, Reese S (2022) The incidental parameters problem in testing for remaining cross-section correlation. J Bus Econ Stat 40(3):1193–1203. https://doi.org/10.1080/07350015.2021.1906687
Kao C (1999) Spurious regression and residual-based tests for cointegration in panel data. J Econom 90:1–44. https://doi.org/10.1016/S0304-4076(98)00023-2
Kechagia A, Kyriazi F (2021) Structural funds and regional economic growth: the Greek experience. Rev Econ Anal 13(4):501–532
Kapetanios G, Pesaran MH, Yamagata T (2011) Panel with non-stationarity multifactorerrorstructures. J Econom 160:326–348. https://doi.org/10.1016/j.jeconom.2010.10.001
Katircioglu ST (2009) Revisiting the tourism-led-growth hypothesis for Turkey using the bounds test and Johansen approach for cointegration. Tour Manag 30(1):17–20. https://doi.org/10.1016/j.tourman.2008.04.004
Kostakis I, Lolos S, Doulgeraki C (2020) Cultural Heritage led Growth: Regional evidence from Greece (1998–2016).MPRA_paper_98443. https://mpra.ub.uni-muenchen.de/98443/
Kripfganz S (2019) Generalized method of moments estimation of linear dynamic panel data models. In: Proceedings of the 2019 London Stata Conference
Lee W, Chhabra D (2015) Heritage hotels and historic lodging: perspectives on experiential marketing and sustainable culture. J Herit Tour 10:103–110. https://doi.org/10.1080/1743873X.2015.1051211
Lewandowski P (2007) PESCADF: Stata module to perform Pesaran's CADF panel unit root test in presence of cross section dependence. https://EconPapers.repec.org/RePEc:boc:bocode:s456732
Liargovas P, Repousis S (2015) Development paths in the knowledge economy: innovation and entrepreneurship in Greece. J Knowl Econ 6:1063–1077. https://doi.org/10.1007/s13132-013-0176-1
Licciardi G, Amirtahmasebi R (eds) (2012) The economics of uniqueness: investing in historic city cores and cultural heritage assets for sustainable development. World Bank, Washington DC. https://doi.org/10.1596/978-0-8213-9650-6
Book Google Scholar
Lolos S, Papapetrou E (2012) Unemployment disparities and persistence assessing the evidence from Greek regions, 1981–2008. Reg Sect Econ Stud 12:55–77
Lolos S, Palaios P, Papapetrou E (2021) Tourism-led growth asymmetries in Greece: evidence from quantile regression analysis. Portuguese Econ J. https://doi.org/10.1007/s10258-021-00195-7
Loulanski T, Loulanski V (2011) The sustainable integration of cultural heritage and tourism: a meta-study. J Sustain Tour 19(7):837–862. https://doi.org/10.1080/09669582.2011.553286
Louri H, Anagnostaki V (1995) Entry in Greek manufacturing industry: Athens vs. the rest of Greece. Urban Stud 32:1127–1133. https://doi.org/10.1080/00420989550012609
Machado JA, Silva JS (2019) Quantiles via moments. J Econom 213(1):145–173. https://doi.org/10.1016/j.jeconom.2019.04.009
Malecki EJ (2012) Regional social capital: Why it matters. Reg Stud 46(8):1023–1039. https://doi.org/10.1080/00343404.2011.607806
Nocca F (2017) The role of cultural heritage in sustainable development: multidimensional indicators as decision-making tool. Sustainability 9(1882):2–28. https://doi.org/10.3390/su9101882
OECD (2008) The impact of culture on tourism. OECD Publishing, Paris. https://doi.org/10.1787/9789264040731-en
OECD (2009) OECD Economic Surveys: Greece 2009, OECD, Paris https://doi.org/10.1787/eco_surveys-grc-2009-en
OECD (2023) OECD Economic Surveys: Greece, 2023, OECD, Paris. https://doi.org/10.1787/c5f11cd5-en
Okun AM.(1962) Potential GNP: its measurement and significance. Proceedings of the business and economic statistics section, American Statistical Association.
O’Mahony M, de Boer W (2002) Britain’s relative productivity performance: Has anything changed? Natl Inst Econ Rev 179:38–43. https://doi.org/10.1177/002795010217900106
Panzera E, de Graaff T, de Groot HLF (2021) European cultural heritage and tourism flows: the magnetic role of superstarworld heritage sites. Pap Reg Sci 100:101–122. https://doi.org/10.1111/pirs.12562
Panzera E (2023) From Cultural Heritage to Economic Development Through Tourism, Chapter 3, Cultural Heritage and Territorial Identity: Synergies and Development Impact on European Regions, Springer. https://doi.org/10.1007/978-3-030-94468-1
Pasquinelli C, Rovai S, Bellini N (2023) Linking place brands and regional innovation: sustainable business strategies leveraging heritage. Reg Stud. https://doi.org/10.1080/00343404.2023.2187046
Pedroni P (1999) Critical values for cointegration tests in heterogeneous panels with multiple regressors. Oxford Bull Econ Stat 61:653–670. https://doi.org/10.1111/1468-0084.0610s1653
Pedroni P (2004) Panel cointegration: asymptotic and finite sample properties of pooled time series tests with an application to the PPP hypothesis. Econom Theory 20(3):597–625. https://doi.org/10.1017/S0266466604203073
Pesaran MH (2004) General diagnostic tests for cross section dependence in panels. Cambridge working papers in economics. No: 0435, Faculty of Economics, University of Cambridge
Pesaran MH (2007) A simple panel unit root test in the presence of cross-sectiondependence. J Appl Economet 22:265–312. https://doi.org/10.1002/jae.951
Pesaran MH, Ullah A, Yamagata T (2008) A bias-adjusted LM test of error cross section independence. Economet J 11:105–127. https://doi.org/10.1111/j.1368-423X.2007.00227.x
Pesaran MH, Yamagata T (2008) Testing slope homogeneity in large panels. J Εconom 142(1):50–93. https://doi.org/10.1016/j.jeconom.2007.05.010
Pesaran MH (2015) Testing weak cross-sectional dependence in large panels. Economet Rev 34(6–10):1089–1117. https://doi.org/10.1080/07474938.2014.956623
Pesaran MH (2021) General diagnostic tests for cross-sectional dependence in panels. Empir Econ 60:13–50. https://doi.org/10.1007/s00181-020-01875-7
Pesaran MH, Xie Y (2023) A bias-corrected CD test for error cross- sectional dependence in panel data models with latent factors (v3). Cornell University. https://doi.org/10.48550/arXiv.2109.00408
Richards G (2002) Tourism attraction systems: exploring cultural behavior. Ann Bus Res 29:1048–1064. https://doi.org/10.1016/S0160-7383(02)00026-9
Richards G (2007) Cultural tourism: global and local perspectives. Haworth Press, New York
Richards G (2018) Cultural tourism: a review of recent research and trends. J Hosp Tour Manag 36:12–21. https://doi.org/10.1016/j.jhtm.2018.03.005
Rodríguez-Pose A (2013) Do institutions matter for regional development? Reg Stud 47(7):1034–1047. https://doi.org/10.1080/00343404.2012.748978
Rodríguez-Pose A (2020) Institutions and the fortunes of territories. Reg Sci Policy Pract 12(3):371–386
Romão J, Neuts B (2017) Territorial capital, smart tourism specialization and sustainable regional development: Experiences from Europe. Habitat Int 68:64–74. https://doi.org/10.1016/j.habitatint.2017.04.006
Roodman D (2009) How to do xtabond2: an introduction to difference and system GMM in stata. Stata J 9:86–136. https://doi.org/10.1177/1536867X0900900106
Saccone D, Bertaccini E (2011) Culture, heritage and economic development-empowering developing countries. Working Paper New Series, University of Turin
Sangiácomo M (2018) XTCIPS: Stata module to compute Pesaran panel unit root test in the presence of cross-section dependence. https://EconPapers.repec.org/RePEc:boc:bocode:s457850
Sarafidis V, Robertson D (2009) On the impact of error cross-sectional dependence in short dynamic panel estimation. Econom J 12(1):62–81. https://doi.org/10.1111/j.1368-423X.2008.00260.x
Sasaki M (2010) Urban regeneration through cultural creativity and social inclusion: rethinking creative city theory through a Japanese case study. Cities 27:S3–S9. https://doi.org/10.1016/j.cities.2010.03.002
Scott AJ (2000) The cultural economy of cities: essays on the geography of image-producing industries. The Cultural Economy of Cities, 1–256
Škrabic B, Šimundic B, Muštra V, Vugdelija M (2021) The role of UNESCO cultural heritage and cultural sector in tourism development: the case of EU countries. Sustainability 13:5473. https://doi.org/10.3390/su13105473
Solow RM (1956) A contribution to the theory of economic growth. Q J Econ 70(1):65–94. https://doi.org/10.2307/1884513
Srakar A, Vecco M (2017) Ex-ante versus ex-post : comparison of the effects of the European Capital of Culture Maribor 2012 on tourism and employment. J Cult Econ 41:197–214. https://doi.org/10.1007/s10824-017-9294-0
Throsby D (1999) Cultural Capital. J Cult Econ 23:3–12. https://doi.org/10.1023/A:1007543313370
Throsby D (2012) Heritage economics: a conceptual framework. In: Licciardi G, Amirtahmasebi R (eds) The economics of uniqueness: investing in historic city cores and cultural heritage assets for sustainable development. The World Bank, Washington, DC
Tubadji A, Gheasi M, Crociata A, Odoardi I (2022) Cultural capital and income inequality across Italian regions. Reg Stud 56(3):459–475
UNWTO - World Tourism Organization (2018) Tourism and Culture Synergies. UNWTO, Madrid
Westerlund J (2005) New simple tests for panel cointegration. Econom Rev 24:297–316. https://doi.org/10.1080/07474930500243019
Westerlund J, Edgerton DL (2007) A panel bootstrap cointegration test. Econ Lett 97(3):185–190. https://doi.org/10.1016/j.econlet.2007.03.003
Wooldridge JM (2002) Econometric analysis of cross section and panel data. MIT Press, Cambridge, MA
Xiao J, Karavias Y, Juodis A, Sarafidis V, Ditzen J (2023) Improved tests for Granger noncausality in panel data. Stand Genomic Sci 23(1):230–242. https://doi.org/10.1177/1536867X231162034
Yang C, Lin H, Han C (2010) Analysis of international tourist arrivals in China: the role of world heritage sites. Tour Manage 31:827–837. https://doi.org/10.1016/j.tourman.2009.08.008
Download references
Open access funding provided by HEAL-Link Greece. This research has not received any grant from funding agencies in the public, private, or non-profit sectors.
Author information
Authors and affiliations.
Department of Economics and Sustainable Development, Harokopio University of Athens, 70 Eleftheriou Venizelou, 176 76, Athens, Greece
Ioannis Kostakis
Department of Economic and Regional Development, Panteion University, Athens, Greece
Sarantis Lolos
You can also search for this author in PubMed Google Scholar
Contributions
Both authors contributed equally.
Corresponding author
Correspondence to Ioannis Kostakis .
Ethics declarations
Conflict of interest.
The authors declare that they have no conflict of interest.
Additional information
Publisher's note.
Springer Nature remains neutral with regard to jurisdictional claims in published maps and institutional affiliations.
Rights and permissions
Open Access This article is licensed under a Creative Commons Attribution 4.0 International License, which permits use, sharing, adaptation, distribution and reproduction in any medium or format, as long as you give appropriate credit to the original author(s) and the source, provide a link to the Creative Commons licence, and indicate if changes were made. The images or other third party material in this article are included in the article's Creative Commons licence, unless indicated otherwise in a credit line to the material. If material is not included in the article's Creative Commons licence and your intended use is not permitted by statutory regulation or exceeds the permitted use, you will need to obtain permission directly from the copyright holder. To view a copy of this licence, visit http://creativecommons.org/licenses/by/4.0/ .
Reprints and permissions
About this article
Kostakis, I., Lolos, S. Uncovering the impact of cultural heritage on economic growth: empirical evidence from Greek regions, 2000–2019. Ann Reg Sci (2024). https://doi.org/10.1007/s00168-024-01280-3
Download citation
Received : 13 July 2023
Accepted : 21 April 2024
Published : 16 May 2024
DOI : https://doi.org/10.1007/s00168-024-01280-3
Share this article
Anyone you share the following link with will be able to read this content:
Sorry, a shareable link is not currently available for this article.
Provided by the Springer Nature SharedIt content-sharing initiative
- Cultural heritage
- Regional economic growth
JEL Classification
- Find a journal
- Publish with us
- Track your research

IMAGES
VIDEO
COMMENTS
Numerous studies have focused on delineating the relationship between tourism and economic growth. In this article, we present the results of a rigorous meta-regression analysis based on 545 estimates drawn from 113 studies that empirically tested the tourism-led growth hypothesis (TLGH).
Abstract. Numerous studies have focused on delineating the relationship between tourism and economic growth. In this article, we present the results of a rigorous meta-regression analysis based on 545 estimates drawn from 113 studies that empirically tested the tourism-led growth hypothesis (TLGH).
For example, a meta-regression analysis based on 113 studies supports the statistically positive effects of tourism activities on economic growth in a country or a region, while the effects are ...
Numerous studies have focused on delineating the relationship between tourism and economic growth. In this article, we present the results of a rigorous meta-regression analysis based on 545 estimates drawn from 113 studies that empirically tested the tourism-led growth hypothesis (TLGH). The results suggest the presence of publication bias in the literature on this topic, where the majority ...
These variables inform the development of the meta-regression model of our study as we explain in the following section. Meta-regression Analysis A meta-regression analysis can help to explain the extent to which the particular choice of data, specification techniques, and methodological approaches influence the reported results (Stanley 2001).
In this article, we present the results of a rigorous meta-regression analysis based on 545 estimates drawn from 113 studies that empirically tested. Numerous studies have focused on delineating the relationship between tourism and economic growth. In this article, we present the results of a rigorous meta-regression analysis based on 545 ...
@article{Nunkoo2020TourismAE, title={Tourism and Economic Growth: A Meta-regression Analysis}, author={Robin Nunkoo and Boopen Seetanah and Zameelah Rifkha Khan Jaffur and Paul George Warren Moraghen and Raja Vinesh Sannassee}, journal={Journal of Travel Research}, year={2020}, volume={59}, pages={404 - 423} }
Numerous studies have focused on delineating the relationship between tourism and economic growth. In this article, we present the results of a rigorous meta-regression analysis based on 545 estimates drawn from 113 studies that empirically tested the tourism-led growth hypothesis (TLGH). The results suggest the presence of publication bias in the literature on this topic, where the majority of...
Show simple item record. Tourism and Economic Growth: A Meta-regression Analysis
All of Griffith Research Online. Communities & Collections; Authors; By Issue Date; Titles; This Collection
Numerous studies have focused on delineating the relationship between tourism and economic growth. In this article, we present the results of a rigorous meta-regression analysis based on 545 estimates drawn from 113 studies that empirically tested the tourism-led growth hypothesis (TLGH).
Numerous studies have focused on delineating the relationship between tourism and economic growth. In this article, we present the results of a rigorous meta-regression analysis based on 545 estimates drawn from 113 studies that empirically tested the tourism-led growth hypothesis (TLGH). The results suggest the presence of publication bias in ...
This work is divided into six sections which describe the meta-analysis approach taken with respect to tourism-economic growth. 2. METHODOLOGY. Following Glass et al. (1981) and Lipsey & Wilson (2001), the meta-analysis method consists of deducing a summary effect based on the combination of different
From a sample of 11 studies based on panel data techniques published through to 2011, and for a total of 87 heterogeneous estimations, a meta-analysis is performed by applying models for both ...
This work analyzes whether tourism development affects the economic growth. Using yearly data of 1995-2017 for the sample of 35 countries, the empirical findings reveal that tourism arrivals and tourism receipts stimulate the economic growth. Similarly, renewable and non-renewable energy consumption enhance economic growth in the selected countries. Further, human capital positively affects ...
Three-quarters (74%) of the countries experiencing growth in tourism demand are high-income (51%) and upper-middle-income (23%) countries. Among them, 84% may experience demand growth rates exceeding 1.5% solely based on the climate effect, with Canada, Russia, Finland, Norway and Sweden being the top beneficiaries.
1. Introduction. The absence of regional coordination and collaboration in tourism growth can lead to destination competition and slow economic development [].In contrast, cooperation between organizations and countries can result in advantageous partnerships that facilitate the attainment of shared objectives [].Previous research argued that regional tourism cooperation is the current trend ...
Numerous studies have focused on delineating the relationship between tourism and economic growth. In this article, we present the results of a rigorous meta-regression analysis based on 545 estimates drawn from 113 studies that empirically tested the tourism-led growth hypothesis (TLGH). The results suggest the presence of publication bias in the literature on this topic,
This study employs a meta-analysis method to reanalyze 140 effect sizes from 32 independent research samples across diverse global contexts. ... Endogenous growth theory underscores the central role of knowledge and technology in economic growth . On ... For instance, Stanley (2021) used multiple linear regression analysis to analyze the ...
Figure 6. Bayesian model averaging (BMA) model inclusion for the tourism and economic growth nexus. Note: Response variable: partial correlation coefficient (PCC). Columns denote individual models. The variables are sorted in descending order of posterior inclusion probabilities. A blue-shaded cell implies that the variable is included with a positive estimated sign; a red-shaded cell implies ...
New York, USA, 21 May 2024 - International tourist arrivals and the travel and tourism sector's contribution to global GDP are expected to return to pre-pandemic levels this year, driven by the lifting of COVID-19-related travel restrictions and strong pent-up demand, as per the new World Economic Forum travel and tourism study, released ...
In this article, we sum up the literature on the study of Granger causality relationships between tourism and income using a meta-regression analysis. We conclude that the acceptance of the tourism-led growth hypothesis is likelier the higher the countries' degree of tourism specialization and population size.
Main. Emerging infectious diseases are on the rise, often originate from wildlife, and are significantly correlated with socioeconomic, environmental and ecological factors 1. As a consequence ...
An Empirical Study on Tourism and Economic Growth in Greece: An Autoregressive Distributed Lag Boundary Test Approach. The objective of this research is to analyze the impact of the tourism industry on the economic growth of Greece. The study employs empirical analysis and time series econometric techniques to….
This paper brings empirical evidence on the role of cultural heritage assets in promoting economic growth. The case of Greece at regional level over the period 2000-2019 is taken as an example, owing to important cultural heritage endowment. Economic growth is approached by formulating a neoclassical growth model augmented with a dynamic cultural heritage index. The relationship between ...
The positive effect of the tourism industry on economic growth has been extensively demonstrated [1,2,3].In recent years, cultural tourism has become the main growth area of the tourism industry, and thus, researchers are paying increasing attention to cultural tourism [4,5].Therefore, the Chinese government has introduced a series of policies promoting the integration of the cultural and ...
Understanding the relationship between the intake of sugars and diet quality can inform public health recommendations. This systematic review synthesized recent literature on associations between sugar intake and diet quality in generally healthy populations aged 2 years or older. We searched databases from 2010 to 2022 for studies of any design examining associations between quantified sugar ...
Table 4. Bayesian Model Averaging. - "Tourism and Economic Growth: A Meta-regression Analysis" Skip to search form Skip to main content Skip to account menu ... @article{Nunkoo2020TourismAE, title={Tourism and Economic Growth: A Meta-regression Analysis}, author={Robin Nunkoo and Boopen Seetanah and Z Khan Jaffur and Paul George Warren Moraghen ...