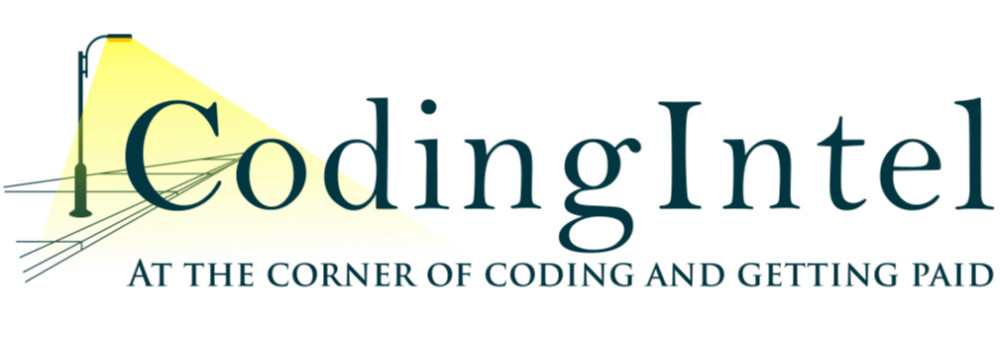
- Become a Member
- Everyday Coding Q&A
- Can I get paid
- Coding Guides
- Quick Reference Sheets
- E/M Services
- How Physician Services Are Paid
- Prevention & Screening
- Care Management & Remote Monitoring
- Surgery, Modifiers & Global
- Diagnosis Coding
- New & Newsworthy
- Practice Management
- E/M Rules Archive
June 6, 2024

Coding for Prolonged Services: CPT and HCPCS Codes

Common rules:
- Prolonged services codes are add-on codes to E/M services.
- In order to use prolonged care, the primary code must be selected based on time. This is in the CPT and HCPCS definition of prolonged services.
- Prolonged services codes may only be added to the highest-level code in the category.
- The full 15 minutes of prolonged services must be met. These do not follow the CPT mid-point time rule.
- The work of the prolonged care may include both face-to-face and non-face-to-face time.
- Prolonged care services can no longer be used on psychotherapy codes. There is no replacement code.
Where the rules vary:
- There are different CPT® and HCPCS codes that describe the same prolonged care services.
- In the 2024 CPT book, time ranges were removed from the office visit codes, and they now have only a single, threshold time listed. CMS has changed its manual or time thresholds for using prolonged care in response to this.
- For other services (hospital, nursing facility and home and residence services), CPT® uses the times stated in the CPT® book for the primary code when calculating if a prolonged services code may be added. CMS uses the time in the CMS time file , which includes pre and post visit times on other days, to calculate if prolonged care services may be added to hospital, nursing facility and home and residence services.
- CPT® includes only time spent on the date of the encounter. For hospital, nursing facility and home and residence services, CMS uses time on other dates of service.
- CPT® still has non-face-to-face prolonged care in the CPT® book, codes 99358, +99359 which can be used on days that do not include a face-to-face visit. CMS has given them a status indicator of invalid and doesn’t pay for them. There is no replacement of these services for Medicare patients.
- Home and residence services
- Hospital services
- Nursing facility for services
- Table 24 from the Final rule
Implementing prolonged services codes
Coding prolonged services in the office.
CMS does not recognize consultation codes.
Note: For home and residence services and assessment of cognitive functions, see below.
Coding for prolonged services is complicated by the fact CPT ® and CMS use different codes and different time thresholds. These codes and rules have been in effect since 2021.
- The AMA developed CPT ® code 99417 for 15 minutes of prolonged care, done on the same day as office/outpatient codes 99205 and 99215.
- Medicare has assigned a status indicator of invalid to code 99415, and developed a HCPCS code to replace it, G2212
- If using either code, only report it with codes 99205 and 99215, use only clinician time, and use it only when time is used to select the code
- Use for time spent face-to-face and in non-face-to-face activities
In their 2021 Physician Fee Schedule Final Rule, CMS indicated its agreement with the new E/M definitions for codes 99202-99215 that were developed by the AMA that are in the 2021 CPT ® book. However, CMS and the AMA are not in agreement about the use of prolonged care code 99417, resulting in HCPCS code.
Using time for E/M services
A practitioner may include these activities in their time, when using time to select an E/M service:
- preparing to see the patient (eg, review of tests)
- obtaining and/or reviewing separately obtained history
- performing a medically appropriate examination and/or evaluation
- counseling and educating the patient/family/caregiver
- ordering medications, tests, or procedures
- referring and communicating with other health care professionals (when not separately reported)
- documenting clinical information in the electronic or other health record
- independently interpreting results (not separately reported) and communicating results to the patient/family/caregiver
- care coordination (not separately reported)
Per CPT, use 99417 for office visits, outpatient consults, home and residence services and cognitive assessment planning.
# ✚ 99417 Prolonged office or other outpatient evaluation and management service(s) (beyond the total time of the primary procedure which has been selected using total time), requiring total time with or without direct patient contact beyond the usual service, on the date of the primary service; each 15 minutes (List separately in addition to codes 99205, 99215 for office or other outpatient Evaluation and Management services)
(Use 99417 in conjunction with 99205, 99215, 99245, 99345, 99350, 99483)
(Use 99417 in conjunction with 99483, when the total time on the date of the encounter exceeds the typical time of 99483 by 15 minutes or more.)
- You can’t report the new add on code on the same day as 90833, 90936, 90838, non-face-to-face prolonged care codes 99358, 99359 or staff prolonged care codes.
- The time reported must be 15 minutes, not 7.5 minutes. The entire 15 minutes must be done, in order to add on this new, prolonged services code.
CMS developed its own code G2212
G2212 Prolonged office or other outpatient evaluation and management service(s) beyond the maximum required time of the primary procedure which has been selected using total time on the date of the primary service; each additional 15 minutes by the physician or qualified healthcare professional, with or without direct patient contact (List separately in addition to CPT ® codes 99205, 99215 for office or other outpatient evaluation and management services)
(Do not report G2212 on the same date of service as 99354, 99355, 99358, 99359, 99415, 99416). (Do not report G2212 for any time unit less than 15 minutes)).”
Both CMS and CPT allow a prolonged service in addition to 99483, assessment of and care planning for a patient with cognitive impairment, requiring an independent historian, in the office or other outpatient, home or domiciliary or rest home. The typical time for this code is 60, making the threshold time to add a prolonged care code 75 minutes. Note that CMS allows the practitioner to include time spent three days before the date of the visit and seven days after.
Coding prolonged services in a home or residence
For CPT®, use add-on code 99417 for prolonged care. As with all of these codes, both CPT ®️ and HCPCS, the prolonged code may only be added to the highest-level code in the category and then only when time is used to select the service. The definition of 99417 is above.
G0318 ( Prolonged home or residence evaluation and management service(s) beyond the total time for the primary service (when the primary service has been selected using time on the date of the primary service); each additional 15 minutes by the physician or qualified healthcare professional, with or without direct patient contact (list separately in addition to CPT codes 99345, 99350 for home or residence evaluation and management services). (Do not report G0318 on the same date of service as other prolonged services for evaluation and management 99358, 99359, 99417). (Do not report G0318 for any time unit less than 15 minutes) )
CMS is allowing time on days prior to and after the date of the encounter to be used for prolonged services in relation to home/residence visits.
Coding prolonged services in the hospital: CPT and HCPCS codes
99418 Prolonged inpatient or observation evaluation and management service(s) time with or without direct patient contact beyond the required time of the primary service when the primary service level has been selected using total time, each 15 minutes of total time (List separately in addition to the code of the inpatient and observation Evaluation and Management service)
(Use in 99418 conjunction with 99223, 99233, 99236, 99255, 99306, 99310) (Do not report 99418 on the same date of service as 90833, 90836, 90838, 99358, 99359) (Do not report 99418 for any time unit less than 15 minutes)
99418 may be used on the highest-level initial and subsequent inpatient and observation codes, inpatient consult, and initial and subsequent nursing facility services. It may not be reported with psychotherapy or non-face to face prolonged care codes, or discharge services 99238, 99239, 99315, 99316. It may not be used with Emergency Department codes. The full 15 minutes is required and time must have been used to select the level of service.
As expected, CMS is not recognizing the new CPT ® code 99418. For Medicare patients, there is a HCPCS code. CMS is not using the published CPT typical times for the codes, but the time in the CMS time file, developed by the RUC. For Medicare patients, the time thresholds to add G0316 are different than those in our CPT books. CMS is not using allowing practices to report G0316 when the time is 15 more minutes than the CPT ® typical time. Instead, in a break from prior policy, CMS is using the time in the CMS time file. The 2023 time file is here .
G0316 Prolonged hospital inpatient or observation care evaluation and management service(s) beyond the total time for the primary service (when the primary service has been selected using time on the date of the primary service); each additional 15 minutes by the physician or qualified healthcare professional, with or without direct patient contact (list separately in addition to CPT ® codes 99223, 99233, and 99236 for hospital inpatient or observation care evaluation and management services). (Do not report G0316 on the same date of service as other prolonged services for evaluation and management 99358, 99359, 99418). (Do not report G0316 for any time unit less than 15 minutes)
See the CMS Table 24 below. CMS is allowing time on after the date of the encounter to be used for prolonged services in relation to hospital services.
Coding prolonged services in a nursing facility
Prolonged services in a nursing facility: CPT code 99418/HCPCS code for Medicare G0317
CPT ® defines the new prolonged add-on code 99418 (above) as the code to use in a nursing facility, as well as in the hospital. And, CPT ®️ simply states to use the code when the total time of the highest-level service (selected based on time) is 15 minutes more than the time described in the CPT ®️ book. Both the base time and the prolonged time can include face-to-face care and non-direct care on the date of the visit.
G0317 ( Prolonged nursing facility evaluation and management service(s) beyond the total time for the primary service (when the primary service has been selected using time on the date of the primary service); each additional 15 minutes by the physician or qualified healthcare professional, with or without direct patient contact (list separately in addition to CPT codes 99306, 99310 for nursing facility evaluation and management services). (Do not report G0317 on the same date of service as other prolonged services for evaluation and management 99358, 99359, 99418,). (Do not report G0317 for any time unit less than 15 minutes) )
Table 24 Required Time Thresholds to Report Other E/M Prolonged Services
* Time must be used to select visit level. Prolonged service time can be reported when furnished on any date within the primary visit’s surveyed timeframe, and includes time with or without direct patient contact by the physician or NPP. Consistent with CPT’s approach, we do not assign a frequency limitation.
The source of this chart is CMS’s 2023 Final Rule. It doesn’t follow CPT typical times, or CPT prolonged services rules. It includes time for some services on the days before or after the face-to-face encounter. It adds to confusion and complexity for medical practices.
Implementation of using prolonged care HCPCS codes
It was never easy for clinicians to select prolonged services codes. When they were applicable to all levels of service, the threshold time was different for each code. Now, they are only applicable on the highest level of service, but there are two sets of codes and the time thresholds are different for each one. This makes no sense. Effectively, all prolonged services coding will need to be done by coders. Effectively, it is so byzantine that most practices will never be able to bill for them.
Add-on prolonged services HCPCS codes
Can an add-on code to be submitted without its primary code? In particular, the add-on prolonged services HCPCS codes developed by CMS.
An add-on code must be submitted with its primary code. A colleague said she was getting conflicting opinions about this. Let’s see what CPT® and CMS say.
Page xviii of the CPT® Professional Edition 2024 states, “Add-on codes are always performed in addition to the primary service or procedure and must never be reported as a standalone code.” It is easy to ignore the information in the introduction of the CPT® book but when I’m stuck, I regularly find answers there. And wish I had started looking there in the first place!
What about CMS? CMS has edits in place to ensure that an add-on code is only paid when reported with a correct primary code. Naturally, they have three levels of edits but you can read about this on the CMS website .
I think the question was prompted by the fact that for certain services provided by practitioners in a facility the add-on prolonged care codes includes time the days before or in the days after the face-to-face encounter. You can see the chart from the CMS final rule and read about it here .
I don’t know what edits individual MACs are setting up for these codes, but I recommend that you continue to submit all add-on codes on the claim with the primary code, following CPT® rules and CMS guidance.
Non-face-to-face prolonged services codes 99358, 99359
The non-face-to-face prolonged care codes are still active, billable codes. But, they may not be reported on the same date of service as 99202-99215 per CPT®. And, Medicare has given them a status code of invalid, which means they won’t pay for it. And, there is not a replacement code for this service for Medicare.
I understand from your article about prolonged services in 2021 that CMS won’t pay for prolonged code 99417 and instead developed a HCPCS code for the service. (G2212) Do you have any recommendations about how to manage this in the office?
Although in general, I believe most clinicians can code for most of the work they do (not a universally held opinion, I know) this is a case where the claims must go to a coder for review. Not only are there different codes depending on payer, the time thresholds are different. CPT® allows you to add the 15 minutes to the lower time threshold in the range, and CMS requires you to add the 15 minutes to the higher time threshold in the range.
Just a few reminders. The prolonged codes can only be used on 99205 and 99215, and only when time is used to select the office visit code. The total time must be documented. CMS’s manual does not currently require start and stop times. Look for a description of what activities are included in the time, because this is required when using time to select the office visit codes. “I spent 90 minutes caring for the patient today. It included reviewing test results, documenting in the record and arranging for follow up at pain management. It also included an extensive discussion with the patient and his sister about treatment options and recovery time, if he decides on surgery.”
Source documents
- EM from 2020 Final Rule
- G2212 from 2021 Final Rule
Get more tips and coding insights from coding expert Betsy Nicoletti.
Subscribe to receive our FREE monthly newsletter and Everyday Coding Q&A.
Last revised May 7, 2024 - Betsy Nicoletti Tags: CPT updates , prolonged care
CPT®️️ is a registered trademark of the American Medical Association. Copyright American Medical Association. All rights reserved.
All content on CodingIntel is copyright protected. Any resource shared within the permissions granted here may not be altered in any way, and should retain all copyright information and logos.
- What is CodingIntel
- Terms of Use
- Privacy Policy
Our mission is to provide up-to-date, simplified, citation driven resources that empower our members to gain confidence and authority in their coding role.
In 1988, CodingIntel.com founder Betsy Nicoletti started a Medical Services Organization for a rural hospital, supporting physician practice. She has been a self-employed consultant since 1998. She estimates that in the last 20 years her audience members number over 28,400 at in person events and webinars. She has had 2,500 meetings with clinical providers and reviewed over 43,000 medical notes. She knows what questions need answers and developed this resource to answer those questions.
Copyright © 2024, CodingIntel A division of Medical Practice Consulting, LLC Privacy Policy

- For Ophthalmologists
- For Practice Management
- For Clinical Teams
- For Public & Patients
Museum of the Eye
- Latest Issue
- Back Issues (Nov. 2012 to present)
- Archive Highlights (pre Oct. 2012)
- Dr. Richard Mills' Opinions, 2002 to 2016
- Write For Us
- Corporate Webinars
- EyeNet Magazine
- / January 2023
- / E/M 2023—Starting Jan. 1, Streamlined Rules Apply Beyond the Office Setting
E/M 2023—Starting Jan. 1, Streamlined Rules Apply Beyond the Office Setting
- Mark Complete
Download PDF
Jan. 1, 2023, saw big changes to E/M coding. In a range of settings, use of E/M codes has become much less of a headache.
Streamlined Requirements Are No Longer Just for the Office
In 2021, it started with the office-based E/M codes. In 2021, CMS streamlined its documentation guidelines for the office-based E/M codes (CPT codes 99202-99215). Before that year, you had to obtain and document a review of body systems plus a past medical history, family history, and social history, plus a chief complaint, plus elements of the history of the present illness. Additionally, examination elements were required for specific levels of E/M codes. Since 2021, documentation of office-based E/M services has required only a medically relevant patient history and examination. This means that the chart only needs to document the information that will be medically relevant for the physician, and this will vary depending on the nature of the patient encounter.
The streamlined rules now apply to more E/M services. The 2021 changes for office-based E/M services are now being applied to the following categories of E/M services and their related families of codes:
- Hospital inpatient and observation care services
- Consultations
- Emergency department (ED) services
- Nursing facility services
- Home or residence services
Determine the appropriate level of E/M code. As with the office-based E/M codes, when using E/M codes in the above places of service you should perform and document a medically appropriate history and examination. The appropriate level of E/M code is determined by the following:
- The level of medical decision making (MDM) or
- The total time that the physician spends caring for the patient—including before, during, and after the face-to-face encounter—on the date of the visit.
The ED exception. Levels of service for the ED E/M codes (CPT codes 99281-99285) cannot be determined by total physician time. This is because, according to the AMA, “emergency department services are typically provided on a variable intensity basis, often involving multiple encounters with several patients over an extended period of time.” 1
99281—ED E/M Code Revised
Prior to 2023, CPT code 99281 Emergency department visit for the evaluation of a patient was selected when documentation supported a problem-focused history and exam and straightforward MDM. These requirements have now been removed and the descriptor updated to: Emergency department visit for the evaluation of a patient that may not require the presence of a physician or other qualified health care professional .
E/M code 99281 (like its office-based equivalent, 99211) is for a service provided by clinical staff under the supervision of a physician. For both codes, the concept of MDM does not apply. In an ED, 99281 will rarely—if ever—be used for ophthalmology.
MDM Table Updated for Extra Places of Service
The overall complexity level of MDM can be straightforward or of low, moderate, or high complexity. You determine the overall level of MDM by looking at three decision-making components: 1) problems, 2) data, and 3) risk. If at least two components point to the same level of MDM, then that would be the overall level of MDM. If the three components each point to a different level, then the middle one determines the overall level of MDM.
Since 2021, when practices have coded for an office-based encounter, they have used the Final Determination Table for MDM to determine which level of complexity those three components indicate. Now that MDM is to be used to determine E/M level in other places of service, there is a new Final Determination Table that includes examples from nonoffice settings. The Academy’s 2023 Final Determination Tables can be downloaded at aao.org/em for both office and hospital settings.
Prolonged Service Codes
This table demonstrates how the prolonged service codes 99417 and 99418 can be used in the office and inpatient settings, respectively.
2023 E/M Overview: Office Visits, Inpatient Hospital, and ED
When using time to determine level of E/M code, you must meet or exceed defined times, as shown below.
99418—New Add-On Code for Prolonged Services
Inpatient hospital care. When you are providing inpatient hospital care and using time as the factor for determining the level of E/M code, there could be scenarios that exceed the time designated for the highest level of service. If this is the case, prolonged services should be reported. As of Jan. 1, 2023, you can use CPT code 99418 to report for each additional 15 minutes. This code replaced codes 99354-99357, which were deleted on Dec. 31, 2022. It is an inpatient counterpart to 99417, which has been in use since 2021 for office-based E/M services.
When to use 99418. Only use this new add-on code if 1) time is the determining factor and 2) the total time is at least 15 minutes greater than the time allotted for the highest level of service in that setting. For example, if you are using time to determine the level of E/M for a subsequent hospital inpatient visit, you would need a time of at least 50 minutes to bill 99233, which is the highest level of E/M for that service. If the total time was 65-79 minutes, you could bill 99233 + 99418; if 80-94 minutes, bill 99233 + 99418 × 2; and for every additional 15 minutes, you can bill an extra 99418.
Note: Prolonged services of less than 15 minutes are not reported
Office Consultations
Medicare Part B does not cover consultation codes. However, there are a few remaining commercial and/or Medicaid payers that do. If one of your payers does so, report CPT codes 99242-99245 for consultations performed in the office or other outpatient place of service. As of Jan. 1, 2023, this family of codes had descriptor changes and—as mentioned above—are now selected based on MDM or total physician time. Furthermore, CPT code 99241 was deleted on Jan. 1. The lowest level of MDM, straightforward, is designated as CPT code 99242.
Reminder—How to Code for Inpatient Consultations
Since 2010, you must report initial inpatient consultations with CPT codes 99221-99223 when submitting to Medicare Part B. For subsequent consultations, report CPT codes 99231-99233. These codes should be reported instead of CPT codes 99251-99255 for payers not recognizing consultations. As a result, multiple claims for inpatient hospital visits can occur on one date of service by different providers. To distinguish between the principal physician of record (the admitting physician) and consultants, the former should append modifier –AI to the appropriate level of E/M. All other physicians (consultants) should submit the E/M code without modifier –AI.
___________________________
Coding resources. For more information, visit aao.org/em . You also can order 2023 Fundamentals of Ophthalmic Coding at aao.org/store .
1 www.ama-assn.org/system/files/2023-e-m-descriptors-guidelines.pdf . Accessed Nov. 3, 2022.
All content on the Academy’s website is protected by copyright law and the Terms of Service . This content may not be reproduced, copied, or put into any artificial intelligence program, including large language and generative AI models, without permission from the Academy.
- About the Academy
- Jobs at the Academy
- Financial Relationships with Industry
- Medical Disclaimer
- Privacy Policy
- Terms of Service
- Statement on Artificial Intelligence
- For Advertisers
- Ophthalmology Job Center
FOLLOW THE ACADEMY
Medical Professionals
Public & Patients

An official website of the United States government
The .gov means it’s official. Federal government websites often end in .gov or .mil. Before sharing sensitive information, make sure you’re on a federal government site.
The site is secure. The https:// ensures that you are connecting to the official website and that any information you provide is encrypted and transmitted securely.
- Publications
- Account settings
Preview improvements coming to the PMC website in October 2024. Learn More or Try it out now .
- Advanced Search
- Journal List

Length-of-Stay in the Emergency Department and In-Hospital Mortality: A Systematic Review and Meta-Analysis
Dominique lauque.
1 Department of Emergency Medicine, Purpan Hospital and Toulouse III University, 31300 Toulouse, France
2 Department of Emergency of Medicine, Beth Israel Deaconess Medical Center, Teaching Hospital of Harvard Medical School, Boston, MA 02115, USA
Anna Khalemsky
3 Management Department, Hadassah Academic College, Jerusalem 91010, Israel
Zoubir Boudi
4 Department of Emergency Medicine, Dr Sulaiman Alhabib Hospital, Dubai 2542, United Arab Emirates
5 Global Network on Emergency Medicine, Brookline, MA 02446, USA
Linda Östlundh
6 Örebro University Library, Örebro University, 70182 Örebro, Sweden
7 Ministry of Education, Key Laboratory for Population Health Across-Life Cycle, Anhui Medical University, Hefei 230032, China
Mohammed Alsabri
8 Department of Pediatrics, Brookdale University Hospital and Medical Center, 1 Brookdale Plaza, Brooklyn, NY 11212, USA
Churchill Onyeji
Jacqueline cellini.
9 Countway Library, Harvard Medical School, Boston, MA 02115, USA
10 MATHEMATICA, Inc., Princeton, NJ 08540, USA
Geroge Intas
11 Department of Critical Care, General Hospital of Nikaia Agios Panteleimon, 18454 Athens, Greece
Kapil Dev Soni
12 Jai Prakash Narayan Apex Trauma Center, Ring Road, New Delhi 110029, India
Detajin Junhasavasdikul
13 Department of Medicine, Faculty of Medicine Ramathibodi Hospital, Mahidol University, Bangkok 10400, Thailand
Jose Javier Trujillano Cabello
14 Intensive Care Unit, Hospital Universitari Arnau de Vilanova, 25198 Lleida, Spain
Niels K. Rathlev
15 Department of Emergency Medicine, University of Massachusetts Medical School, Baystate, Springfield, MA 01199, USA
Shan W. Liu
16 Department of Emergency Medicine, Massachusetts General Hospital, Harvard Medical School, Boston, MA 02114, USA
Carlos A. Camargo, Jr.
Anna slagman.
17 Division of Emergency and Acute Medicine, Campus Virchow Klinikum and Charité Campus Mitte, Charité Universitätsmedizin, 10117 Berlin, Germany
Michael Christ
18 Department of Emergency Medicine, 6000 Lucerne, Switzerland
Adam J. Singer
19 Department of Emergency Medicine, Renaissance Scholl of Medicine at Stony Brook University, Stony Brook, NY 11794, USA
Charles-Henri Houze-Cerfon
Elhadi h. aburawi.
20 Department of Pediatrics, College of Medicine and Health Sciences, UAE University, Al Ain P.O. BOX 15551, United Arab Emirates
Karim Tazarourte
21 Department of Health Quality, University Hospital, Hospices Civils, 69002 Lyon, France
22 Department of Emergency Medicine, University Hospital, Hospices Civils, 69002 Lyon, France
Lisa Kurland
23 Department of Medical Sciences, Örebro University, 70182 Örebro, Sweden
Phillip D. Levy
24 Department of Emergency Medicine, Wayne State University School of Medicine, Detroit, MI 48201, USA
James H. Paxton
Dionyssios tsilimingras.
25 Department of Family Medicine & Public Health Sciences, Wayne State University School of Medicine, Detroit, MI 48201, USA
Vijaya Arun Kumar
David g. schwartz.
26 Information Systems Department, Graduate School of Business Administration, Bar-Ilan University, Ramat-Gan 529002, Israel
27 Department of Emergency Medicine, Emergency Medicine Cumming School of Medicine, University of Calgary, Alberta Health Services, Calgary, AB T2N 1N4, Canada
David W. Bates
28 Department of Healthcare Quality, Brigham and Women Hospital, Harvard Medical School, Boston, MA 02115, USA
Gabriele Savioli
29 Emergency Department, IRCCS Fondazione Policlinico San Matteo, 27100 Pavia, Italy
Shamai A. Grossman
Abdelouahab bellou.
30 Institute of Sciences in Emergency Medicine, Department of Emergency Medicine, Guangdong Provincial People’s Hospital, Guangdong Academy of Medical Sciences, Guangzhou 510080, China
Associated Data
Template data collection forms, data extracted from included studies, data used for all analyses, analytic code, and any other materials used in the review are available from the corresponding author upon request. We also utilized de-identified data pertaining to EDLOS cut-offs and mortality that did not require ethical committee approval.
The effect of emergency department (ED) length of stay (EDLOS) on in-hospital mortality (IHM) remains unclear. The aim of this systematic review and meta-analysis was to determine the association between EDLOS and IHM. We searched the PubMed, Medline, Embase, Web of Science, Cochrane Controlled Register of Trials, CINAHL, PsycInfo, and Scopus databases from their inception until 14–15 January 2022. We included studies reporting the association between EDLOS and IHM. A total of 11,337 references were identified, and 52 studies (total of 1,718,518 ED patients) were included in the systematic review and 33 in the meta-analysis. A statistically significant association between EDLOS and IHM was observed for EDLOS over 24 h in patients admitted to an intensive care unit (ICU) (OR = 1.396, 95% confidence interval [CI]: 1.147 to 1.701; p < 0.001, I 2 = 0%) and for low EDLOS in non-ICU-admitted patients (OR = 0.583, 95% CI: 0.453 to 0.745; p < 0.001, I 2 = 0%). No associations were detected for the other cut-offs. Our findings suggest that there is an association between IHM low EDLOS and EDLOS exceeding 24 h and IHM. Long stays in the ED should not be allowed and special attention should be given to patients admitted after a short stay in the ED.
1. Introduction
Prolonged length of stay (LOS) in the emergency department (ED), characterized by an inappropriately long period before final departure for an in-hospital bed, home, or another facility, is believed to adversely affect clinical outcomes. The time spent in the ED can be divided into distinct periods that are marked by time of arrival (triage registration), time of the start of care, time of the disposition decision (discharge or admission), time at the end of care, and time at ED departure ( Figure 1 ). EDLOS is defined as the time elapsed between the initial triage registration and physical departure from the ED. Boarding time (BT), defined as the time spent waiting for inpatient bed availability after the decision to admit the patient is made, is a significant contributor to the LOS. BT may also affect outcomes, as boarded patients require ongoing, often intensive care that several EDs are not well equipped to deliver [ 1 , 2 , 3 ]. The definition of prolonged EDLOS may vary. Prolonged ED visits have been defined as >4 h in the United Kingdom, >6 h in Canada and the U.S., and >8 h in Australia [ 4 , 5 , 6 ].

Definition of time spent in the emergency department related to each segment of the care process.
Prior studies have shown that ED boarding delays care, including the commencement of home medication, and increases the risk of adverse events, prolongs in-hospital LOS, and is associated with staff and patient dissatisfaction [ 7 , 8 , 9 , 10 ]. Prolonged ED BT also consumes already scarce ED resources, making them unavailable for the care of new patients and potentially affecting the outcomes of non-boarded patients [ 1 , 11 ].
Despite increased recognition of the adverse effects of prolonged EDLOS, its effect on patient mortality remains unclear. Several studies have found that ED crowding and increased BT are associated with higher mortality rates [ 11 , 12 , 13 , 14 , 15 , 16 ].
Crowding can increase both EDLOS and BT, since the rate of patient intake exceeds the capacity of the triage process. Throughput is also overwhelmed, because the number of patients requiring managing is high, and a lack of hospital beds throttles patient outflow [ 17 ]. Although there is a significant relationship between crowding, boarding time, and EDLOS, the relationship with in-hospital mortality (IHM) remains unclear.
Given the lack of evidence, additional research is needed to explore the association between EDLOS and IHM. This is important, considering recent evidence demonstrating the limited implementation and thus limited impact of hospital strategies to improve patient flow through the ED [ 1 , 2 , 17 , 18 ].
To address this knowledge gap, we performed a systematic review and meta-analysis (MA) which examined the association between EDLOS and IHM. We hypothesized that a longer EDLOS would predict greater IHM risk.
2. Materials and Methods
This systematic review and MA focused on studies analyzing the relationship between total EDLOS and IHM. Studies analyzing only the BT, which represents a time segment within the EDLOS (see Figure 1 ), and overcrowding studies that did not refer to the EDLOS were excluded.
The review follows the 2020 Preferred Reporting Items for Systematic Reviews and Meta-Analyses (PRISMA) guidelines recommended by the Cochrane Handbook for Systematic Reviews of Interventions [ 19 ]. A PRISMA checklist is presented in Supplemental Table S1 . The protocol for this review was registered in PROSPERO, CRD42016050422 ( http://www.crd.york.ac.uk/PROSPERO , accessed on 29 November 2022).
2.1. Data Sources and Searches
We defined EDLOS as the time elapsed between the initial triage patient registration and physical departure from the ED ( Figure 1 ). Our primary endpoint was all-cause mortality.
A systematic search of the PubMed, Embase, Web of Science, Cochrane Controlled Register of Trials, CINAHL, PsycInfo, and Scopus databases was prepared by two medical librarians specializing in systematic reviews (L.Ö., J.C.), in close collaboration with D.L. and A.B. (emergency medicine expert physicians). All terms were searched in the fields for “Abstract” and “Article Title” (alternatively in the field for “Topic”) and MeSH/Subject Headings/Thesaurus when available. The databases were first searched from their inception to January 2020 (L.Ö.). A search update was conducted in the same databases during manuscript preparation on 14–15 January 2022 (L.Ö.), to ensure the inclusion of recently published papers. No filters or limitations were applied to retrieve the best possible results. We screened all published studies related to ED boarding and crowding to identify those reporting data on EDLOS and IHM. Studies reporting EDLOS cut-off times were included in the MA. Studies not mentioning EDLOS or IHM were excluded. We also screened the reference lists of the selected studies manually. The reviewers also manually searched the gray literature (including congress and meeting abstracts) but excluded these sources when they were not subsequently followed by full-text articles published in scientific journals. Reproducible search strings, results, and technical notes for each database are presented in Supplemental Table S2 .
2.2. Inclusion Criteria and Study Selection
All patients over 18 years old who visited an ED were included. Exposure was defined as the time spent in the ED from the arrival to the admission to inpatient bed. This time exposure was defined as a EDLOS cut-off chosen in selected studies. The outcome was IHM whatever the cause and the delay of death in the in-hospital bed was. We considered all studies based on a prospective or retrospective design, namely cohort studies, case-control studies, as well as randomized controlled trials.
Records identified in the literature search were uploaded to the Covidence (Veritas Health Innovation, 2021, https://www.covidence.org , accessed on 29 November 2022) systematic review software for blinded screening and automatic removal of duplicates. We extracted articles focused on the association between EDLOS and IHM in an adult ED setting. Studies analyzing the effects of boarding and ED crowding on mortality were also included when EDLOS was reported in their statistical analysis. Publications in English and other languages using translators when necessary were included.
Two emergency medicine specialists (D.L., A.B.) independently screened the titles and abstracts yielded by the literature searches. Any selection disagreements identified by Covidence were resolved by discussion to reach consensus or were adjudicated by a third independent reviewer (Z.B.). Full reports were obtained for all titles or abstracts that met the inclusion criteria. Both reviewers independently read all full-text articles, obtaining additional information from the study authors as needed to resolve questions about eligibility. An overview of the screening and selection process is presented in the PRISMA flow diagram ( Figure 2 ). Study data were extracted into a customized Microsoft Excel ® table, including the following study characteristics: design, setting, population, sample size, main objective, prognostic factors, and outcomes such as boarding, definition and values of EDLOS, crowding, type of mortality, results including precision and significance, and adjustment for confounding factors (e.g., age, comorbidities, diagnosis, triage severity code).

PRISMA 2020 flow diagram over the record de-duplication screening and selection process.
2.3. Data Extraction and Quality Assessment
The quality of each study was rated and recorded in a data collection form. Quality assessments were performed independently by two reviewers (A.B., Z.B.) using the Newcastle–Ottawa Quality Assessment Scale (NOS), a scale designed for non-randomized trials [ 20 ], and disagreements were resolved by discussion to reach consensus.
The NOS consists of four items on “study selection”, one item on “comparability”, and three items on “study outcome” [ 20 ]. Using this scale, reviewers can award one star for each of the four items on “selection”, one star for each of the three items on “outcome”, and a maximum of two stars for “comparability”. Ratings were calculated independently by each reviewer, and the results were averaged. Studies of the highest quality were awarded nine stars.
The risk of bias was summarized for each study and incorporated into the overall findings and data synthesis.
2.4. Data Synthesis and Analysis
The MA was performed using OpenMeta Analyst through (1) CEBM@Brown OpenMeta[Analyst] (Brown University, http://www.cebm.brown.edu/openmeta/ , access on 29 November 2022), (2) Cross-platform Excel package (MetaXL, www.epigear.com , EpiGear International Pty Ltd., Castaways Beach, Noosa Heads & Sunrise Beach, Queensland, Australia), and (3) MedCalc easy-to-use statistical software package (MedCalc Software Ltd., Acacialaan, Ostend, Belgium).
Odds ratios (ORs) were used to measure the potential association between EDLOS and IHM. For binary outcome variables, the measured effect was expressed as the log-transformed estimated OR. The weight of each study in the analysis was expressed as the inverse of the variance of the log-transformed estimated OR. The amount of between-study heterogeneity against the total variance was measured by I 2 and presented as 0–100%.
Sensitivity analysis was performed by the leave-one-out method, in which one study at a time was removed iteratively to confirm that our findings were not dictated by any specific study. With this method, if the results are consistent, there is confidence that the overall MA results are robust.
To illustrate the foundations, we used forest plots to summarize and visualize the effect size of each study, including 95% confidence intervals (CIs), with respect to the study’s weight. The location of the 95% CI for the OR in relation to 1, in the case of ORs, also indicated the significance of the effect size.
We used a DerSimonian–Laird random-effects model in our study. Because the weight of each study should be approximately the same, the weighted analysis for the random-effects model was treated as an unweighted analysis.
To examine the influence of population characteristics on overall heterogeneity, we separated the studies into two subsets for each cut-off: intensive care unit (ICU) and non-ICU population subsets. Two additional meta-analyses were conducted for each subset.
Moreover, to improve the accuracy of our heterogeneity evaluation in the MA, we used the IVhet model in the Microsoft Excel ® MA package, designed particularly for use in meta-analyses with high heterogeneity (MetaXL, available at www.epigear.com , accessed on 29 November 2022) [ 21 , 22 ]. This method uses the quasi-likelihood estimator as an alternative to random-effects models with the problem of underestimation of the statistical error and overconfident estimates. The estimator retains a correct coverage probability and a lower observed variance than the random-effect model estimator, regardless of heterogeneity [ 23 , 24 ].
The symmetry of a funnel plot and Egger and Begg tests were used to qualitatively determine the presence of publication bias (MedCalc Software, version 19.6.1) [ 21 , 22 ].
To analyze the factors underlying heterogeneity, we performed a univariate meta-regression analysis using the following factors: age, sex, country of study, ED population, and disease severity.
A total of 23,176 records were identified in the database search, with 11,337 references screened after the removal of duplicates. Two papers were added after the manual screening of the reference lists of the included papers. A search log with details and results from the search is provided in Supplemental Table S2 . After screening, 50 studies were selected for inclusion in the review ( Figure 2 , Table 1 and Table 2 ) [ 3 , 9 , 25 , 26 , 27 , 28 , 29 , 30 , 31 , 32 , 33 , 34 , 35 , 36 , 37 , 38 , 39 , 40 , 41 , 42 , 43 , 44 , 45 , 46 , 47 , 48 , 49 , 50 , 51 , 52 , 53 , 54 , 55 , 56 , 57 , 58 , 59 , 60 , 61 , 62 , 63 , 64 , 65 , 66 , 67 , 68 , 69 , 70 , 71 , 72 ]. Thirty-three of these were included in the MA ( Table 3 ) [ 25 , 26 , 27 , 28 , 29 , 30 , 31 , 32 , 33 , 34 , 35 , 36 , 38 , 39 , 40 , 41 , 42 , 43 , 44 , 45 , 46 , 47 , 48 , 49 , 50 , 57 , 58 , 59 , 60 , 61 , 63 , 68 , 71 ]. The remaining 17 studies [ 3 , 9 , 37 , 51 , 52 , 53 , 54 , 55 , 56 , 62 , 64 , 65 , 66 , 67 , 69 , 70 , 72 ] were excluded for one of the following reasons: (1) the specific EDLOS cut-off was not defined [ 3 , 9 , 37 , 51 , 52 , 53 , 54 , 56 , 62 , 64 , 65 , 66 , 67 , 69 , 72 ]; (2) one study reported data in severely ill mechanically ventilated patients [ 54 ]; and (3) raw data were missing in one study [ 70 ]. We subsequently acquired the original databases for four studies [ 34 , 41 , 42 , 45 ], which allowed us to perform analysis of nine different EDLOS cut-off values: 1.2 h [ 34 , 41 , 42 , 45 , 50 ], 1.5 h [ 34 , 40 , 41 , 42 , 45 ], 2 h [ 32 , 34 , 41 , 42 , 45 ], 3 h [ 34 , 41 , 42 , 45 , 47 , 68 ], 4 h [ 33 , 40 , 41 , 42 , 44 , 57 , 58 ], 5 h [ 32 , 34 , 38 , 42 , 46 ], 6 h [ 33 , 36 , 39 , 40 , 42 , 44 , 45 , 46 , 48 , 49 , 53 , 59 , 60 , 61 ], 8 h [ 25 , 26 , 27 , 28 , 29 , 30 , 31 , 34 , 41 , 42 , 45 ], 12 h [ 59 ], and 24 h [ 35 , 71 ].
Characteristics of the selected studies for the systematic review.
EDLOS, Emergency Department Length of Stay.
Factors analyzed and association between EDLOS and in-hospital mortality in the selected studies for the systematic review.
AIS, Abbreviated Injury Score; ACS, American College of Surgeons; APACH, Acute Physiology and Chronic Health Evaluation; CRRT, continuous renal replacement therapy; ED, emergency department; EDLOS, ED length of stay; GCS, Glasgow Coma Scale; ICU, intensive care unit; Injury Severity Score, ISS; In-Hospital Mortality, IHM; International Classification of Diseases, ICD; KTAS, Korean Triage and Acuity Scale; MAP, mean arterial blood pressure; MEWS, Modified Early Warning Score; MV, mechanical ventilation; NEWS, National Early Warning Score; NIH-SS, NIH Stroke Scale; RTS, Revised Trauma Score; SAPS, Simplified Acute Physiology Score II; TRISS, therapeutic intervention scoring system; SOFA, Sequential Organ Failure Assessment; TISSS, Therapeutic Intervention Scoring System Score.
Distribution of the studies that showed an association between EDLOS and IHM for each population and quality score of studies.
The average study quality score is 6.48 (max.: 8, min.: 3.5) for the ICU-admitted population and 6.45 (max.: 8, min.: 4) for the non-ICU population. The Newcastle–Ottawa Quality Assessment Scale was used to evaluate the quality of the studies [ 20 ]. ACS, American College of Surgeons; APACH, Acute Physiology and Chronic Health Evaluation; CRRT, continuous renal replacement therapy; ED, emergency department; EDLOS, emergency department length of stay; GCS, Glasgow Coma Scale; ICU, intensive care unit; IHM, in-hospital mortality; ISS, Injury Severity Score; MA, meta-analysis; MEWS, Modified Early Warning Score; MV, mechanical ventilation; SAPS, Simplified Acute Physiology Score II; SOFA, Sequential Organ Failure Assessment; SS, Stroke Severity; TISSS, Therapeutic Intervention Scoring System Score.
3.1. Systematic Review
3.1.1. characteristics of the selected studies.
The selected studies included a total of 1,027,838 ED visits. Eleven studies were conducted in Europe (Spain [ 34 , 38 ], Greece [ 45 ], the U.K. [ 37 , 49 ], Sweden [ 62 ], Finland [ 47 , 54 ], The Netherlands [ 50 ], France [ 64 ], Norway [ 65 ]), 15 in North America (USA [ 9 , 30 , 32 , 33 , 35 , 36 , 38 , 40 , 46 , 55 , 58 , 60 , 68 , 72 ], Canada [ 3 ]), 15 in Asia (Qatar [ 29 ], Saudi Arabia [ 61 ], China [ 31 , 44 , 55 , 66 ], Thailand [ 41 ], India [ 42 , 72 ], Pakistan [ 48 , 63 ], Israel [ 57 ], Iran [ 70 ], South Korea [ 59 ], Turkey [ 69 ]), 7 in Australia [ 25 , 26 , 27 , 28 , 43 , 51 , 67 ], and 2 in Latin America [ 52 , 56 ]. The characteristics of the selected studies are listed in Table 1 and Table 2 .
Two patient population types were identified across the selected studies: the non-ICU-admitted population, and the ICU-admitted population ( Table 3 ).
Thirty studies examined patients who were transferred from the ED to the ICU [ 3 , 25 , 32 , 34 , 35 , 36 , 38 , 39 , 40 , 42 , 44 , 45 , 47 , 48 , 50 , 51 , 52 , 53 , 54 , 55 , 56 , 58 , 59 , 60 , 61 , 63 , 67 , 69 , 70 , 71 ]. Twenty studies examined patients who were not admitted to the ICU, but were admitted to non-ICU wards [ 9 , 26 , 27 , 28 , 29 , 30 , 31 , 33 , 37 , 41 , 43 , 46 , 49 , 57 , 62 , 64 , 65 , 66 , 68 , 72 ].

3.1.2. Non-Intensive Care Unit-Admitted Population
The non-ICU-admitted ED population was defined as a population seen in the ED and admitted to a non-critical care inpatient hospital ward ( Table 3 ). Twenty studies analyzed the relationship between EDLOS and IHM in ED populations not admitted to the ICU [ 9 , 26 , 27 , 28 , 29 , 30 , 31 , 33 , 37 , 41 , 43 , 46 , 49 , 57 , 62 , 64 , 65 , 66 , 68 , 72 ]. Nine studies found an association with IHM when EDLOS exceeded a cut-off value [ 26 , 29 , 33 , 37 , 43 , 62 , 64 , 66 , 68 ], and 11 studies did not find an association [ 9 , 27 , 28 , 30 , 31 , 41 , 46 , 49 , 57 , 65 , 72 ]. Detailed information for each study is available in the Supplemental Text .
3.1.3. Intensive Care Unit-Admitted Emergency Department Population
The ICU-admitted ED population was defined as a population seen in the ED and admitted to ICU inpatient hospital ward ( Table 3 ). Thirty studies analyzed the association between EDLOS and IHM in ED patients admitted to the ICU [ 3 , 25 , 32 , 34 , 35 , 36 , 38 , 39 , 40 , 42 , 44 , 45 , 47 , 48 , 50 , 51 , 52 , 53 , 54 , 55 , 56 , 58 , 59 , 60 , 61 , 63 , 67 , 69 , 70 , 71 ]. Thirteen studies found an association between EDLOS and IHM [ 32 , 38 , 44 , 45 , 50 , 55 , 56 , 59 , 60 , 61 , 63 , 70 , 71 ], while 17 did not find such an association [ 3 , 25 , 34 , 35 , 36 , 39 , 40 , 42 , 47 , 48 , 51 , 52 , 53 , 54 , 58 , 67 , 69 ].
Detailed information is available in the Supplemental Text .
3.1.4. Quality of the Selected Studies
The methodological quality of the studies is presented in Supplemental Table S3 . The evaluation was performed by two independent evaluators. The average quality score was 6.53 ± 1.23 (min.: 3; max.: 8), which can be considered intermediate.
3.2. Meta-analysis
3.2.1. random-effects models.
The DerSimonian–Laird random-effects model [ 73 ] showed no statistically significant association between EDLOS and IHM, regardless of the cut-off value used: low EDLOS (1.2–3 h) (OR 0.954, 95% CI 0.685 to 1.330; p = 0.783, I 2 = 75.481%), 4 h (OR 0.958, 95% CI 0.455 to 2.018; p = 0.910, I 2 = 97.29%), 5 h (OR = 1.005, 95% CI 0.494 to 2.046; p = 0.989, I 2 = 81.16%), 6 h (OR = 0.952, 95% CI 0.690 to 1.315; p = 0.766, I 2 = 97.11%), 8 h (OR 1.064, 95% CI 0.838 to 1.352; p = 0.611, I 2 = 94.82%), or 24 h (OR 1.220, 95% CI 0.85 to 1.748; p = 0.279, I 2 = 45.58%) cut-off values ( Figure 3 , Supplemental Table S4 ).

Meta-analysis including studies with the same EDLOS (1.2 h, 1.5 h, 2h, 3 h cut-offs), 4 h, 5 h, 6 h, 8 h, and 24 h cut-offs using the DerSimonian–Laird random effects model). I^2 = I 2 . EDLOS, emergency department length of stay.
The ED populations included in these studies were divided into two categories: the patients admitted to the ICU (ICU-admitted population, representing the most critically ill patients) and those not admitted to the ICU (non-ICU-admitted population; those admitted to lesser-acuity in-patient wards). Our meta-analysis identified an association between EDLOS and IHM for the 24 h cut-off only in ED ICU-admitted patients, with a significant OR of 1.396 (95% CI 1.147 to 1.701, I 2 = 0%; p < 0.001). Another association was found for a low EDLOS cut-off in the non-ICU-admitted ED patients’ subgroup, with a significant OR of 0.581 (95% CI 0.453 to 0.745, I 2 = 0%; p < 0.001) ( Supplemental Figures S1 and S2 ).
No significant association was found between EDLOS and IHM for any of the cut-off values when all studies, including both ICU and non-ICU populations, were tested together. After dividing the patients into the two population types to create a certain level of homogeneity in each subgroup, the effect of prolonged EDLOS on IHM could be identified. For all cut-off values, the overall effect size was close to 1, and was not statistically significant, but in the ICU subgroup, the effect size was above 1 (significant for 24 h cut-off), and in the non-ICU subgroup, the effect size was less than 1 (significant for a low EDLOS cut-off).
3.2.2. Funnel Plots
We observed a publication bias ( Supplemental Figures S3–S5 ), as confirmed by Egger and Begg tests ( Supplemental Tables S5–S7 ).
3.2.3. Cross-validation (Leave-one-out)
The results of the cross-validation performed by the leave-one-out method are given in Supplemental Tables S8–S10 . This procedure was used in cases where insufficient data were available for partitioning between the training and test datasets. The sensitivity analysis confirmed the high heterogeneity among studies, which was not decreased by the exclusion of any single study ( Supplemental Table S8 ).
We performed a sensitivity analysis in both ICU and non-ICU populations. The observed heterogeneity remained high in both subpopulations ( Supplemental Tables S9 and S10 ). The exclusion of studies one by one, as suggested by Choi et al. [ 59 ], Intas et al. [ 45 ], Servia et al. [ 34 ], Soni et al. [ 42 ], Tilluckhdarry et al. [ 35 ], and Verma et al. [ 71 ], significantly reduced the heterogeneity in the ICU population for the 24 h cut-off value ( Supplemental Table S9 ). Sensitivity analysis for the non-ICU population was possible only for a cut-off of 4 h, with the exclusion of the Paton et al. study ( Supplemental Table S10 ) [ 44 ].
To summarize, for most cut-off values except for EDLOS <3 h and EDLOS >24 h, in the studies overall and in the ICU and non-ICU subgroups separately, no single study had a significant effect on the test results.
3.2.4. Inverse Variance Heterogeneity Model
Because of the high level of heterogeneity between studies, we decided to conduct a meta-analysis using the inverse variance heterogeneity (IVhet) model [ 23 , 24 ]. We did not find a significant difference in IHM between patients staying in the ED for any of the investigated cut-off periods ( Supplemental Table S11 ). The use of the IVhet model allows reducing the underestimation of the statistical error and overconfident estimates. In all cases, even if the 95% CI for the effect size of the random-effects model revealed a significant result, the IVhet model provided a broader 95% CI for the same effect size, so that eventually, none of the effect sizes were statistically significant. This finding supported the main conclusion that there is no significant association between EDLOS and IHM.
3.2.5. Subgroup Meta-Analyses and Univariate Meta-Regression Analysis
We performed different meta-analyses to isolate subpopulations to explain the observed high heterogeneity. First, we excluded step-by-step studies because we observed that this exclusion decreased heterogeneity. The random-effects model confirmed the absence of an association ( Supplemental Table S12 ). Next, we performed meta-analyses of studies that included the general ED population ( Supplemental Table S12 ), specific disease populations, and patients with different severities of illness (ICU and non-ICU populations) ( Supplemental Table S12 ). We found that the disease population and severity of illness were involved in the heterogeneity ( Supplemental Table S12 ).
To explain the source of heterogeneity, a univariate meta-regression analysis was performed for each cut-off value separately. As expected, some of the factors had a significant effect on heterogeneity. For example, in meta-regression analysis for the 6 h cut-off, all the factors were significant at a 5% significance level.
4. Discussion
EDLOS and BT are used by hospital administrators as measures of the quality of care delivered in the ED. A prolonged EDLOS is a source of dissatisfaction for patients and family; however, this indicator in isolation is not sufficient to comprehensively evaluate the quality of care. Combining ED time and the occurrence of negative outcomes, such as adverse events and IHM, is comparatively more relevant, and could help to improve quality of care. We previously found that there was a trend that BT increases IHM [ 74 ]. This new systematic review and MA did not find a significant relationship between EDLOS and IHM for any of the studied cut-off time points. However, our research did uncover a new and relevant result for EDLOS >24 h in ED ICU-admitted patients and EDLOS <3 h in non-ICU-admitted ED patients. For these cut-offs and types of ED populations, we did not find heterogeneity (I 2 = 0). The absence of a statistically significant difference in IHM for the other cut-offs is likely multifactorial, including the heterogeneity among the studies and various other factors, including population characteristics (e.g., age, sex, triage severity score, type of disease, mode of arrival at the ED, ED daytime, time shift, etc.), variation in hospital organization, adherence to clinical guidelines, type of admission source, and other factors. We used IVhet, designed particularly for use in meta-analyses with high heterogeneity, to provide better validation for the same estimated effect size [ 23 , 24 ]. Regular random-effects models, such as inverse variance or DerSimonian–Laird [ 73 , 75 , 76 , 77 ], emphasize the need for larger studies and indicate an underestimation of the statistical error. However, the IVhet model provides the correct coverage of the estimated effect size. The CI of the effect size obtained with this model was wider than that in other random-effects models. All 95% CIs using the IVhet model included 1; thus, we can conclude that there was no significant association between EDLOS and IHM for cut-off values of 4–8 h, which represent the target times in some countries [ 4 , 5 , 6 ]. Cross-validation analysis did not reduce the heterogeneity ( Supplemental Table S11 ). However, meta-regression analysis showed that factors, such as type of population, type of disease, and severity of illness, could explain the heterogeneity for EDLOS <3 h, 4 h, 5 h, and 6 h cut-off values ( Supplemental Table S12 ). Most categorical variables (e.g., population type, severity score, and country) were found to be significant in at least some of the meta-regression models at different cut-offs. However, there was no consistent impact of one variable on all cut-offs.
In exploring this lack of association between EDLOS and IHM for some cut-offs, we recognize that processing time and patient care time are complex variables, combining many different factors that influence the EDLOS, quality of care, and patient safety in the ED [ 78 , 79 ]. Given the frenzied nature of the ED environment, crowding may prevent providers from giving critically ill patients the close and constant attention they need [ 80 , 81 , 82 , 83 , 84 , 85 , 86 , 87 , 88 , 89 , 90 , 91 , 92 ]. This could be expected to lead to worse outcomes for patients, including increased IHM, but the evidence that we found in this systematic review was mixed. While some studies suggested that EDLOS is an independent predictor of ICU mortality [ 3 , 25 , 34 , 35 , 36 , 39 , 40 , 42 , 47 , 48 , 51 , 52 , 53 , 54 , 58 , 67 , 71 ], others reported no adverse association [ 32 , 38 , 44 , 45 , 50 , 55 , 56 , 59 , 60 , 61 , 63 , 69 , 70 ]. MA of the studies reporting IHM in patients admitted to the ICU showed an association with EDLOS over 24 h, with absence of heterogeneity. In most EDs, it is only acceptable to keep critical patients in the ED when there are no ICU beds. Many EDs are not designed to manage those patients optimally, due to a lack of trained emergency specialists in some countries, a lack of nurse resources, or the absence of a specific intensive care area where critical patients can be safely observed by a specific team. In ED patients who were admitted to non-ICU wards, some studies showed an association between EDLOS and IHM [ 26 , 29 , 33 , 37 , 43 , 62 , 64 , 66 , 68 ], while other studies did not [ 9 , 27 , 28 , 30 , 31 , 41 , 46 , 49 , 57 , 65 , 72 ]. Surprisingly, with cut-off values analyzed through different random-effects meta-analyses, we found a significant association between EDLOS < 3 h and IHM. Our data do not provide an explanation of this finding, and prospective studies analyzing all factors that contribute to the EDLOS are needed. It is typically rare to admit ED patients within 3 h, which is often below the threshold for obtaining all laboratory and imaging results, and for some patients’ specialist consultations. Sicker patients and those with clear-cut diagnoses who receive certain specific treatments may account for early departures, which could explain this result.
Our recommendations for policy makers are that long stays in the ED must be discouraged, unless there is a specific track for these patients including a specific ED area with a dedicated team. Another lesson from our study is that ED teams must be careful when they admit patients after a short stay in the ED and should be sure that there is continued close monitoring to avoid the risk of clinical deterioration. This may be particularly true in older patients where clinical presentations are often atypical. Some critically ill patients will be treated in the ED [ 58 ]. In such cases, the outcome and EDLOS will be dependent on the rapidity to stabilize the patients and the decision to admit them to hospital [ 57 ].
Another recommendation is to fast-track the care of specific events, such as myocardial infarction or stroke, that will be directly addressed to the angiography laboratory and acute neurovascular unit, resulting in a markedly reduced EDLOS for these patients. The association between EDLOS at different cut-off time points and IHM at different hospital time points (24 h, 48 h, 72 h, 7 days, 28 days), including the occurrence of adverse events after the ED care, could be worth investigating.
In contrast to high priority/sicker patients, mortality in patients with lower triage could be positively associated with EDLOS. One explanation could be the effect of under-triaging, where patients with medical urgency remain undetected by the ED triaging system. Patients with non-specific symptoms and low clinical urgency often have increased hospitalization, increased EDLOS, increased mortality, and more often are frail and of advanced age [ 9 , 93 ].
4.1. Study Strengths
The strengths of this MA include an extensive comprehensive search strategy, strong eligibility criteria that enhance generalizability, rigorous use of the NOS approach for rating the quality of evidence, a robust step-by-step MA, and a large number of included studies. This is the first MA exploring the association between EDLOS and IHM.
4.2. Study Limitations
Our study had some limitations and potential presence of publication bias. Some studies included a univariate analysis, while others used multivariate analysis, making it difficult to compare the effect sizes. Therefore, we chose to use an overall univariate analysis using the crude data reported by the studies. We used various meta-analyses and used the IVhet method to confirm the absence of an association for the classical cut-offs observed in the EDs. Moreover, a dose–response meta-analysis model was inapplicable for the relationship of EDLOS and IHM, given the lack of sufficient EDLOS-specific comparisons within each included study (i.e., only two-time ranges in each study) [ 94 ]; a dose–response model would be useful to determine the golden time range of EDLOS for patients needing emergency healthcare, and meanwhile explain the heterogeneity of the results. In addition, our study explored the association between EDLOS and IHM, but did not address the causes of prolonged EDLOS. With 33 studies from 50 countries worldwide included in the systematic review, we believe the results are generalizable to larger, urban, academic EDs, which represent the vast majority of EDs contributing to this MA. Representation of smaller, rural, non-academic EDs is limited, and therefore, generalizability to these EDs is unclear. More studies are needed to evaluate the correlation between EDLOS and IHM in different countries and hospital types, with variable equipment and human resources to confirm the results for EDLOS <3 h and EDLOS >24 h. In addition, a better understanding of the role played by potential confounding factors can help to reduce heterogeneity for the other cut-offs.
5. Conclusions
This MA was designed to analyze the association between EDLOS and IHM; we did not find evidence supporting this hypothesis when all ED patients were included for each cut-off. However, we did find a new and relevant result confirming an association with EDLOS and IHM for patients exceeding 24 h in ED ICU-admitted patients and for low EDLOS below 3 h in non-ICU-admitted ED patients. Other factors involved in the negative outcomes after ED care should be carefully explored to determine the role of EDLOS in the occurrence of IHM.
Acknowledgments
We thank Detajin Junhasavasdikul, Jose Javier Trujillano Cabello, Georges Intas, and Kapil Dev Soni for sharing their databases with approval by their ethical committees. We would also like to thank Mariam Al Ahbabi at the UAEU National Medical Library for her help with ordering and uploading full-text papers to the screening module in Covidence.
Abbreviations
Supplementary materials.
The following supporting information can be downloaded at: https://www.mdpi.com/article/10.3390/jcm12010032/s1 , Supplemental Text. Detailed information for each selected study; Figure S1. Meta-analysis of studies including the ICU-admitted ED population with the same cut-off using the random-effects model (DerSimonian–Laird), I^2 = I 2 ; Figure S2. Meta-analysis of studies including the non-ICU-admitted ED population with the same cut-off using the random-effects model (DerSimonian–Laird), I^2 = I 2 ; Figure S3. Funnel plots for all studies for each cut-off. (A) Low EDLOS (1.2 h, 1.5 h, 2 h, 3 h), (B) 4h cutoff, (C) 5h cut-off, (D) 6h cut-off, (E) 8h cut-off, (F) 24h cut-off; Figure S4. Funnel plots for ICU-admitted population. (A) Low EDLOS (1.2 h, 1.5 h, 2 h, 3 h), (B) 4 h cut-off, (C) 5 h cut-off, (D) 6 h cut-off, (E) 8 h cut-off, (F) 24 h cut-off; Figure S5. Funnel plots for non-ICU-admitted population. (A) 4 h cut-off, (B) 4 h cut-off, (C) 6 h cut-off, (D) 8 h cut-off. The remaining groups include 1–2 studies only; Table S1 [ 95 ]. PRISMA 2009 checklist for the systematic review and meta-analysis; Table S2. Search specifications for PubMed, Medline, Embase, Web of Science, Cochrane Library, CINAHL, APA PsycInfo, and Scopus; Table S3. Quality assessment of the studies. The Newcastle–Ottawa Quality Assessment Scale consists of 4 items on study selection, 1 item on comparability, and 3 items on study outcomes (see Reference 20 in main manuscript). According to this scale, studies can be awarded one star for each of the 4 items on selection and for each of the 3 items on outcomes, and a maximum of 2 stars for comparability. The highest quality studies are awarded up to nine stars. The evaluation was performed by two independent evaluators (E1 and E2). The average is 6.53 ± 1.23 (min.: 3; max.: 8); Table S4. Summary of the random-effects model results (DerSimonian–Laird); Table S5. Publication bias tests for all cut-offs; Table S6. Publication bias tests for all cut-offs in the ICU population; Table S7. Publication bias tests for all cut-offs in the non-ICU population; Table S8. Sensitivity analysis (leave-one-out) results of the overall data. Random-effects model (DerSimonian–Laird); Table S9. Sensitivity analysis (leave-one-out) results of the ICU population. Random-effects model (DerSimonian–Laird); Table S10. Sensitivity analysis (leave-one-out) results of the non-ICU population. Random-effects model (DerSimonian–Laird); Table S11. Heterogeneity analysis: comparison between inverse variance (IV) and inverse variance heterogeneity (IVhet) methods. Estimated effect size and CI (95%); Table S12: Meta-regression analysis. Discrete (categorical) factors are population type, country, and number of patients included in the studies. The continuous factors are age and sex. Discrete (categorical) factors = population type, severity (ICU/not ICU), country, disease. Continuous factors = age and % male.
Funding Statement
This research received no external funding.
Author Contributions
All authors made a significant contribution to the scientific quality of this paper and fulfilled the ICMJE criteria. All authors have approved the final version of this manuscript and have agreed to be held accountable for all aspects of the submitted work, including ensuring that any questions related to the accuracy or integrity of any part of the work are appropriately investigated and resolved. D.L., A.K., Z.B. and A.B. are considered co-first authors as they wrote the protocol, participated in the screening and selection of the studies, and drafted the manuscript. A.K. gathered the data, performed the MA, and analyzed and interpreted the results. L.Ö. developed and performed the search based on search terms selected in collaboration with A.B. and participated in the revision of the paper. J.C. performed the first search and participated in the revision of the paper. C.X., C.O., M.A., G.I., K.D.S., D.J., J.J.T.C., N.K.R., S.W.L., C.A.C., A.S., M.C., A.J.S., C.-H.H.-C., E.H.A., K.T., L.K., P.D.L., J.H.P., D.T., V.A.K., D.G.S., E.L., D.W.B., G.S. and S.A.G. participated in the revision of the paper. A.B. served as the principal investigator, proposed the project, participated in the protocol writing and screening and selection of the studies, and co-wrote and revised the draft manuscript with D.L., A.K. and Z.B. A.B. act as a senior and principal corresponding author, D.L and S.A.G. act as co-senior and co-corresponding. All authors have read and agreed to the published version of the manuscript.
Institutional Review Board Statement
We utilized de-identified data pertaining to EDLOS cut-offs and mortality that did not require ethical committee approval. This is a systematic review and meta-analysis of peer reviewed studies; each study had its own ethics approval.
Informed Consent Statement
Not applicable.
Data Availability Statement
Conflicts of interest.
The authors declare no conflict of interest.
Disclaimer/Publisher’s Note: The statements, opinions and data contained in all publications are solely those of the individual author(s) and contributor(s) and not of MDPI and/or the editor(s). MDPI and/or the editor(s) disclaim responsibility for any injury to people or property resulting from any ideas, methods, instructions or products referred to in the content.
Extended ED Mental Health Care Now Reportable as Observation
One in eight visits to the emergency department is related to a mental health or substance abuse issue. That number has grown each year over the past decade. 1 An informal poll of ACEP members showed that 100 percent of those asked had at least one behavioral health patient remaining in their emergency department for a multiple day stay in the past week.
You Might Also Like
- Coding for Behavioral Health Patients With Extended Stays
- Mental Health Care Across the Age Spectrum
- New CMS Rules Introduce Bundled Payments for Observation Care
Appropriate treatment for this vulnerable population is a high priority. An often loud and hectic ED setting is arguably one of the worst environments for a patient under mental stress. ACEP has long advocated for better access to facilities designed to care for behavioral health patients. The Improving Mental Health Access from the Emergency Department Act (H.R. 2519) was pushed forward by ACEP earlier this summer. This ACEP-drafted legislation would provide additional resources for patients with acute mental health needs who seek care in the ED due to a critical shortage of inpatient and outpatient resources. Working in parallel, the ACEP Coding and Reimbursement teams have been hard at work fighting for funds to care for these patients during their prolonged ED stays.
We all know that resources follow revenue. Historically, there was no approved way to capture the subsequent days of care provided after the initial ED visit, which was typically reported using the appropriate ED evaluation and management (E/M) code (99281–99285). As a result, long, multi-day mental health stays created both uncompensated care issues and further strained the safety net provided by our nation’s emergency departments.
Emergency providers faithfully support the safety net for our most vulnerable patients. We now have a mechanism and clear guidance to bill for multiday mental health stays.—Michael Granovsky, MD, FACEP
Due to the escalating mental health crisis, ACEP’s current procedural terminology (CPT) team has worked through the American Medical Association (AMA) CPT Editorial Panel and CPT Assistant , the monthly AMA publication that offers guidance related to correct use of the CPT code set, to seek a solution for this problem.
ACEP submitted a typical behavioral health vignette to CPT staff illustrating a patient who had presented with a behavioral health crisis who also required medical care while waiting to find an available inpatient psychiatric bed. The patient described presents with agitation, is severely decompensated, and has auditory hallucinations. The documented medical history reveals he has been off his medication for two weeks and has a prior suicide attempt. He has a history of diabetes and his glucose level is elevated. The plan is for a psychiatric admission, but no psychiatric beds are available at this hospital, and the patient remains in the emergency department for three days until he can be transferred. The patient remains under the care of the ED group for daily rounds, medication adjustments, and the management of any threats to the patient or hospital staff. On the third day, available space is found, and the patient is transferred to the psychiatric facility. Traditionally, coding guidance had been that “psych holding patients” did not meet the requirements to report observation services.
Pages: 1 2 3 | Single Page
Topics: Billing and Coding Medicare Psychiatric Boarding Reimbursement

Medicare’s Reimbursement Updates for 2024

What Is ACEP Fighting for in 2023?

Keep Safe While Boarding Psychiatric Patients in the Emergency Department
Current issue.
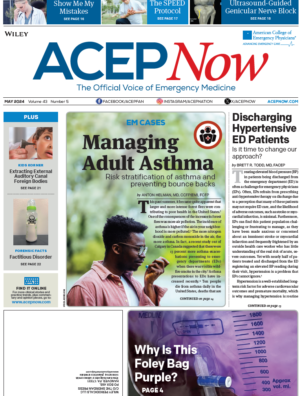
ACEP Now: Vol 43 – No 05 – May 2024
Download PDF
No Responses to “Extended ED Mental Health Care Now Reportable as Observation”
Leave a Reply Cancel Reply
Your email address will not be published. Required fields are marked *

Disclaimer » Advertising
- HealthyChildren.org

- Next Article
Study Design and Data Source
Study sample, conclusions, acknowledgment, prolonged emergency department length of stay for us pediatric mental health visits (2005–2015).
POTENTIAL CONFLICT OF INTEREST: The authors have indicated they have no potential conflicts of interest to disclose.
FINANCIAL DISCLOSURE: The authors have indicated they have no financial relationships relevant to this article to disclose.
- Split-Screen
- Article contents
- Figures & tables
- Supplementary Data
- Peer Review
- CME Quiz Close Quiz
- Open the PDF for in another window
- Get Permissions
- Cite Icon Cite
- Search Site
Katherine A. Nash , Bonnie T. Zima , Craig Rothenberg , Jennifer Hoffmann , Claudia Moreno , Marjorie S. Rosenthal , Arjun Venkatesh; Prolonged Emergency Department Length of Stay for US Pediatric Mental Health Visits (2005–2015). Pediatrics May 2021; 147 (5): e2020030692. 10.1542/peds.2020-030692
Download citation file:
- Ris (Zotero)
- Reference Manager
Children seeking care in the emergency department (ED) for mental health conditions are at risk for prolonged length of stay (LOS). A more contemporary description of trends and visit characteristics associated with prolonged ED LOS at the national level is lacking in the literature. Our objectives were to (1) compare LOS trends for pediatric mental health versus non–mental health ED visits and (2) explore patient-level characteristics associated with prolonged LOS for mental health ED visits.
We conducted an observational analysis of ED visits among children 6 to 17 years of age using the National Hospital Ambulatory Medical Care Survey (2005–2015). We assessed trends in rates of prolonged LOS and the association between prolonged LOS and demographic and clinical characteristics (race and ethnicity, payer type, and presence of a concurrent physical health diagnosis) using descriptive statistics and survey-weighted logistic regression.
From 2005 to 2015, rates of prolonged LOS for pediatric mental health ED visits increased over time from 16.3% to 24.6% (LOS >6 hours) and 5.3% to 12.7% (LOS >12 hours), in contrast to non–mental health visits for which LOS remained stable. For mental health visits, Hispanic ethnicity was associated with an almost threefold odds of LOS >12 hours (odds ratio 2.74; 95% confidence interval 1.69–4.44); there was no difference in LOS by payer type.
The substantial rise in prolonged LOS for mental health ED visits and disparity for Hispanic children suggest worsening and inequitable access to definitive pediatric mental health care. Policy makers and health systems should work to provide equitable and timely access to pediatric mental health care.
Over time, more children are visiting the emergency department (ED) for mental health conditions and are vulnerable to prolonged length of stay (LOS). National trends in ED LOS and characteristics associated with prolonged LOS have not been well described.
LOS for pediatric mental health ED visits is increasing over time. By 2014 to 2015, on average, 12.7% of visits exceeded 12 hours. Hispanic children are nearly 3 times as likely to have a prolonged visit than non-Hispanic white children.
For the increasing number of children who use the emergency department (ED) for mental health needs, 1 – 12 prolonged ED length of stay (LOS) is a common adverse outcome 13 – 17 that impacts quality, the patient experience, and efficiency of our health care system. 18 – 20 Time spent in the ED represents a delay in accessing definitive mental health care. 9 , 21 – 23 The ED environment is not well suited to address mental health needs and can be traumatic because of loud noise, frequent changes in providers, and security presence. 24 Furthermore, prolonged LOS leads to general ED crowding, rendering other patients at risk for delays, decreased quality of care, 9 , 25 – 28 and leaving the ED without being seen by a provider. 26 , 29 Acknowledging the impact of prolonged LOS on quality, The Joint Commission defined ED visits >4 hours as prolonged, 30 and the National Quality Forum endorsed 2 relevant measures: NQF0495 and NQF0496. 31
Previous literature reveals that children who present to the ED with mental health needs are susceptible to prolonged LOS. 13 – 17 , 22 , 32 – 34 Recent changes to the health care landscape, including the 2010 Affordable Care Act, 35 coupled with efforts at the state, local, and institutional level, may have impacted access to care over time. 11 , 36 – 44 Children who are traditionally marginalized as racial and ethnic minorities, 9 , 16 , 33 living in poverty and/or publicly insured, 6 , 8 , 15 or who are without a concurrent physical health diagnosis may be particularly vulnerable to prolonged LOS. 12 , 45 More current, nationally representative, pediatric-specific data that describe trends over time and visit characteristics associated with prolonged ED LOS for mental health visits are lacking.
Accordingly, we described more than a decade (2005–2015) of national, temporal trends in ED LOS, comparing pediatric mental health visits with non–mental health visits. Second, we examined whether demographic and clinical characteristics (race and ethnicity, payer type, absence of a concurrent physical health diagnosis) were associated with prolonged ED LOS for mental health visits.
We conducted an observational analysis of pediatric ED visits between 2005 and 2015 using the National Hospital Ambulatory Medical Care Survey (NHAMCS). NHAMCS is a cross-sectional survey of ambulatory and ED visits to US hospitals (excluding federal, military, and Veterans Affairs facilities) administered by the National Center for Health Statistics (NCHS) of the Centers for Disease Control and Prevention. The NHAMCS uses 3-stage probability sampling to provide survey-weighted national estimates. 46 All NHAMCS data are deidentified and publicly available; this study was exempt from the local institutional review board.
We included ED visits for patients ages 6 to 17 years 6 , 9 , 47 between 2005 and 2015. We excluded visits with missing ED LOS data and a LOS of 0 minutes (5% of the total ED visits for ages 6–17), visits of patients who were dead on arrival or died in the ED, and visits of patients who left before or after triage, before being seen by a provider, or against medical advice ( Fig 1 ).
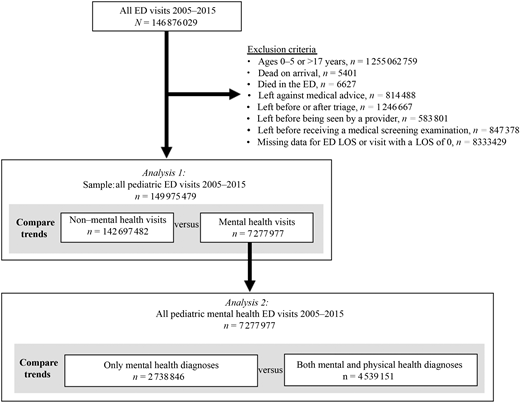
Inclusion and exclusion criteria and visit sample used for each analysis.
We examined 3 definitions of our primary outcome, prolonged ED LOS: >6, >12, and >24 hours. ED LOS >6 hours is a conservative approximation of The Joint Commission definition (>4 hours) 30 and consistent with most studies used to examine mental health ED visits. 15 , 16 , 32 – 34 , 48 We explored 2 additional definitions of prolonged ED LOS, >12 and >24 hours, with face validity as being disruptive to patients’ care and experience. We defined prolonged ED LOS by time cutoffs as opposed to mutually exclusive time intervals to optimize our sample size and ensure model stability.
Consistent with previous studies in which researchers use NHAMCS data, 8 , 9 , 15 , 17 , 49 – 51 we defined an ED visit as a mental health visit if any of the first 3 discharge diagnoses contained a mental health International Classification of Diseases , Ninth Revision diagnosis code, defined by the Child and Adolescent Mental Health Disorder Classification System. The Child and Adolescent Mental Health Disorder Classification System classifies pediatric mental health disorders across International Classification of Diseases coding systems and aligns with the Diagnostic and Statistical Manual of Mental Health Disorders, Fifth Edition and Clinical Classifications Software. 52 The full list of diagnostic codes is publicly available on the Children’s Hospital Association Web site. 53 We included diagnoses of autism spectrum disorder and developmental delay and injury codes related to suicide attempt and intentional self-harm. We further categorized pediatric mental health ED visits as visits with only mental health diagnoses or visits with both mental and physical health diagnoses.
On the basis of clinical relevance and previous literature, we explored the relationship between prolonged ED LOS and demographic variables: race and ethnicity (NCHS-generated 4-level variable); payer type (private, public, other); and clinical variables: mental health visits with only mental health diagnoses versus mental health visits with both mental and physical health diagnoses.
We reported visits as survey-weighted estimates and rates of prolonged ED LOS as proportions (ED visits with LOS >x hours)/total visits). As recommended by the NCHS, to ensure reliable estimates, we grouped data into 2-year increments and did not report population-level estimates for <30 observations or relative SEs >30%. 46
Using descriptive statistics, we compared rates and temporal trends (2005–2015) in prolonged LOS between pediatric mental health and non–mental health ED visits. We then used survey-weighted logistic regression, examining the relationship between our 3 prolonged LOS outcomes, mental health versus non–mental health visits, and year. We used an interaction term to assess whether changes over time (by 1-year intervals) differed by mental health versus non–mental health visit. We adjusted for sex, age, payer type, race and ethnicity, and visit occurring during a school month (September to June).
We used survey-weighted logistic regression to examine the relationship between our 3 prolonged LOS outcomes and demographic and clinical visit characteristics. For demographic variables, we prespecified comparisons between Black non-Hispanic, Hispanic, and other race and ethnicity versus white non-Hispanic, as well as public and other payer type versus private payer. For clinical variables, we prespecified comparisons between visits with only mental health diagnoses versus visits with both physical and mental health diagnoses. We adjusted for age, sex, year, and school versus summer month. To statistically test whether trends over time differed between visits with only mental health diagnoses and visits with both physical and mental health diagnoses, we added an interaction term between year and type of mental health visit to the regression model.
Because of sample size constraints, we largely restricted our reported results to ED LOS >6 and >12 hours and limited reported results for ED LOS >24 hours to summary statistics.
To test the robustness of our findings using categorical ED LOS variables, we repeated each analysis using ED LOS as a continuous variable.
ED mental health visits resulting in admission or transfer often have longer LOS. 32 To assess whether differences in prolonged LOS might be explained by differences in disposition type, we compared mental health and non–mental health ED visits by disposition type and examined changes in disposition type over time.
All models were examined at the visit level by using survey sampling weights. Analyses were conducted by using R version 3.6.3.
We studied 36 125 records, which, after survey weighting, represent 149 975 479 pediatric ED visits between 2005 and 2015, 7 277 997 (4.9%) of which were mental health visits. Mental health visits had higher rates of prolonged LOS compared with non–mental health ED visits: 21.2% vs 4.8% >6 hours, 7.7% vs 1.2% >12 hours, and 1.9% vs 0.3% >24 hours. On average each year, 12 279 ED visits had an LOS >24 hours. Of mental health visits, 61% were white non-Hispanic, 20% were Black non-Hispanic, and 17% were Hispanic; 46% used a public payer and 35% used private insurance only; 62.4% had both mental health and physical health diagnoses associated with the visit and 37.6% had only mental health diagnoses associated with the visit ( Table 1 ).
Characteristics of Pediatric Mental Health and Non–Mental Health Visits by Prolonged ED LOS (2005–2015)
All results represent weighted estimates. N/A, not applicable to visit sample; N/R, not reportable because of unreliable survey-weighted estimates (<30 unweighted observations or relative SEs >30%).
Between 2005 and 2015, rates of prolonged ED LOS remained stable for non–mental health visits but increased for mental health visits. From 2005–2006 to 2014–2015, the average annual rate of ED LOS >6 hours for mental health visits increased from 16.3% to 24.6% (441 542 visits) and from 5.3% (86 791 visits) to 12.7% (227 010 visits) for LOS >12 hours ( Fig 2 , Supplemental Table 4 ).
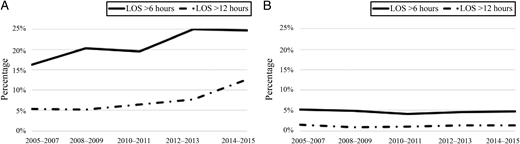
Trends over time in rates of prolonged ED LOS for pediatric mental health and non–mental health visits (2005–2015). All results represent weighted estimates. We were unable to report population-level estimates for visits with prolonged LOS >24 hours because of sample size. A, Mental health ED visits. B, Non–mental health ED visits.
After adjusting for visit characteristics in regression analysis ( Table 2 ), the odds of prolonged LOS were greater for mental health visits compared with non–mental health visits at each LOS outcome: a 3.3-times greater odds of LOS >6 hours (95% confidence interval [CI] 2.2–4.9) and a 2.9-times greater odds of LOS >12 hours (95% CI 1.3–6.2). Additionally, the interaction between mental health visit and year was statistically significant for LOS >6 hours (odds ratio [OR] 1.1; 95% CI 1.0–1.2) and LOS >12 hours (OR 1.15; 95% CI 1.0–1.3) revealing that the relationship between prolonged ED LOS and year differed by type of visit. Stratified models revealed a statistically significant and positive relationship between prolonged ED LOS and year for mental health visits but not for non–mental health visits ( Supplemental Table 5 ).
Adjusted ORs of Prolonged ED LOS for Pediatric Mental Health Versus Non–Mental Health Visits and Year of Visit (2005–2015)
Adjusted for age, sex, payer type, race and ethnicity, and visit during school month. P < .05 is considered statistically significant. aOR, adjusted odds ratio.
Year in 1-year intervals.
Whereas a higher proportion of mental health visits resulted in admission or transfer (27.9%) compared with non–mental health visits (4.4%) ( Supplemental Table 6 , Supplemental Fig. 3 ), the distribution of disposition type did not change over time for mental health or non–mental health visits ( Supplemental Fig. 4 ).
Regression analysis ( Table 3 ) revealed that visits for Hispanic compared with white non-Hispanic children had increased odds of LOS >12 hours (OR 2.74; 95% CI 1.7–4.4). There was otherwise no statistically significant difference in prolonged LOS by race and ethnicity or payer type. After adding an interaction term to the model ( Supplemental Table 7 ), there was a statistically significant relationship between LOS >12 hours and the interaction between year and type of mental health visit (OR 1.3; 95% CI 1.1–1.6), revealing that the relationship between prolonged ED LOS and year differed by type of mental health visit. Stratified models revealed a statistically significant and positive relationship between prolonged ED LOS and year for mental health visits with only mental health diagnoses but not for mental health visits with both mental and physical health diagnoses ( Supplemental Table 8 ).
Adjusted ORs of Prolonged ED LOS for Pediatric Mental Health Visits by Demographic and Clinical Characteristics (2005–2015)
P < .05 is considered statistically significant. aOR, adjusted odds ratio.
Repeating regression analyses by using ED LOS as a continuous as opposed to a categorical variable yielded similar results.
Over the 11-year study period, the odds of prolonged LOS for mental health ED visits were threefold greater and increased over time compared with non–mental health ED visits, which remained stable. Compared with white non-Hispanic children, Hispanic children had an increased risk of prolonged ED LOS. There was no difference in ED LOS by payer type. Additionally, the odds of prolonged ED LOS for visits with only mental health diagnoses increased over time in comparison with visits with both mental and physical health diagnoses.
This study builds on previous literature suggesting that pediatric mental health ED visits are more vulnerable to prolonged LOS, 13 – 17 , 22 , 32 – 34 as well as recent literature indicating a rise in pediatric mental health ED visits over time, most notably from Kalb et al 9 and Lo et al, 54 who examine two different nationally representative data sets over the same time period as our study. Our study reveals that this increasing number of children seeking mental health care in the ED are also increasingly vulnerable to prolonged ED LOS. Although a higher percentage of mental health ED visits resulted in admission or transfer compared with non–mental health visits, the proportion of mental health visits resulting in admission or transfer did not change over time and thus cannot explain increasing rates of prolonged ED LOS for these visits. Although the etiology of prolonged ED LOS is likely multifactorial, the increasing rate of prolonged ED LOS over time, especially at the extremes of LOS >12 and >24 hours, likely represents worsening access to essential mental health services across the care continuum, namely, in the ED, inpatient, and outpatient settings. Concurrent with an increasing incidence of mental health conditions, over the past half century, the number of inpatient psychiatric beds has declined without compensatory expansion of outpatient services. 55 – 57 Mental health care for children is expensive, and suboptimal reimbursement limits incentives to expand services. 56 There continues to be a dearth of child psychiatrists and community supports. 3 , 58 EDs struggle to safely discharge children who present in crisis to appropriate care settings in light of limited services and poor coverage, which in turn leads to prolonged ED LOS. 59
Although limited previous literature explicitly examines the association between ED LOS and race and ethnicity for mental health visits, 9 , 16 , 33 our study reveals that mental health ED visits for Hispanic patients have an increased odds of prolonged LOS compared with visits for white non-Hispanic patients. Further research should be used to explore etiologies of prolonged ED LOS for the Hispanic population, which likely include structural drivers of poor access to mental health care such as racism, 49 , 51 , 60 – 64 language barriers, fear of accessing care, and limited insurance coverage related to immigration status. 65 , 66 Individual institutions should examine their own ED LOS data to ensure equity for all children with mental health needs.
We were interested in the relationship between LOS and payer type, as an indicator of socioeconomic status and comparative access based on insurance. Previous literature reveals inconsistent results. 8 , 15 , 32 Our study revealed no significant difference in rates of prolonged ED LOS by payer type. Examining the relationship between payer type and ED LOS on a national level is challenging. Approximately 40% of children in the United States are covered by state-run Medicaid programs 67 ; therefore, differences in coverage and reimbursement between private and public insurers vary by state. The Affordable Care Act mandated inclusion of mental health treatment under minimum standards for Medicaid coverage; however, states often define these mandated services differently. In some states, Medicaid might provide more comprehensive coverage for mental health care than private insurers, and in other states, less coverage is provided. Future analysis should be used to examine ED LOS among patients with Medicaid at the state level to understand variation in access. 68 – 70
Previous literature reveals that a concurrent physical health diagnosis impacts use patterns in mental health visits. 12 , 45 We hypothesized that visits with both mental and physical health diagnoses would be associated with decreased rates of prolonged ED LOS because they would include presentations necessitating prompt admission to a medical unit, such as injuries or toxidromes secondary to attempts at self-harm. In contrast, the disposition and LOS for visits with only mental health diagnoses would be dictated by the mental health condition and associated with higher rates of prolonged ED LOS. We found that the rate of prolonged ED LOS for visits with only mental health diagnoses increased over time compared with visits with a concurrent physical health diagnosis, which supports our primary finding: for children requiring definitive access to mental health care, the rate of prolonged ED LOS is increasing over time.
During our study period and more recently, prolonged ED LOS for children with mental health conditions has become increasingly visible among the medical and lay community, 71 , 72 leading to promising changes at the health system and policy level. 42 , 43 , 47 However, there is still much to be done. Currently, the majority of EDs are not adequately resourced nor have written policies to care for children with mental health conditions. 23 Kalb et al 9 note that only 16% of children who presented to the ED for a mental health visit are seen by a mental health provider. Every ED should establish protocols, based on existing recommendations and tool kits, 73 – 77 that address pediatric mental health and secure 24-hour access to pediatric-trained mental health providers via in-person consultation or telepsychiatry. 78 ED staff should be trained in trauma-informed care, verbal de-escalation, pharmacologic, and when necessary, physical restraint for children. EDs with high volumes of pediatric mental health patients should consider dedicating space within or adjacent to the ED to ensure a therapeutic environment and to help manage throughput. 59 Furthermore, quality improvement programs that include root cause analyses and institution-specific interventions have the potential to improve care for children with mental health needs. 18 , 38
Beyond the ED, inadequate access to mental health care for children must also be addressed through changes in state and federal policy. 18 , 36 High-profile court cases, such as in Washington State, 37 , 39 have resulted in significant local policy change and have motivated other states to pursue legislation protective against prolonged LOS. 40 , 79 , 80 Policy must address the shortage of providers 3 , 4 and expand coverage and reimbursement for the full spectrum of mental health services including inpatient treatment, community-based interventions, and integrated primary care and medical home models to ensure increased and equitable access to care across payer types. 44 , 59 , 81 , 82
This study has limitations. First, NHAMCS collects data at the visit as opposed to at the patient level; therefore, we cannot assess the impact of repeat visits. Second, sample size constraints and the quality of discharge diagnosis data limited more granular visit categorization and our ability to determine if a mental health diagnosis was the primary reason for visit or if there was a contributing comorbid condition or noncontributing but significant comorbid condition. Although the NHAMCS is a national database and our sample represents millions of ED visits, the relatively small number of mental health visits limited our ability to report accurate estimates for ED LOS >24 hours, as well as some subanalyses, such as stratification of regression models by disposition type. Because prolonged LOS and admission or transfer are likely related, it is possible that demographic characteristics associated with prolonged LOS actually represent predictors of admission or transfer. Further research should be used to explore characteristics associated with prolonged LOS, specifically for visits resulting in admission or transfer. Finally, the NHAMCS does not provide hospital location data beyond region. State-level data might have provided additional insight into the association between payer type and ED LOS.
Rates of prolonged ED LOS for pediatric mental health visits are increasing over time. Despite national attention to a pediatric mental health epidemic, our study suggests that timely and definitive access to mental health care for children is worsening. By 2014–2015, nearly 450 000 annual ED visits exceeded 6 hours and 227 010 visits exceeded 12 hours. Over the 11-year study period, 135 070 visits exceeded 24 hours. Vulnerability to prolonged ED LOS in mental health visits is not equitable: Hispanic patients have an almost threefold increased odds of prolonged LOS compared with white non-Hispanic patients. We found no difference in ED LOS by payer type; however, aggregate national data can obscure variation and important relationships at the state level. Although future research should be used to further explore drivers of prolonged LOS, we must address this crisis in access to acute mental health care for children through changes in our health care delivery systems, state policy, and federal policy.
We dedicate this article in memory of our coauthor Dr Marjorie Rosenthal.
Dr Nash conceptualized and designed the study, drafted the initial manuscript, and revised the manuscript; Drs Zima contributed to the conceptualization and design of the study, critically reviewed the manuscript for important intellectual content, and reviewed and revised the manuscript; Mr Rothenberg conducted data analyses and reviewed and revised the manuscript; Drs Moreno, Hoffmann, and Rosenthal contributed to the conceptualization and design of the study and critically reviewed and revised the manuscript; Dr Venkatesh conceptualized and designed the study, critically reviewed the manuscript for important intellectual content, and reviewed and revised the manuscript; and all authors approved the final manuscript as submitted and agree to be accountable for all aspects of the work.
FUNDING: This publication was made possible by the National Clinician Scholars Program and the Clinical and Translational Science Award grant TL1 TR001864 from the National Center for Advancing Translational Science, a component of the National Institutes of Health (NIH). Its contents are solely the responsibility of the authors and do not necessarily represent the official view of the NIH. Dr Venkatesh reports support of the American Board of Emergency Medicine–National Academy of Medicine Anniversary Fellowship and previous support of award KL2 TR001862 from the National Center for Advancing Translational Science, a component of the NIH. The funders/sponsors did not participate in the work. Dr Hoffmann reports support from the US Agency for Healthcare Research and Quality (5K12HS026385-03). Funded by the National Institutes of Health (NIH).
COMPANION PAPER: A companion to this article can be found online at www.pediatrics.org/cgi/doi/10.1542/peds.2020-049843 .
confidence interval
emergency department
length of stay
National Center for Health Statistics
National Hospital Ambulatory Medical Care Survey
Competing Interests
Supplementary data.
Advertising Disclaimer »
Citing articles via
Email alerts.

Affiliations
- Editorial Board
- Editorial Policies
- Journal Blogs
- Pediatrics On Call
- Online ISSN 1098-4275
- Print ISSN 0031-4005
- Pediatrics Open Science
- Hospital Pediatrics
- Pediatrics in Review
- AAP Grand Rounds
- Latest News
- Pediatric Care Online
- Red Book Online
- Pediatric Patient Education
- AAP Toolkits
- AAP Pediatric Coding Newsletter
First 1,000 Days Knowledge Center
Institutions/librarians, group practices, licensing/permissions, integrations, advertising.
- Privacy Statement | Accessibility Statement | Terms of Use | Support Center | Contact Us
- © Copyright American Academy of Pediatrics
This Feature Is Available To Subscribers Only
Sign In or Create an Account
- General Nursing
- Nursing Specialties
- Nursing Students
- United States Nursing
- World Nursing
- Boards of Nursing
- Breakroom / Clubs
- Nurse Q&A
- Student Q&A
- Fastest BSN
- Most Affordable BSN
- Fastest MSN
- Most Affordable MSN
- Best RN to BSN
- Fastest RN to BSN
- Most Affordable RN to BSN
- Best LPN/LVN
- Fastest LPN/LVN
- Most Affordable LPN/LVN
- Fastest DNP
- Most Affordable DNP
- Medical Assistant
- Best Online Medical Assistant
- Best Accelerated Medical Assistant
- Most Affordable Medical Assistant
- Nurse Practitioner
- Pediatric NP
- Neonatal NP
- Oncology NP
- Acute Care NP
- Aesthetic NP
- Women's Health NP
- Adult-Gerontology NP
- Emergency NP
- Best RN to NP
- Psychiatric-Mental Health NP
- RN Specialties
- Best RN Jobs and Salaries
- Aesthetic Nurse
- Nursing Informatics
- Nurse Case Manager
- Forensic Nurse
- Labor and Delivery Nurse
- Psychiatric Nurse
- Pediatric Nurse
- Travel Nurse
- Telemetry Nurse
- Dermatology Nurse
- Best NP Jobs and Salaries
- Family NP (FNP)
- Orthopedic NP
- Psychiatric-Mental Health NP (PMHNP)
- Nurse Educator
- Nurse Administrator
- Certified Nurse Midwife (CNM)
- Clinical Nurse Specialist (CNS)
- Certified Registered Nurse Anesthetist (CRNA)
- Best Free Online NCLEX-RN Study Guide
- The Nursing Process
- Question Leveling
- NCLEX-RN Question Identification
- Expert NCLEX-RN Test-Taking Strategies
- Best Scrubs for Nurses
- Best Shoes for Nurses
- Best Stethoscopes for Nurses
- Best Gifts for Nurses
- Undergraduate
- How to Become an LPN/LVN
- How to Earn an ADN
- Differences Between ADN, ASN, AAS
- How to Earn a BSN
- Best MSN Concentrations
- Is an MSN Worth It?
- How to Earn a DNP
- MSN vs. DNP
Anyone else have Extended ER???
Specialties Emergency
Published Jun 8, 2011
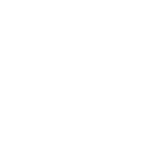
- + Add a Comment
nurse2033, MSN, RN
3 Articles; 2,133 Posts
We call this Clinical Decision Unit. It's for overnight, ambulatory patients who might need a stress test in AM or non urgent appy for example, tele is available.
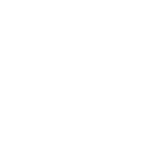
1,850 Posts
Hmmmm, it sounds like you are talking about pts being admitted as observation, which, for billing purposes, is treated like an ED visit for the first 23 hours. There are some exceptions that go longer than that, but I couldn't begin to tell you what they are.
As for what qualifies them for extended ER, or observation, I don't know that either. Criteria depends on a lot of things, and one larger hospital that I work for has utilization review nurses who work right in the ER to make sure patients who are admitted as inpatients or observation actually meet criteria, and the CRM nurses change it if they don't. Docs can disagree (though they rarely do) and still admit a patient as observation or inpatient even if they don't meet critera, but they will then have that case put up for review and potentially be penalized by the hospital if they are wrong.
So, in short, it can be complicated and not something the average nurse knows how to determine.
I think this is a good general statement. But just to clarify this for the OP, different CDUs can have their own criteria concerning which patients they will accept to that unit, but for purposes of classifying a patient for observation to the CDU, they don't have to be ambulatory, and they could be admitted for many different reasons besides those stated above.
Edited to add: Observation, extended ER, whatever they are called, those patients don't HAVE to go to the CDU. If the CDU is full, obs patients can be placed in a room on the floor without compromising their observation status or the hospital's ability to bill as obs.
I should also add that the CLASSIFICATION of a patient as observation or extended ER is for billing purposes and is different than extended ER, or CDU as a location where these types of patients typically stay while in the hospital, so the criteria you are looking for may be based on location rather than billing. If so, then that's a different ballgame. I also work at a smaller hospital that doesn't have a separate CDU, so all obs patients are admitted to the floor.
I once had an obs patient at this hospital stay right in the ER because the 23 hour clock includes all time spent in the ER from the time patients are first admitted to the ER, and by the time he was dispositioned, it didn't make sense to move him to a bed upstairs for the remaining few hours that he would be there.
Thank you for all the information!!! Leads me to another question. How long does everyone keep their pts in the ER? For example, a pt comes in dehyrdrated and IVF are started. This pt is not put in obs, but kept in the ER recieving fluids. Anyone have a policy stating how long a pt like this can be kept? Is anything over 4 hrs acceptable in other ER departments? Do other ER departments have a time limit on whether to admit a pt (either full admit or obs)?
Altra, BSN, RN
6,255 Posts
I think there are several things wrapped up in your question.
In and out of the ER in under 4 hours ... is either a low-acuity/urgent care type patient on a good, not very busy, fast-moving day OR a sick-as-crap patient who quickly heads to the OR, cath lab or ICU (on a magical day when there are ICU beds immediately available).
Your "dehydration" example is pretty generic -- lots of folks who are not elderly or do not have other acute conditions or multiple comorbidities can and do come in to the ER after a day or two of vomiting/diarrhea with "dehydration" and we tank them up with some fluids and then discharge them if they show sufficient improvement. It's not necessary to admit them, but their total ER time may well be longer than 4 hours.
Clinical Decision Units can be very successful at pulling out patients who don't quite meet the threshhold for a typical obs admission out of the main ER flow.
I've not worked anywhere where there was a "time limit" by policy on a decision to admit -- because it's so dependent on so many factors beyond the control of ER physicians and nurses.
Is your ED trying to implement what you're calling "extended ER" and finding difficulty/resistance? If so, what are the sticking points?
My problem is we are a rural hospital with no CDU. We either admit (full admit or observation, treat and release, or transfer). Somehow, over the years "extended ER" has developed in our ER. Basically, doctors place this pt in that status to receive numerous treatments. It varies from IVF, to q 1 hr FSBS, to just watching the pt. We do not have a policy covering "extended ER". Several Pts are being kept from anywhere from 2-24 hrs as "extended ER". Our "extended ER" is unofficial and needs guidelines. Just attempting to get an idea on how others handle or even deal with situations like this. I am finding most do not!!! I have found that at Stony Brook University Hospital, they have a policy in place to make a decision to admit, DC, or transfer after 8 hrs.
Sorry forgot one more thing. The average stay for an "extended ER" pt is 16 hrs.
GM2RN, My problem is we are a rural hospital with no CDU. We either admit (full admit or observation, treat and release, or transfer). Somehow, over the years "extended ER" has developed in our ER. Basically, doctors place this pt in that status to receive numerous treatments. It varies from IVF, to q 1 hr FSBS, to just watching the pt. We do not have a policy covering "extended ER". Several Pts are being kept from anywhere from 2-24 hrs as "extended ER". Our "extended ER" is unofficial and needs guidelines. Just attempting to get an idea on how others handle or even deal with situations like this. I am finding most do not!!! I have found that at Stony Brook University Hospital, they have a policy in place to make a decision to admit, DC, or transfer after 8 hrs.
I can tell you more about mine when I have a bit more time. Gotta get ready for work now...

Official websites use .gov
A .gov website belongs to an official government organization in the United States.
Secure .gov websites use HTTPS
A lock ( ) or https:// means you've safely connected to the .gov website. Share sensitive information only on official, secure websites.
Heat-Related Emergency Department Visits — United States, May–September 2023
Weekly / April 18, 2024 / 73(15);324–329
Ambarish Vaidyanathan, PhD 1 ; Abigail Gates, MSPH 2 ; Claudia Brown, MDP 1 ; Emily Prezzato, MPH 1 ; Aaron Bernstein, MD 3 ( View author affiliations )
What is already known about this topic?
Unprecedented heat waves can affect all persons, but some are more sensitive to the effects of heat, including children and adults with underlying health conditions, pregnant women, and outdoor workers.
What is added by this report?
During the 2023 warm-season months (May–September), rates of emergency department visits for heat-related illness substantially increased across several U.S. regions compared with previous years, especially among males and adults aged 18–64 years.
What are the implications for public health practice?
Heat-related illness will continue to be a significant public health concern as climate change results in longer, hotter, and more frequent episodes of extreme heat. By monitoring heat-related health impacts, public health agencies can detect trends in health care utilization rates, identify subpopulations at increased risk, and guide public health actions tailored to specific heat exposure levels.
- Article PDF
- Full Issue PDF
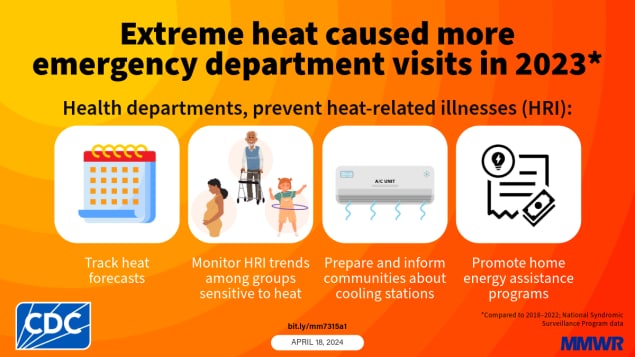
Unprecedented heat waves can affect all persons, but some are more sensitive to the effects of heat, including children and adults with underlying health conditions, pregnant women, and outdoor workers. Many regions of the United States experienced record-breaking high temperatures in 2023, with populations exposed to extremely high temperatures for prolonged periods. CDC examined emergency department (ED) visits associated with heat-related illness (HRI) from the National Syndromic Surveillance Program and compared daily HRI ED visit rates during the warm-season months (May–September) of 2023 with those during 2018–2022. In the 2023 warm-season months, daily HRI ED visit rates peaked in several regions and remained elevated for a prolonged duration. More males than females sought care in EDs for HRI, especially males aged 18–64 years. CDC issued multiple public health alerts using the Epidemic Information Exchange system to bring attention to increases in ED utilization for HRI. Deaths and illnesses associated with heat exposure are a continuing public health concern as climate change results in longer, hotter, and more frequent episodes of extreme heat. Near real-time monitoring of weather conditions and adverse health outcomes can guide public health practitioners’ timing of risk communication and implementation of prevention measures associated with extreme heat.
Introduction
The warm-season months (May–September) of 2023 were the hottest ever recorded in the United States,* and adverse health impacts, including deaths and illnesses attributable to high ambient temperatures, received considerable attention. † Hot weather conditions can affect all persons; however, for certain specific populations, exposure and health risks are compounded by adverse physiologic, behavioral, demographic, or socioeconomic factors that result in their being disproportionately affected by extreme heat ( 1 ). Populations at highest risk typically include older persons, children and adolescents, persons with preexisting health conditions, pregnant women, outdoor workers, persons with limited access to cooling resources, and persons living in low-income communities. § Further, exceptionally hot conditions can increase the demand for medical services and strain health systems (e.g., a surge in persons seeking emergency department [ED] care) ( 2 ). Successful public health measures to reduce heat-related illness (HRI), including targeted communication and outreach campaigns for populations at risk, require coordination across various health care sectors and are often guided by near real-time assessments of heat exposure and its resulting adverse health impacts. To assess the health impact of exceedingly high temperatures observed during the warm-season months of 2023, CDC analyzed National Syndromic Surveillance Program (NSSP) data to compare daily HRI ED visit rates during May–September 2023 with those during May–September 2018–2022.
Data Sources
Data on HRI ED visits ¶ occurring during January 2018–December 2023 were extracted from NSSP’s Electronic Surveillance System for the Early Notification of Community-Based Epidemics (ESSENCE).** The daily number of HRI and all-cause ED visits were tabulated for each of the 10 U.S. Department of Health and Human Services (HHS) regions. †† NSSP data were analyzed to compare the 2023 heat season with the 2018–2022 seasons. To account for temporal changes among facilities sharing data with NSSP, comparisons between 2023 and previous years were restricted to those EDs with consistent reporting during the study period. §§
Descriptive and Statistical Analyses
After applying data quality filters to reduce artifactual changes in reporting patterns during 2018–2023, a maximum of 826 (range = 3–826; median = 36) ED facilities that participate in NSSP reported one or more visits associated with HRI. The HHS region–specific daily HRI ED visit rate (the number of ED visits for HRI per 100,000 all-cause ED visits) observed during the warm-season months of 2023 was compared with the 95th percentile value of the daily HRI ED visit rate distribution. The 95th percentile for each region was computed based on HRI ED data recorded for the 2018–2022 warm-season months.
Differences in HRI ED visit rates were evaluated by age group (0–17, 18–25, 26–54, 55–64, 65–74, and ≥75 years), sex, HHS region, and occurrence of the HRI ED visits during the hotter warm-season months (i.e., July and August). Rate ratios (RRs) and associated 95% CIs were estimated using a multivariate Poisson regression model. The daily number of HRI visits was regressed against predictors such as age group, sex, HHS region, and an indicator to denote the occurrence of HRI ED visits during the hotter warm-season months of July and August. The model also included the logarithm of all-cause ED visits to account as an offset term. For each predictor, the category with the lowest warm-season HRI ED visit rate was identified as the referent population. Regressions were executed for 2023 and 2018–2022 with the same model specifications and parameters. Analysis and visualization were conducted using R software (version 4.1.2; R Foundation) and SAS software (version 9.4; SAS Institute). This activity was reviewed by CDC, deemed not research, and was conducted consistent with applicable federal law and CDC policy. ¶¶
Characteristics of HRI ED Visits
During January 1–December 31, 2023, a total of 119,605 HRI ED visits were recorded in the ESSENCE system***; 92% of these visits occurred during May–September. Across the study period, July and August accounted for a higher average HRI ED visit rate (303 per 100,000 ED visits) compared with other warm-season months (May, June, and September) (97) ( Table 1 ). Further, the risk observed during July–August 2023 was more than three times that during May, June, and September (mean RR = 3.07), consistent with record-breaking temperatures observed across several HHS regions in 2023. ††† In comparison, the risk observed in July–August 2018–2022 was approximately twice as high as that of May, June, and September of the same period.
Demographic Characteristics of Persons with HRI ED Visits
In 2023, among the demographic groups considered, higher rates of HRI ED visits were observed among males (271 per 100,000 ED visits) than among females (104) and among adults aged 18–64 years (range = 207–222) than adults aged ≥65 years (range = 120–173). In addition, the risk for HRI ED visits among adults aged 18–25 and 26–54 years was approximately 2.5 times the risk in the referent population (persons aged <18 years).
Regional Differences in HRI ED Visits
HHS regional differences in warm-season HRI ED visit rates were observed in 2023. The lowest average warm-season HRI ED visit rate (51 per 100,000 ED visits) was reported by HHS Region 2 (New Jersey and New York), whereas the highest rate was reported by Region 6 (Arkansas, Louisiana, New Mexico, Oklahoma, and Texas) (483). Compared with Region 2 (the referent region), the HRI ED visit risks for regions 4, 6, 7, and 9 in 2023 were 1.5–2.5 times those during 2018–2022.
Daily HRI ED visit rates during the warm-season months in 2023 for several regions exceeded the 95th percentile of the daily HRI ED visit rate distribution for the warm-season months during 2018–2022 for multiple periods of ≥3 consecutive days in some regions (Supplementary Figure, https://stacks.cdc.gov/view/cdc/153146 ). For instance, in regions 6 and 9, HRI ED rates in July 2023 exceeded the 2018–2022 95th percentile for 16 and 18 consecutive days, respectively. In the warm-season months of 2023, every HHS region experienced ≥1 day above the 95th percentile ( Table 2 ). In regions 4, 6, 7, and 9, the number of days with HRI ED visit rates exceeding the 95th percentile was higher than that in any previous year in the study period. In Region 6 alone, more than one third (37%; 56) of the days during the warm season of 2023 had daily HRI ED visit rates exceeding the 95th percentile. Regions 6 and 7 experienced days with the highest rate of HRI ED visits ever recorded in the ESSENCE system for their respective region since 2018.
In recent years, health emergencies caused by heat exposure have become more frequent and widespread in the United States ( 1 ). The severity, frequency, and duration of heat waves in 2023 in some HHS regions resulted in record-high rates of HRI ED visits during the year, which prompted CDC to issue Epidemic Information Exchange (Epi-X) public health alerts. §§§
The finding of increased risk for HRI ED visit rates among certain demographic groups in 2023, particularly among males and adults aged 18–64 years, is similar to findings reported in other studies ( 3 ). Although the lowest HRI ED visit rates occurred among persons aged <18 years, previous studies of children and adolescents in different age groups suggest that children might also be subject to the effects of heat exposure at rates similar to those among adults in some areas of the United States ( 4 ). Persons who work outdoors might regularly endure extreme heat; this group warrants particular attention because of the high prevalence of HRI ED visits observed in working-aged adults. Frontline essential workers tending to emergencies, such as firefighters, might be at particularly high risk for exposure to heat stress ( 5 ). Regional differences in rates of HRI ED visits might reflect differential acclimatization, behavioral responses, and adaptation strategies ( 1 , 6 ). Understanding the causes of these differences can help guide the development and implementation of public health interventions, such as heat action plans and issuance of heat alerts calibrated based on local epidemiologic data (e.g., HeatRisk). ¶¶¶
Effective implementation of heat mitigation strategies is associated with social determinants of health. For example, even in areas with high rates of air conditioning, such as the South and southeastern United States, persons exposed to extreme heat might have limited or no access to cooling spaces ( 1 ). Factors that affect air conditioning use and access to cooling spaces include energy costs**** and the occurrence of outages due to power grid failure ( 1 , 7 , 8 ). HHS programs that provide financial assistance for residential energy †††† and monitor the safety of persons reliant on electricity-dependent durable medical equipment in case of power outages during extreme heat §§§§ can protect populations affected by heat stress. The intersection of communities with a high proportion of groups at risk, especially those with limited access to health care, with areas that experience persistent high ambient temperatures (e.g., heat islands or lack of green spaces) could be more susceptible to the effects of heat exposure ( 1 ). Public health initiatives can be designed to help communities prepare for extreme heat conditions and complement the efforts of weather and emergency management agencies, reducing illnesses and deaths. Tools used for syndromic surveillance, including ESSENCE, local systems, and visualization dashboards, help guide and strengthen public health preparedness and response. An example is CDC’s Heat and Health Tracker ( https://ephtracking.cdc.gov/Applications/heatTracker/ ), which provides local heat and health information for communities.
Limitations
The findings in this report are subject to at least five limitations. First, NSSP data are not nationally representative, and participation can vary by HHS region. Second, although the prevalence of HRI among U.S. military veterans has been increasing ( 9 ), this analysis does not include facilities operated by U.S. Department of Veterans Affairs. In addition, the HRI ED visit rate reported by ESSENCE might not be representative of the rate in the general population because ESSENCE is not a population-based system but rather reflects the number of HRI ED visits among all-cause ED visits. Third, HRI information reported at the HHS regional level can obscure subregional variation. Fourth, estimation of HRI ED visit rates might have been affected during the COVID-19 pandemic because overall ED utilization patterns changed for specific subpopulations ( 10 ). Finally, HRI data from the ESSENCE system are based on ED visits only and do not identify cases of HRI among persons who sought treatment elsewhere, likely resulting in an underestimation of HRI prevalence.
Implications for Public Health Practice
The record-breaking temperatures of the 2023 warm-weather season had a substantial public health impact, and this trend might increase in the coming years because of climate change ( 1 ). Public health agencies rely on tools and surveillance systems to assess the adverse health effects of heat exposure. Timely mechanisms for tracking and reporting health effects, along with the ability to detect anomalous trends, especially during extreme heat emergencies, can facilitate the implementation of public health strategies to protect affected populations.
Acknowledgments
Shubhayu Saha, Paul Schramm, Division of Environmental Health Science and Practice, National Center for Environmental Health, CDC; Sue Swensen, Office of Public Health Data, Surveillance, and Technology, CDC.
Corresponding author: Ambarish Vaidyanathan, [email protected] .
1 Division of Environmental Health Science and Practice, National Center for Environmental Health, CDC; 2 Detect and Monitor Division, Office of Public Health Data, Surveillance, and Technology, CDC; 3 National Center for Environmental Health, Agency for Toxic Substances and Disease Registry, CDC.
All authors have completed and submitted the International Committee of Medical Journal Editors form for disclosure of potential conflicts of interest. No potential conflicts of interest were disclosed.
* https://www.nasa.gov/news-release/nasa-announces-summer-2023-hottest-on-record/
† https://www.whitehouse.gov/briefing-room/statements-releases/2023/07/27/fact-sheet-president-biden-to-announce-new-actions-to-protect-workers-and-communities-from-extreme-heat
§ https://www.cdc.gov/disasters/extremeheat/index.html
¶ HRI ED visits were identified using administrative discharge diagnosis codes and free text search of the patient’s reason for visit (i.e., their chief complaint). https://knowledgerepository.syndromicsurveillance.org/heat-related-illness-v2
** https://publichealth.jmir.org/2021/6/e26303/authors
†† The dataset does not include data from American Samoa, Federated States of Micronesia, Marshall Islands, Northern Mariana Islands, Palau, Puerto Rico, or the U.S. Virgin Islands. https://www.hhs.gov/about/agencies/iea/regional-offices/index.html
§§ To reduce artifactual impact from changes in reporting patterns, analyses were restricted to facilities with a coefficient of variation for ED visits ≤40 and average weekly informative discharge diagnosis ≥70% complete with discharge diagnosis code formatting during January 2018–December 2023. After applying this data quality filter, a maximum of 823 ED (range = 3–823; median = 111) facilities that participate in NSSP returned one or more visits associated with HRI. https://www.cdc.gov/nssp/index.html
¶¶ 45 C.F.R. part 46.102(l)(2), 21 C.F.R. part 56; 42 U.S.C. Sect. 241(d); 5 U.S.C. Sect. 552a; 44 U.S.C. Sect. 3501 et seq.
*** All facilities reporting HRI data to NSSP were considered to obtain the total HRI ED visits reported during January 1–December 31, 2023, across all HHS regions. During this period, HRI data were reported by a maximum of 1,238 EDs (range = 12–1,238; median = 152) that participated in NSSP and returned one or more visits associated with HRI.
††† https://www.ncei.noaa.gov/access/monitoring/monthly-report/global/202313
§§§ On June 30, 2023, and August 23, 2023, CDC issued public health alerts using the Epi-X system upon noticing high levels of heat-related ED visits.
¶¶¶ HeatRisk is a health-based heat forecast developed by the National Oceanic and Atmospheric Administration’s National Weather Service and CDC. It integrates health and temperature data to deliver a 7-day outlook for hot weather. HeatRisk uses a 5-level scale to indicate how risky the heat level is in a specific area. www.cdc.gov/HeatRisk
**** https://neada.org/wp-content/uploads/2023/07/summercoolingestPR.pdf
†††† https://www.acf.hhs.gov/ocs/programs/liheap
§§§§ https://empowerprogram.hhs.gov/
- US Global Change Research Program. The fifth national climate assessment. Washington, DC: US Global Change Research Program; 2023. https://nca2023.globalchange.gov
- Schramm PJ, Vaidyanathan A, Radhakrishnan L, Gates A, Hartnett K, Breysse P. Heat-related emergency department visits during the northwestern heat wave—United States, June 2021. MMWR Morb Mortal Wkly Rep 2021;70:1020–1. https://doi.org/10.15585/mmwr.mm7029e1 PMID:34292925
- Sun S, Weinberger KR, Nori-Sarma A, et al. Ambient heat and risks of emergency department visits among adults in the United States: time stratified case crossover study. BMJ 2021;375:e065653. https://doi.org/10.1136/bmj-2021-065653 PMID:34819309
- Bernstein AS, Sun S, Weinberger KR, Spangler KR, Sheffield PE, Wellenius GA. Warm season and emergency department visits to US children’s hospitals. Environ Health Perspect 2022;130:17001. https://doi.org/10.1289/EHP8083 PMID:35044241
- Kim S, Kim D-H, Lee H-H, Lee J-Y. Frequency of firefighters’ heat-related illness and its association with removing personal protective equipment and working hours. Ind Health 2019;57:370–80. https://doi.org/10.2486/indhealth.2018-0063 PMID:30210098
- Hondula DM, Balling RC Jr, Vanos JK, Georgescu M. Rising temperatures, human health, and the role of adaptation. Curr Clim Change Rep 2015;1:144–54. https://doi.org/10.1007/s40641-015-0016-4
- Mallen E, Roach M, Fox L, et al. Extreme heat exposure: access and barriers to cooling centers—Maricopa and Yuma counties, Arizona, 2010–2020. MMWR Morb Mortal Wkly Rep 2022;71:781–5. https://doi.org/10.15585/mmwr.mm7124a1 PMID:35709011
- Andresen AX, Kurtz LC, Hondula DM, Meerow S, Gall M. Understanding the social impacts of power outages in North America: a systematic review. Environ Res Lett 2023;18:053004. https://doi.org/10.1088/1748-9326/acc7b9
- Osborne TF, Veigulis ZP, Vaidyanathan A, Arreola DM, Schramm PJ. Trends in heat related illness: nationwide observational cohort at the US Department of Veteran Affairs. J Clim Change Health 2023;12:100256. https://doi.org/10.1016/j.joclim.2023.100256
- Hartnett KP, Kite-Powell A, DeVies J, et al.; National Syndromic Surveillance Program Community of Practice. Impact of the COVID-19 pandemic on emergency department visits—United States, January 1, 2019–May 30, 2020. MMWR Morb Mortal Wkly Rep 2020;69:699–704. https://doi.org/10.15585/mmwr.mm6923e1 PMID:32525856
Abbreviations: ED = emergency department; HHS = U.S. Department of Health and Human Services; HRI = heat-related illness; NA = not applicable; Ref = referent group; RR = rate ratio. * To reduce artifactual impact from changes in reporting patterns, analyses were restricted to facilities with a coefficient of variation for ED visits ≤40 and average weekly informative discharge diagnosis ≥70% complete with discharge diagnosis code formatting during January 2018–December 2023. After applying this data quality filter, a maximum of 823 ED (range = 3–823; median = 111) facilities that participate in the National Syndromic Surveillance Program returned one or more visits associated with HRI. https://www.cdc.gov/nssp/index.html † HRI ED visits per 100,000 ED visits. § https://www.hhs.gov/about/agencies/iea/regional-offices/index.html ¶ Region 2 (Ref) includes New Jersey, New York, Puerto Rico, and the U.S. Virgin Islands. Puerto Rico and the U.S. Virgin Islands currently do not report data to the National Syndromic Surveillance Program.
Abbreviations: ED = emergency department; HHS = U.S. Department of Health and Human Services. * 95th percentile based on region-specific heat-related illness ED visit rate during warm-season months (May–September) during 2018–2022. † To reduce artifactual impact from changes in reporting patterns, analyses were restricted to facilities with a coefficient of variation for ED visits ≤40 and average weekly informative discharge diagnosis ≥70% complete with discharge diagnosis code formatting during January 2018–December 2023. After applying this data quality filter, a maximum of 823 ED (range = 3–823; median = 111) facilities that participate in the National Syndromic Surveillance Program returned one or more visits associated with heat-related illness. https://www.cdc.gov/nssp/index.html § https://www.hhs.gov/about/agencies/iea/regional-offices/index.html ¶ Region 2 includes New Jersey, New York, Puerto Rico, and the U.S. Virgin Islands. Puerto Rico and the U.S. Virgin Islands currently do not report data to the National Syndromic Surveillance Program.
Suggested citation for this article: Vaidyanathan A, Gates A, Brown C, Prezzato E, Bernstein A. Heat-Related Emergency Department Visits — United States, May–September 2023. MMWR Morb Mortal Wkly Rep 2024;73:324–329. DOI: http://dx.doi.org/10.15585/mmwr.mm7315a1 .
MMWR and Morbidity and Mortality Weekly Report are service marks of the U.S. Department of Health and Human Services. Use of trade names and commercial sources is for identification only and does not imply endorsement by the U.S. Department of Health and Human Services. References to non-CDC sites on the Internet are provided as a service to MMWR readers and do not constitute or imply endorsement of these organizations or their programs by CDC or the U.S. Department of Health and Human Services. CDC is not responsible for the content of pages found at these sites. URL addresses listed in MMWR were current as of the date of publication.
All HTML versions of MMWR articles are generated from final proofs through an automated process. This conversion might result in character translation or format errors in the HTML version. Users are referred to the electronic PDF version ( https://www.cdc.gov/mmwr ) and/or the original MMWR paper copy for printable versions of official text, figures, and tables.
Exit Notification / Disclaimer Policy
- The Centers for Disease Control and Prevention (CDC) cannot attest to the accuracy of a non-federal website.
- Linking to a non-federal website does not constitute an endorsement by CDC or any of its employees of the sponsors or the information and products presented on the website.
- You will be subject to the destination website's privacy policy when you follow the link.
- CDC is not responsible for Section 508 compliance (accessibility) on other federal or private website.
- Introduction
- Conclusions
- Article Information
eTable 1. ICES Data Sources
eTable 2. Operational Definitions of All Variables
eTable 3. Search Strategy for Direct-to-Consumer (Virtual-Only Walk-In Clinic) Group Numbers
eTable 4. Characteristics of Patients in Unmatched Cohort
eTable 5. Top 20 Diagnoses of All Patients in Matched Cohort
eTable 6. Relative Risk of Having an Emergency Department Visit After a Virtual Visit With a Physician Outside Patient Enrolling Group by Age and Rurality Subgroup
eTable 7. Sensitivity Analysis: Characteristics of Patients in the Matched Cohort
eTable 8. Patient Outcomes in the Matched Sensitivity Cohort
eFigure 1. Study Population Flowchart
eFigure 2. Density of Propensity Scores Before and After Matching
eFigure 3. Kaplan-Meier Curve of Time to Emergency Department Visit for Patients With Index Virtual Visit With Own Enrolling Family Physician and Physician Outside Enrolling Group
eFigure 4. Kaplan-Meier Curve of Time to Emergency Department Visit for Patients With Index Virtual Visit With Own Enrolling Physician and Virtual-Only Walk-In Clinic
eReferences.
Data Sharing Statement
See More About
Sign up for emails based on your interests, select your interests.
Customize your JAMA Network experience by selecting one or more topics from the list below.
- Academic Medicine
- Acid Base, Electrolytes, Fluids
- Allergy and Clinical Immunology
- American Indian or Alaska Natives
- Anesthesiology
- Anticoagulation
- Art and Images in Psychiatry
- Artificial Intelligence
- Assisted Reproduction
- Bleeding and Transfusion
- Caring for the Critically Ill Patient
- Challenges in Clinical Electrocardiography
- Climate and Health
- Climate Change
- Clinical Challenge
- Clinical Decision Support
- Clinical Implications of Basic Neuroscience
- Clinical Pharmacy and Pharmacology
- Complementary and Alternative Medicine
- Consensus Statements
- Coronavirus (COVID-19)
- Critical Care Medicine
- Cultural Competency
- Dental Medicine
- Dermatology
- Diabetes and Endocrinology
- Diagnostic Test Interpretation
- Drug Development
- Electronic Health Records
- Emergency Medicine
- End of Life, Hospice, Palliative Care
- Environmental Health
- Equity, Diversity, and Inclusion
- Facial Plastic Surgery
- Gastroenterology and Hepatology
- Genetics and Genomics
- Genomics and Precision Health
- Global Health
- Guide to Statistics and Methods
- Hair Disorders
- Health Care Delivery Models
- Health Care Economics, Insurance, Payment
- Health Care Quality
- Health Care Reform
- Health Care Safety
- Health Care Workforce
- Health Disparities
- Health Inequities
- Health Policy
- Health Systems Science
- History of Medicine
- Hypertension
- Images in Neurology
- Implementation Science
- Infectious Diseases
- Innovations in Health Care Delivery
- JAMA Infographic
- Law and Medicine
- Leading Change
- Less is More
- LGBTQIA Medicine
- Lifestyle Behaviors
- Medical Coding
- Medical Devices and Equipment
- Medical Education
- Medical Education and Training
- Medical Journals and Publishing
- Mobile Health and Telemedicine
- Narrative Medicine
- Neuroscience and Psychiatry
- Notable Notes
- Nutrition, Obesity, Exercise
- Obstetrics and Gynecology
- Occupational Health
- Ophthalmology
- Orthopedics
- Otolaryngology
- Pain Medicine
- Palliative Care
- Pathology and Laboratory Medicine
- Patient Care
- Patient Information
- Performance Improvement
- Performance Measures
- Perioperative Care and Consultation
- Pharmacoeconomics
- Pharmacoepidemiology
- Pharmacogenetics
- Pharmacy and Clinical Pharmacology
- Physical Medicine and Rehabilitation
- Physical Therapy
- Physician Leadership
- Population Health
- Primary Care
- Professional Well-being
- Professionalism
- Psychiatry and Behavioral Health
- Public Health
- Pulmonary Medicine
- Regulatory Agencies
- Reproductive Health
- Research, Methods, Statistics
- Resuscitation
- Rheumatology
- Risk Management
- Scientific Discovery and the Future of Medicine
- Shared Decision Making and Communication
- Sleep Medicine
- Sports Medicine
- Stem Cell Transplantation
- Substance Use and Addiction Medicine
- Surgical Innovation
- Surgical Pearls
- Teachable Moment
- Technology and Finance
- The Art of JAMA
- The Arts and Medicine
- The Rational Clinical Examination
- Tobacco and e-Cigarettes
- Translational Medicine
- Trauma and Injury
- Treatment Adherence
- Ultrasonography
- Users' Guide to the Medical Literature
- Vaccination
- Venous Thromboembolism
- Veterans Health
- Women's Health
- Workflow and Process
- Wound Care, Infection, Healing
Get the latest research based on your areas of interest.
Others also liked.
- Download PDF
- X Facebook More LinkedIn
Lapointe-Shaw L , Salahub C , Austin PC, et al. Virtual Visits With Own Family Physician vs Outside Family Physician and Emergency Department Use. JAMA Netw Open. 2023;6(12):e2349452. doi:10.1001/jamanetworkopen.2023.49452
Manage citations:
© 2024
- Permissions
Virtual Visits With Own Family Physician vs Outside Family Physician and Emergency Department Use
- 1 University Health Network, Toronto, Ontario, Canada
- 2 ICES, Toronto, Ontario, Canada
- 3 Institute of Health Policy, Management and Evaluation, University of Toronto, Toronto, Ontario, Canada
- 4 Department of Medicine, University of Toronto, Toronto, Ontario, Canada
- 5 Women’s College Institute for Health System Solutions and Virtual Care, Women’s College Hospital, Toronto, Ontario, Canada
- 6 Division of General Internal Medicine and Geriatrics, University Health Network and Sinai Health System, Toronto, Ontario, Canada
- 7 Sunnybrook Research Institute, Toronto, Ontario, Canada
- 8 Department of Cardiology, University Health Network, Toronto, Ontario, Canada
- 9 Patient Partner, Toronto, Ontario, Canada
- 10 Department of Family and Community Medicine, University of Toronto, Toronto, Ontario, Canada
- 11 MAP Centre for Urban Health Solutions, St Michael’s Hospital, Toronto, Ontario, Canada
- 12 Faculty of Health Sciences, Simon Fraser University, Burnaby, British Columbia, Canada
- 13 Department of Family Medicine, Women’s College Hospital, Toronto, Ontario, Canada
- 14 Women’s College Research Institute, Women’s College Hospital, Toronto, Ontario, Canada
- 15 Leslie Dan Faculty of Pharmacy, University of Toronto, Toronto, Ontario, Canada
- 16 Department of Family and Community Medicine, St Michael’s Hospital, Unity Health Toronto, Toronto, Ontario, Canada
Question Does health care use differ after a virtual visit with a patient’s own family physician compared with a visit with an outside physician?
Findings In this cohort study among 5 229 240 Ontario residents with a family physician and virtual visit, patients who had a virtual visit with an outside physician were 66% more likely to visit the emergency department within 7 days compared with those with a virtual visit with their own physician.
Meaning This finding suggests that virtual care with an outside physician is associated with more emergency department visits.
Importance Virtual visits became more common after the COVID-19 pandemic, but it is unclear in what context they are best used.
Objective To investigate whether there was a difference in subsequent emergency department use between patients who had a virtual visit with their own family physician vs those who had virtual visits with an outside physician.
Design, Setting, and Participants This propensity score–matched cohort study was conducted among all Ontario residents attached to a family physician as of April 1, 2021, who had a virtual family physician visit in the subsequent year (to March 31, 2022).
Exposure The type of virtual family physician visit, with own or outside physician, was determined. In a secondary analysis, own physician visits were compared with visits with a physician working in direct-to-consumer telemedicine.
Main Outcome and Measure The primary outcome was an emergency department visit within 7 days after the virtual visit.
Results Among 5 229 240 Ontario residents with a family physician and virtual visit, 4 173 869 patients (79.8%) had a virtual encounter with their own physician (mean [SD] age, 49.3 [21.5] years; 2 420 712 females [58.0%]) and 1 055 371 patients (20.2%) had an encounter with an outside physician (mean [SD] age, 41.8 [20.9] years; 605 614 females [57.4%]). In the matched cohort of 1 885 966 patients, those who saw an outside physician were 66% more likely to visit an emergency department within 7 days than those who had a virtual visit with their own physician (30 748 of 942 983 patients [3.3%] vs 18 519 of 942 983 patients [2.0%]; risk difference, 1.3% [95% CI, 1.2%-1.3%]; relative risk, 1.66 [95% CI, 1.63-1.69]). The increase in the risk of emergency department visits was greater when comparing 30 216 patients with definite direct-to-consumer telemedicine visits with 30 216 patients with own physician visits (risk difference, 4.1% [95% CI, 3.8%-4.5%]; relative risk, 2.99 [95% CI, 2.74-3.27]).
Conclusions and Relevance In this study, patients whose virtual visit was with an outside physician were more likely to visit an emergency department in the next 7 days than those whose virtual visit was with their own family physician. These findings suggest that primary care virtual visits may be best used within an existing clinical relationship.
The COVID-19 pandemic has fueled the growth of virtual care throughout the health care system. 1 - 7 This has included direct-to-consumer telemedicine (also known as virtual-only walk-in clinics), which typically offers on-demand virtual health care without an option for an in-person exam and is disconnected from a patient’s existing source of primary care. 8 Although some argue that direct-to-consumer telemedicine met a gap in patient care needs, 9 it also amplified tensions between continuity and access to timely or convenient care. 8 Furthermore, the ease of access afforded by on-demand virtual care could drive demand for in-the-moment health care that otherwise would not exist. 10 Some studies have found that direct-to-consumer telemedicine was associated with decreases in health care use by replacing in-person care, 11 , 12 while others suggest that it was associated with increased total use and costs. 13 - 17
Continuity is a cornerstone of good primary care, and numerous studies have demonstrated that high relational continuity is associated with better health outcomes and lower costs. 18 - 22 However, little research has been done to understand differences in subsequent health care use when virtual primary care is provided in the context of an ongoing relationship vs outside of that relationship, as is the case for direct-to-consumer telemedicine.
We used population-based administrative data from Ontario, Canada, to compare subsequent health care use after a virtual visit with a patient’s own family physician vs an outside family physician. We hypothesized that virtual primary care delivered outside an existing relationship would be associated with increased emergency department use.
The use of data in this cohort study is authorized under section 45 of Ontario’s Personal Health Information Protection Act and did not require review by a research ethics board or informed consent. We followed the Strengthening the Reporting of Observational Studies in Epidemiology ( STROBE ) reporting guideline for cohort studies.
We conducted a population-based, retrospective, propensity score–matched cohort study of residents in Ontario, Canada, who had a virtual visit with a family physician from April 1, 2021, to March 31, 2022. Ontario is Canada’s most populous province, with more than 14.5 million residents. Provincial health insurance is provided to all permanent residents without premiums or copayments and covers emergency department visits, hospitalizations, and all medically necessary physician care. Most primary care is provided by family physicians, and nearly 80% of the population is enrolled to a family physician working in a patient enrollment model. 23 Enrolling practices are expected to meet all primary care needs, including after-hours care. 24 In capitation-based models (the model for 65% of enrolling physicians), there is negation (reduction in an access bonus) when patients of enrolling physicians visit family physicians outside the enrolling group. 25
After the onset of the COVID-19 pandemic in 2020, the Ministry of Health introduced several new, temporary physician billing codes for synchronous virtual visits conducted by video or phone, with a fee equivalent to that of in-person visits. Since then, most publicly funded virtual visits have been conducted by phone. 26 , 27 Asynchronous visits, provided by email or text message, were not covered by provincial insurance.
Population-based health administrative data sets (eTable 1 in Supplement 1 ) were linked using unique encoded identifiers and analyzed at ICES in Ontario, Canada. ICES is an independent, nonprofit research institute whose legal status under Ontario health information privacy law allows the institute to collect and analyze health care and demographic data without consent for health system evaluation and improvement.
We included all Ontarians who were enrolled to a family physician practicing in a patient enrollment model as of April 1, 2021. We excluded individuals with missing Rurality Index of Ontario scores (a measure of urban or rural residence) or neighborhood income quintile information (<1% missing). 28
The exposure (a binary variable) was determined at the first virtual family physician visit (ie, index) that was with the patient’s own enrolling physician or with another physician outside the enrolling group from April 1, 2021, to March 31, 2022. We excluded virtual visits with family physicians who worked in a focused practice or with an emergency medicine subspecialization. 29
We did not include virtual visits with another physician within the same group given that we sought to contrast highest-continuity virtual visits (own physician) with lowest-continuity virtual visits (outside of group). Virtual family physician visits outside the enrolling group likely represented encounters with direct-to-consumer telemedicine clinics (also known as virtual-only walk-in clinics) or combined virtual and in-person walk-in clinics.
The primary outcome was the occurrence of an emergency department visit within 7 days of the index virtual encounter. Secondary outcomes were a low-acuity emergency department visit (defined as a Canadian Triage and Acuity Scale [CTAS] score of 4-5) within 7 days, an emergency department visit within 30 days, a time to emergency department visit within 30 days, and an in-person or virtual visit with any family physician, the same physician, or own enrolling physician within 7 days. Post hoc outcomes were emergency department visits on day 1 and day 2, a high-acuity emergency department visit within 7 days (CTAS score, 1-2), and a tracer outcome of an emergency department visit for a high-acuity (CTAS score, 1-2) motor vehicle accident on days 3 to 30. 30 This tracer was chosen given that the acuity level and separation from the index virtual visit by 3 days made it unlikely that such an event would be a cause or consequence of the type of virtual visit.
We included the following patient characteristics (see operational definitions in eTable 2 in Supplement 1 ): age, sex, urban or rural residence, 31 neighborhood income quintile, and whether the patient was a recent insurance registrant (within the past 10 years), a proxy for recent immigration. 23 We also reported the type of primary care enrollment model, visits to the enrolling physician in the previous 2 years, and the burden of comorbidities using the Johns Hopkins ACG System version 10 resource utilization bands (RUBs), which are based on diagnoses and health care use over the previous 2 years. 32 , 33 Related to the index visit, we measured the diagnosis (top 20 categorized), the calendar quarter, whether the visit was on a weekend, if an after-hours code was claimed, and whether the visit was by phone or video or was missing this information (a required code to identify phone or video was introduced in October 2021).
We first described the cohort using mean (SD), median (IQR), and counts and frequencies. We compared characteristics between groups in unmatched and matched cohorts using standardized mean differences (SMDs), with a difference of 0.1 (10%) or greater considered meaningful. 34
We derived propensity scores using a logistic regression model that included age, sex, neighborhood income quintile, recent immigrant status, count of visits with the patient’s own physician in the previous 2 years, RUB, enrollment model, diagnosis, modality, and whether the visit was on a weekend or after hours on a weekday. Age and count of visits were modeled using restricted cubic splines with 5 knots at the 5th, 27.5th, 50th, 72.5th, and 95th percentile values. 35 We then matched exposure groups 1:1 within a caliper distance of 0.2 × the SD of the logit of the propensity score, 36 while also hard matching on Rurality Index of Ontario category (3 categories: 0-9, large urban; 10-40, small urban; and ≥40, rural) and age group (0-17, 18-64, and ≥65 years).
We reported relative risks (RRs) and risk differences (RDs) for all binary outcomes with 95% CIs, accounting for the paired nature of the matched sample. 37 , 38 For the time-to-event outcome, we reported the hazard ratio obtained using a Cox proportional hazards model with robust variance estimate. 39 Analyses were executed in SAS statistical software version 9.4 (SAS Institute) using a 2-tailed P < .05 statistical significance threshold.
We report primary outcome results for each hard-matched subgroup: patients residing in large urban, small urban, or rural areas and those aged 0 to 17 years, 18 to 64 years, and 65 years or older. We compared the association between type of virtual visit and our tracer outcome to the e value, 40 which is the minimum strength of association that an unmeasured confounder would need to have with the exposure and the outcome to fully explain the observed exposure-outcome association. 41 , 42
To separately assess outcomes associated with low-continuity virtual visits without the possibility of a physical examination, we compared virtual visits to a patient’s own physician with visits to a known direct-to-consumer telemedicine clinic. We identified these visits using group billing numbers, a method we updated 13 for this study (eTable 3 in Supplement 1 ). Notably, this is a convenience sample based on clinics that use group billing numbers; it is unknown what proportion of all direct-to-consumer telemedicine is covered by this definition.
Of 5 229 240 individuals in our cohort (eFigure 1 in Supplement 1 ), 4 173 869 patients (79.8%) had their index virtual encounter with their own physician (mean [SD] age, 49.3 [21.5] years; 2 420 712 females [58.0%]) and 1 055 371 patients (20.2%) had their visit with an outside physician (mean [SD] age, 41.8[20.9] years; 605 614 females [57.4%]). Before matching, patients who had an outside-physician virtual encounter were younger, more often lived in a large urban area (859 772 patients [81.5%] vs 3 207 285 patients [76.8%]; SMD, 0.11), made fewer visits to their own physician in the prior 2 years (mean [SD] 3.2 [4.8] visits vs 7.0 [7.0] visits; SMD, 0.64), were less often enrolled to a non–team capitation model (331 770 patients [31.4%] vs 1 457 804 patients [34.9%]; SMD, 0.13), and more often had visits on weekends (107 945 patients [10.2%] vs 156 859 patients [3.8%]; SMD, 0.26) and using video (14 251 patients [1.4%] vs 9792 patients [0.2%]; SMD 0.13) compared with patients who had an own physician encounter (eTable 4 in Supplement 1 ).
After matching, there were no differences in measured characteristics of 942 983 matched pairs (total 1 885 966 patients) exceeding 10% (SMD of 0.1) ( Table 1 ; eTable 5 and eFigure 2 in Supplement 1 ). For example, the mean (SD) age was 42.3 (21.1) years in the outside physician group and 42.5 (20.8) years in the own physician group (SMD, 0.01). Patients who had a virtual visit with an outside physician saw that physician a median (IQR) of 0 (0-2) times in the 2 years prior to the index visit compared with 2 (0-5) visits with their own physician.
Patients who had a virtual encounter with an outside physician were 66% more likely to visit an emergency department within 7 days (30 748 patients [3.3%] vs 18 519 patients [2.0%]; RD, 1.3% [95% CI, 1.2%-1.3%]; RR, 1.66 [95% CI, 1.63-1.69]) ( Table 2 ). This corresponds to 1 additional emergency department visit for every 77 outside virtual visits. The increase in risk associated with virtual visits with outside vs own physicians was greater for the outcome of low-acuity (7759 patients [0.8%] vs 4084 patients [0.4%]; RR, 1.90 [95% CI, 1.83-1.97]) than high-acuity (7042 patients [0.7%] vs 4836 patients [0.5%]; RR, 1.46 [95% CI, 1.40-1.51]) emergency department visits within 7 days.
The increased risk of an emergency department visit was front-loaded, with an RR of 1.99 (95% CI, 1.93-2.05) on day 1 and 1.86 (95% CI, 1.78-1.93) on day 2 and early separation of survival curves (eFigure 3 in Supplement 1 ). However, there was still an association at 30 days (57 674 patients [6.1%] vs 41 342 patients [4.4%]; RR, 1.40 [95% CI, 1.38-1.41]).
Patients who had an outside-physician virtual encounter were more likely than those with an own physician visit to have an in-person family physician visit within 7 days (57 208 patients [6.1%] vs 45 828 patients [4.9%]; RR, 1.25 [95% CI, 1.23-1.26]) but were less likely to have such a visit with their own physician (9915 patients [1.1%] vs 39 102 patients [4.2%]; RR, 0.25 [95% CI, 0.25-0.26]). Similarly, they were nearly twice as likely to have a virtual visit within 7 days (83 681 patients [8.9%] vs 44 470 patients [4.7%]; RR, 1.88 [95% CI, 1.86-1.90]), and this was also less likely to be with their own physician (19 658 patients [2.1%] vs 39 251 patients [4.2%]; RR, 0.50 [95% CI, 0.49-0.51]).
The increase in risk of a 7-day emergency department visit associated with having an outside-physician virtual visit was greater for younger age groups. Children and adolescents (ages <18 years) were at highest risk (RR, 1.96 [95% CI, 1.86-2.05]), followed by adults (ages 18-64 years; RR, 1.69 [95% CI, 1.65-1.73]) and older adults (ages ≥65 years; RR, 1.40 [95% CI, 1.34-1.45]) (eTable 6 in Supplement 1 ). There were no differences between patients by residence in large urban, small urban, or rural areas.
The type of virtual visit was also associated with our tracer outcome of emergency department visits for high-acuity motor vehicle accidents, with a higher risk for those who visited an outside physician (129 people [0.01%]) than those who visited their own physician (97 people [0.01%]; RD, 0%; RR, 1.33 [95% CI, 1.02-1.73]). This association was weaker than the confounder strength of association ( e value 41 lower confidence limit = 2.32) that would be needed to explain away the findings for our primary outcome.
We used an alternative exposure definition (eTable 7 in Supplement 1 ) that compared a visit with a patient’s own physician with a visit with a known direct-to-consumer telemedicine clinic. In this analysis, 30 216 patients with a direct-to-consumer telemedicine visit were nearly 3 times more likely to visit the emergency department within 7 days than 30 216 patients with own physician visits (1878 patients [6.2%] vs 628 patients [2.1%]; RD, 4.1% [95% CI, 3.8%-4.5%]; RR, 2.99 [95% CI, 2.74-3.27]). Similar to findings in the main cohort, the increased risk was front-loaded in the first 2 days (eTable 8 and eFigure 4 in Supplement 1 ). Direct-to-consumer telemedicine users were more likely to have a repeat virtual visit within 7 days than those with a virtual visit to their own physician (3191 patients [10.6%] vs 1105 patients [3.7%]; RR, 2.89 [95% CI, 2.70-3.09]), although this was not explained by visits with the same physician, their own physician, or another physician in their group.
In this population-based cohort study, we compared outcomes of patients who received a virtual visit with their own family physician with outcomes of those who received a virtual visit with a family physician outside their physician group. We found that the latter patients had more visits to the emergency department in the ensuing 7 days, although the absolute difference was small, at 1.3%, corresponding to 1 additional emergency department visit for every 77 visits outside the group. We found that patients who had a visit with a subset of direct-to-consumer telemedicine clinics had approximately 3 times the risk of 7-day emergency department visits and repeat virtual visits than those who had a virtual visit with their own physician.
The increased use of the emergency department associated with low-continuity virtual visits was front-loaded in the first few days, suggesting that virtual visits may serve a triaging function, allowing for the identification of patients who would benefit from an in-person assessment. Direct-to-consumer telemedicine physicians may direct patients to an in-person follow-up visit, either immediately or if symptoms persist; alternatively, physicians may direct patients to the emergency department for a condition perceived to be urgent. Finally, some patients may choose the emergency department even if not suggested by a physician if they perceive this to be their only option for a timely in-person exam.
Patients having virtual visits outside the enrolling group were also more likely to have repeat virtual or in-person family physician visits, although these were less commonly with their enrolling physician, suggesting that these visits may have been repeat visits to walk-in clinics or virtual walk-in clinics. These patients may have faced challenges or barriers to accessing their usual physician or group, thus leading them to seek care elsewhere, including through a virtual walk-in clinic or an emergency department. Delays to an appointment, even for individuals already attached to a family physician, have been well described in the US and Canada. 43 , 44 However, visits with multiple outside clinicians may be associated with increased costs and fragmented care. 45 Care fragmentation increases the risk for errors and can introduce inconsistent and sometimes conflicting messages, eroding trust in physicians and the health care system as a whole. 18 , 46 - 48 Achieving timely access while preserving care continuity remains an important health policy challenge.
Our study is novel given that we directly contrasted outcomes after high- and low-continuity forms of virtual care. Meanwhile, previous research compared virtual visits with in-person visits. Within-system virtual care (integrated with in-person, office-based care) from US-based Intermountain Healthcare and Kaiser Permanente has compared more favorably with in-person care 49 , 50 than has direct-to-consumer telemedicine, which has been associated with increased downstream health care use and costs. 14 , 15 , 17 , 51 In 2 Ontario studies, 52 , 53 patients whose regular family physicians provided more virtual care did not have higher rates of emergency department use. Furthermore, within-system virtual care has demonstrated the potential for associated increases in equity of care 54 via improved access for individuals who struggle to afford higher visit-related costs. 55 - 57
Some health systems in the US and Europe have adopted integrated virtual care models. 49 , 54 , 58 However, other insurers, such as US Medicare, the UK National Health Service (NHS), and the Canadian provinces of Nova Scotia and British Columbia, have contracted out publicly insured health care services to corporate virtual clinics. 59 - 62 In a time series–based evaluation of privately insured residents of Minnesota, 12 plan members with coverage for direct-to-consumer telemedicine had lower costs of care for episodes of urinary tract infection but not sinusitis after plan expansion. An evaluation 63 of the NHS’s GP at Hand, a program of direct-to-consumer telemedicine supported by in-person locations, found that users had higher rates of consultations than the general population, despite being younger and in better health. This raised concerns about inequities given that public funding was diverted to the service. Policymakers continue to refine virtual care policies to optimize the balance of access, quality, and costs.
Our findings provide evidence to support policy changes that prioritize virtual visits within an existing therapeutic relationship. These findings complement those of McGrail et al 64 from a smaller study of patients who received virtual visits in British Columbia, Canada, in 2013 to 2014. In that study, potential cost savings from virtual visits were greater among patients who saw a known physician. We also build on a previous Ontario-based study 13 in which we found that patients who had visits with virtual walk-in clinics in 2020 were twice as likely as those with other types of virtual family physician visits to visit the emergency department within 30 days. In addition to better addressing potential sources of confounding, this study extends our findings to 2021, when emergency department visit volumes began to rise to their pre-COVID-19 levels. 52
Our study has several limitations. First, some outside-physician virtual visits may have averted emergency department visits for patients who were not able to access their own physician in a timely way. There is no way to identify such patients or determine which elements of access (eg, hours of operation, availability of physicians, or challenges making appointments) may have contributed. Second, we were limited to studying publicly funded virtual visits given that information on privately funded visits was not available. Third, the association between the type of virtual visit and trauma-related emergency department visits is unlikely to be explained by health care–seeking behavior alone given that we included only the highest-acuity visits. This association could reflect differences between groups in risk-taking behavior and health literacy. 65 However, confounding on this basis would not be strong enough to explain the association between the type of virtual visit and 7-day emergency department visits. Patients who perceive their care needs to be urgent or who have a lower tolerance for delays may be more likely to seek immediate care through direct-to-consumer telemedicine and emergency departments, another potential source of confounding. Fourth, Ontario residents do not pay premiums or copayments for any visit type included in this study. Therefore, our findings may not be fully generalizable to settings where outside group care is financially disincentivized.
This cohort study found that for patients attached to a family physician, a virtual visit with an outside physician instead of the patient’s own physician was associated with more emergency department visits and family physician visits in the following week. Our findings support the use of primary care virtual visits within an existing clinician-patient relationship.
Accepted for Publication: November 13, 2023.
Published: December 27, 2023. doi:10.1001/jamanetworkopen.2023.49452
Open Access: This is an open access article distributed under the terms of the CC-BY License . © 2023 Lapointe-Shaw L et al. JAMA Network Open .
Corresponding Author: Lauren Lapointe-Shaw, MD, PhD, University Health Network, 200 Elizabeth St, Toronto, ON M5G 2C4, Canada ( [email protected] ).
Author Contributions: Dr Lapointe-Shaw had full access to all of the data in the study and takes responsibility for the integrity of the data and the accuracy of the data analysis.
Concept and design: Lapointe-Shaw, Bird, Hedden, Ivers, Martin, Shuldiner, Spithoff, Kiran.
Acquisition, analysis, or interpretation of data: Lapointe-Shaw, Salahub, Austin, Bai, Bhatia, Bird, Glazier, Hedden, Ivers, Tadrous, Kiran.
Drafting of the manuscript: Lapointe-Shaw, Salahub, Bird, Ivers.
Critical review of the manuscript for important intellectual content: All authors.
Statistical analysis: Lapointe-Shaw, Bai.
Obtained funding: Lapointe-Shaw, Kiran.
Administrative, technical, or material support: Bhatia, Bird, Shuldiner.
Supervision: Lapointe-Shaw.
Conflict of Interest Disclosures: Dr Glazier reported receiving scientist support from MAP Centre for Urban Health Solutions and ICES and leadership stipends from the Canadian Institutes of Health Research outside the submitted work. No other disclosures were reported.
Funding/Support: This study was supported by ICES, which is funded by an annual grant from the Ontario Ministry of Health (MOH) and the Ministry of Long-Term Care. This study also received funding from the MOH through an Innovations Strengthening Primary Health Care Through Research (INSPIRE-PHC) Applied Health Research Question grant awarded to Dr Lapointe-Shaw and project grant 175285 from the Canadian Institutes of Health Research awarded to Drs Lapointe-Shaw and Ivers.
Role of the Funder/Sponsor: The funders had no role in the design and conduct of the study; collection, management, analysis, and interpretation of the data; preparation, review, or approval of the manuscript; and decision to submit the manuscript for publication.
Disclaimer: The analyses, conclusions, opinions, and statements expressed herein are solely those of the authors and do not reflect those of the funding or data sources; no endorsement is intended or should be inferred.
Data Sharing Statement: See Supplement 2 .
Additional Contributions: We would like to thank Alexander Kopp, BA, (ICES), for contributions to study design and data analysis and our patient partners Krysta Nesbitt and Patrick Roncal for their contributions to result interpretation. Alexander Kopp was not compensated for this work. Patient partners were provided with an hourly honorarium for reviewing study materials and attending project meetings.
Additional Information: This document used data adapted from the Statistics Canada Postal Code OM Conversion File, which is based on data licensed from the Canada Post Corporation and/or data adapted from the Ontario Ministry of Health (MOH) Postal Code Conversion File, which contains data copied under license from the Canada Post Corporation and Statistics Canada. Parts of this material are based on data and/or information compiled and provided by the MOH, Canadian Institutes of Health Research and Ontario Health.
- Register for email alerts with links to free full-text articles
- Access PDFs of free articles
- Manage your interests
- Save searches and receive search alerts
Purdue Online Writing Lab Purdue OWL® College of Liberal Arts
Welcome to the Purdue Online Writing Lab
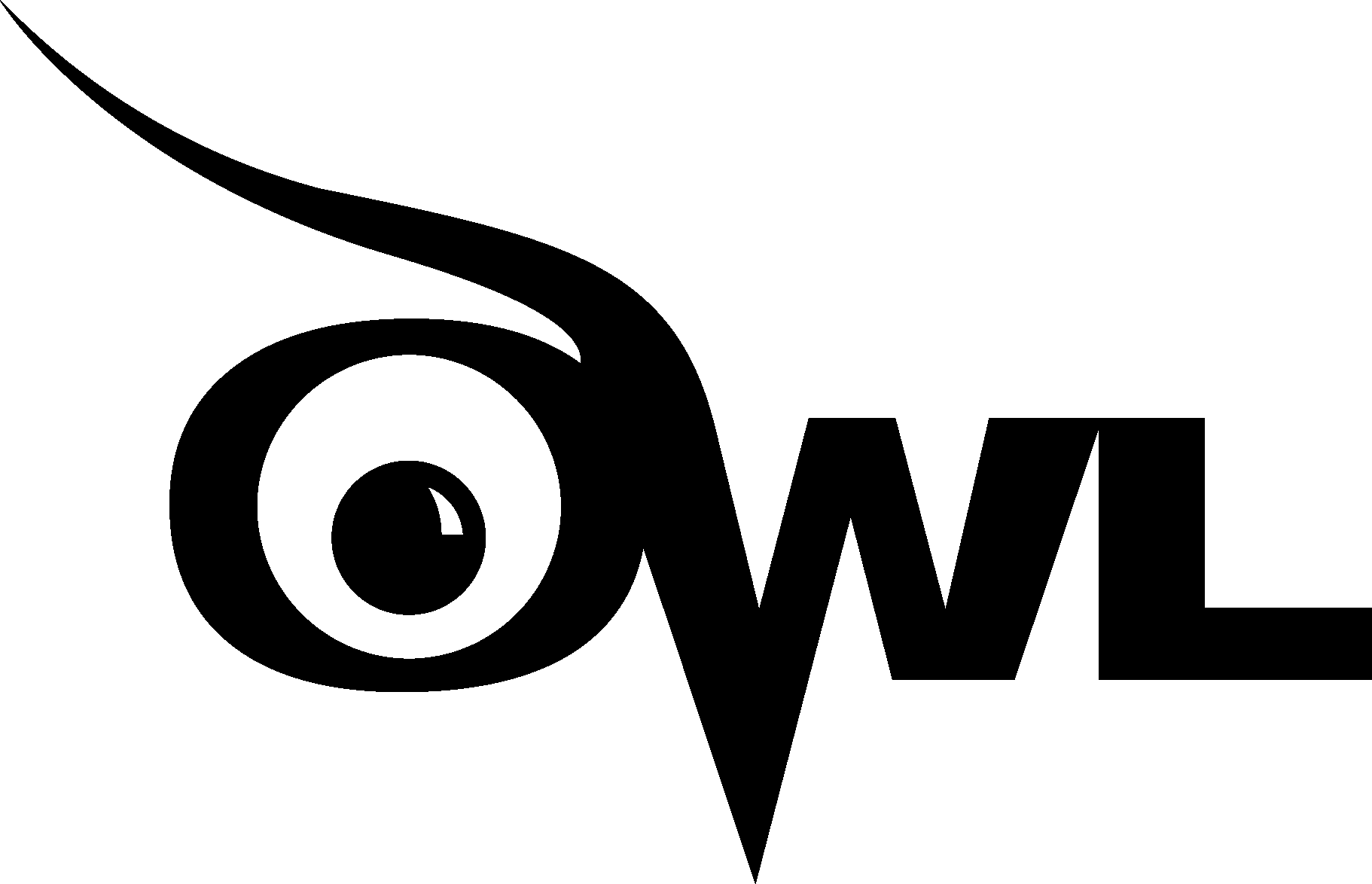
Welcome to the Purdue OWL
This page is brought to you by the OWL at Purdue University. When printing this page, you must include the entire legal notice.
Copyright ©1995-2018 by The Writing Lab & The OWL at Purdue and Purdue University. All rights reserved. This material may not be published, reproduced, broadcast, rewritten, or redistributed without permission. Use of this site constitutes acceptance of our terms and conditions of fair use.
The Online Writing Lab at Purdue University houses writing resources and instructional material, and we provide these as a free service of the Writing Lab at Purdue. Students, members of the community, and users worldwide will find information to assist with many writing projects. Teachers and trainers may use this material for in-class and out-of-class instruction.
The Purdue On-Campus Writing Lab and Purdue Online Writing Lab assist clients in their development as writers—no matter what their skill level—with on-campus consultations, online participation, and community engagement. The Purdue Writing Lab serves the Purdue, West Lafayette, campus and coordinates with local literacy initiatives. The Purdue OWL offers global support through online reference materials and services.
A Message From the Assistant Director of Content Development
The Purdue OWL® is committed to supporting students, instructors, and writers by offering a wide range of resources that are developed and revised with them in mind. To do this, the OWL team is always exploring possibilties for a better design, allowing accessibility and user experience to guide our process. As the OWL undergoes some changes, we welcome your feedback and suggestions by email at any time.
Please don't hesitate to contact us via our contact page if you have any questions or comments.
All the best,
Social Media
Facebook twitter.
- Skip to main content
- Keyboard shortcuts for audio player
TED Radio Hour
- Subscribe to NPR's Up First Email
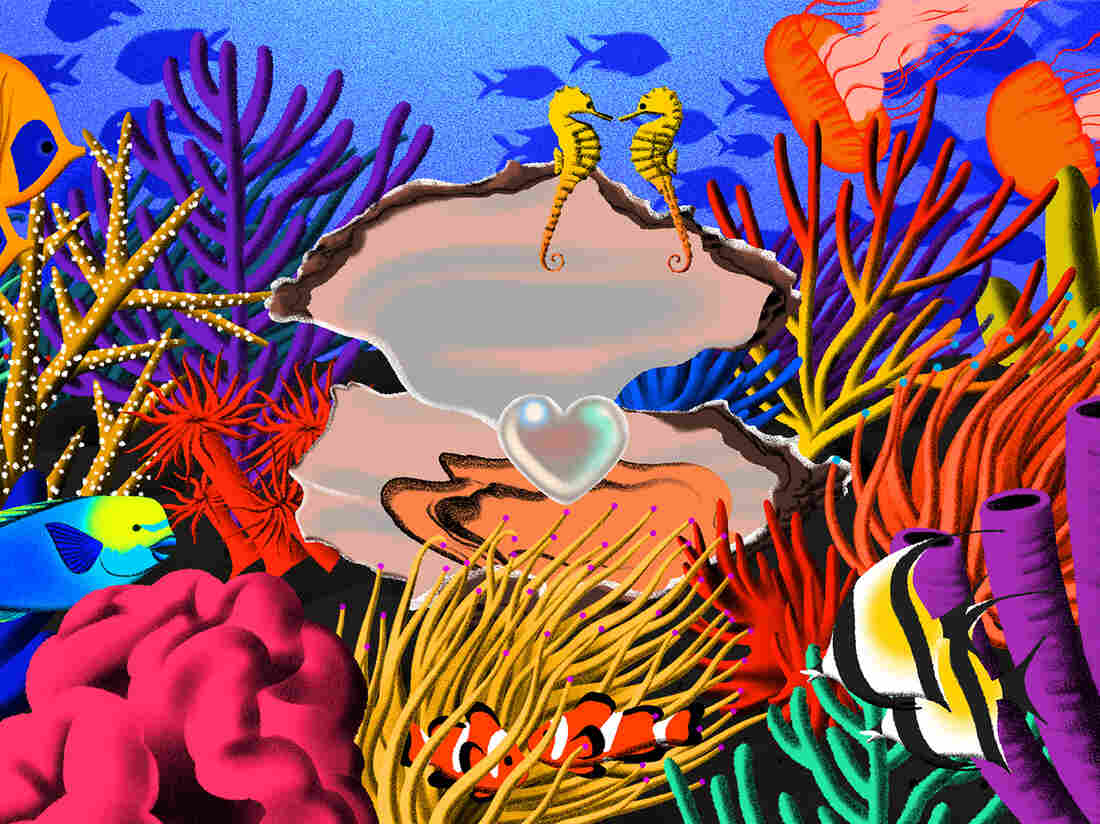
Cathryn Virginia hide caption
A Love Letter To The Ocean
Oceans cover nearly 75% of the Earth. While they seem vast and frightening, they're also enchanting and whimsical. This hour, TED speakers dive into stories of connection — and even love — in the sea.
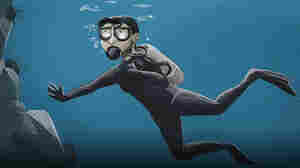
A love story... that begins with a sea urchin
by Manoush Zomorodi , Katie Monteleone , Sanaz Meshkinpour
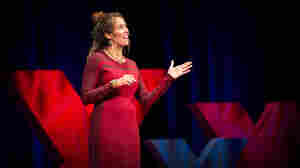
What can we learn from the sex lives of fish?
by Manoush Zomorodi , James Delahoussaye , Sanaz Meshkinpour
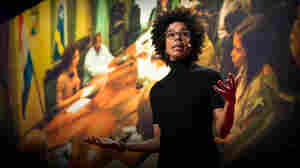
Why the strange and wonderful parrot fish is in trouble
by Manoush Zomorodi , Fiona Geiran , Sanaz Meshkinpour
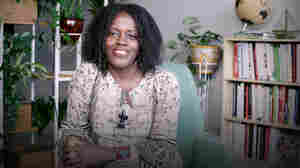
Could snail venom someday save your life?
by Manoush Zomorodi , Rachel Faulkner White , Sanaz Meshkinpour
- See TED Radio Hour sponsors and promo codes

IMAGES
VIDEO
COMMENTS
Emergency Department Visits: n/a: n/a: n/a: Initial NF Visit (99306) G0317: 95 minutes: 1 day before visit + date of visit +3 days after: Subsequent NF Visit (99310) ... * Time must be used to select visit level. Prolonged service time can be reported when furnished on any date within the primary visit's surveyed timeframe, and includes time ...
Coding & Billing Guidelines. Emergency Department (ED) Evaluation and Management (E/M) codes are typically reported per day and do not differentiate between new or established patients. There are 5 levels of emergency department services represented by CPT codes 99281 - 99285. The ED codes require the level of Medical Decision Making (MDM) to ...
• Creation of an extended ED visit professional code after patient is in the ED for greater than six hours. • Require CMS to adopt existing CPT recommendation that emergency physicians may bill for both the ED E/M code and the inpatient/observation care in instances where the patient remains under the care of the ED physician for
99283 (G0382) Emergency department visit for the evaluation and management of a patient, which requires a medically appropriate history and/or examination and low medical-decision making. Any interventions from above, plus any below: Receipt if EMS/Ambulance patient. Heparin/saline lock. One Nebulizer treatment.
with patients or family members within a few days of an ED visit. Contacting patients after an ED visit is important to understand the reason for the visit, identify questions/issues, and review medications. Using a checklist for ED follow-up helps to ensure consistency among patients and to guarantee that all information is gathered and addressed.
99282 - Emergency department visit for the evaluation and management of a patient, which requires a medically appropriate history and/or examination and straightforward medical decision making. ... Prolonged use of these medications can increase the risk of complications, including premature closure of the fetal ductus arteriosus and impaired ...
Highlights. In 2018, there were 143.5 million emergency department (ED) visits, representing 439 visits per 1,000 population. Fourteen percent of ED visits resulted in hospital admission (61 per 1,000 population). Circulatory and digestive system conditions were the most common reasons for these visits. The majority of ED visits (86 percent ...
Codes 99415, 99416 are used when a prolonged evaluation and management (E/M) service is provided in the office or outpatient setting that involves prolonged clinical staff face-to-face time beyond the highest total time of the E/M service, as stated in the ranges of time in the code descriptions.
Berg et al. 3 assessed the impact of prolonged waiting time on the mortality of patients who were allocated lower triage scores. ... The database included more than 700,000 ED visits. This study identified a higher 10-day mortality for the group with a score of 3 (0.16% vs overall mortality of 0.09%), especially if the patients were older and ...
The majority of ED visits (86 percent) resulted in treatment and release (378 per 1,000 population). The two most common general reasons for these visits were (1) injuries and poisonings and (2) symptoms, signs, and abnormal findings. The most common specific reasons for treat-and-release ED visits were abdominal pain, acute upper respiratory ...
Emergency department (ED) services; ... often involving multiple encounters with several patients over an extended period of time." 1 . 99281—ED E/M Code Revised. ... Emergency department visit for the evaluation of a patient that may not require the presence of a physician or other qualified health care professional . E/M code 99281 ...
Effective Jan. 1, 2023, hospital observation codes 99217-99220 and 99224-99226 are deleted. These services are merged into the existing hospital inpatient services codes 99221-99223, 99231-99233, and 99238-99239, and the subsection is renamed Inpatient Hospital or Observation Care. As in the Office or Other Outpatient Services subsection, the ...
During the most recent week, 3,552 EDs reported data. Total ED visit volume, as well as patient age, sex, region, and reason for visit were analyzed. Weekly number of ED visits were examined during January 1, 2019-May 30, 2020. In addition, ED visits during two 4-week periods were compared using mean differences and ratios.
The definition of prolonged EDLOS may vary. Prolonged ED visits have been defined as >4 h in the United Kingdom, >6 h in Canada and the U.S., and >8 h in Australia [4,5,6]. Open in a separate window. Figure 1. Definition of time spent in the emergency department related to each segment of the care process.
Extended ED Mental Health Care Now Reportable as Observation. One in eight visits to the emergency department is related to a mental health or substance abuse issue. That number has grown each year over the past decade. 1 An informal poll of ACEP members showed that 100 percent of those asked had at least one behavioral health patient remaining ...
These changes reflect a once in a generation restructuring of the guidelines for choosing a level of ED E/M visit impacting roughly 85% of the relative value units (RVUs) for typical members. Since 1992, a visit level was based on a combination of history, physical exam, and medical decision-making elements.
Discussion. After a decline in ED visits in the United States associated with the COVID-19 pandemic during March-April 2020 (1), ED visits steadily increased through July 2020, and then stabilized through the fall.During December 2020-January 2021, visits declined again to a level 25% lower than that during December 2019-January 2020.
The E/M codes for home care services now include any patient residence, including assisted living facilities, which prior to 2023 had a separate code category (99324-99328, 99334-99337). Now all home or residence services are reported using codes 99341-99345 for new patients and 99347-99350 for established patients.
Key Points. Question Did patterns of US emergency department (ED) visits and mental health (MH)-related ED visits change during the COVID-19 pandemic?. Findings In this cross-sectional study with 1570 total observations for January 1, 2019, to December 31, 2021, mean total ED visits decreased more than the proportion of ED visits for MH conditions during the weeks after the pandemic was ...
May 02, 2024. Dr. Maria Raven and Dr. Renee Hsia in collaboration with Robert Lowe from OHSU received a grant from the Emergency Medicine Foundation (EMF) to support research on state policy to limit ED visits by denying reimbursement. The title of their study is: Are "avoidable" ED visits really avoidable? Use of the NYU ED algorithm for an ...
Main Outcomes and Measures Prolonged ED visit wait times (arrival to clinician assigned, >4 hours), prolonged LOS (arrival to departure, >12 hours), and ED revisit rate (ED discharge and return within 72 hours). Results A total of 2 761 361 ED visits across 25 EDs in 2021 and 2022 were included. From September 1 to December 31, 2022, there were ...
From 2005 to 2015, rates of prolonged LOS for pediatric mental health ED visits increased over time from 16.3% to 24.6% (LOS >6 hours) and 5.3% to 12.7% (LOS >12 hours), in contrast to non-mental health visits for which LOS remained stable.
Hmmmm, it sounds like you are talking about pts being admitted as observation, which, for billing purposes, is treated like an ED visit for the first 23 hours. There are some exceptions that go longer than that, but I couldn't begin to tell you what they are. As for what qualifies them for extended ER, or observation, I don't know that either.
This report describes rates of emergency department visits for heat-related illnesses in 2023. Skip directly to site content Skip directly to search. Español ... In the 2023 warm-season months, daily HRI ED visit rates peaked in several regions and remained elevated for a prolonged duration. More males than females sought care in EDs for HRI ...
Key Points. Question Does health care use differ after a virtual visit with a patient's own family physician compared with a visit with an outside physician?. Findings In this cohort study among 5 229 240 Ontario residents with a family physician and virtual visit, patients who had a virtual visit with an outside physician were 66% more likely to visit the emergency department within 7 days ...
Mission. The Purdue On-Campus Writing Lab and Purdue Online Writing Lab assist clients in their development as writers—no matter what their skill level—with on-campus consultations, online participation, and community engagement. The Purdue Writing Lab serves the Purdue, West Lafayette, campus and coordinates with local literacy initiatives.
Oceans cover nearly 75% of the Earth. While they seem vast and frightening, they're also enchanting and whimsical. This hour, TED speakers dive into stories of connection — and even love — in ...